Which ML model to choose? Experimental Evaluation for a beyond-5G Traffic Steering case
ICC 2023 - IEEE INTERNATIONAL CONFERENCE ON COMMUNICATIONS(2023)
摘要
Beyond 5G and future next-generation networks will have to cope with the ever-growing traffic demand for mobile traffic, as well as low-latency communications. Network densification has been long proposed as a solution for augmenting the available wireless links with more technologies, thus enhancing the available capacity for the end-users. Nevertheless, selecting the optimal split of traffic among the available links is not a trivial decision. Machine Learning (ML) approaches can assist in these decisions, by forecasting metrics collected directly from the RAN, towards predicting the near-future performance, and appropriately selecting the split of traffic. In this work, we evaluate a total of 22 different ML models in such a traffic steering use case, towards determining the solution that yields the best results in terms of accuracy of predictions, training time, and computational resources. We use a real-world testbed prototype based on OpenAirInterface to evaluate our contributions, and use realistic mobility datasets for emulating client mobility. Our results show that the different algorithms can present variations in terms of the achievable throughput, but several can substantially improve the offered wireless network capacity.
更多查看译文
AI 理解论文
溯源树
样例
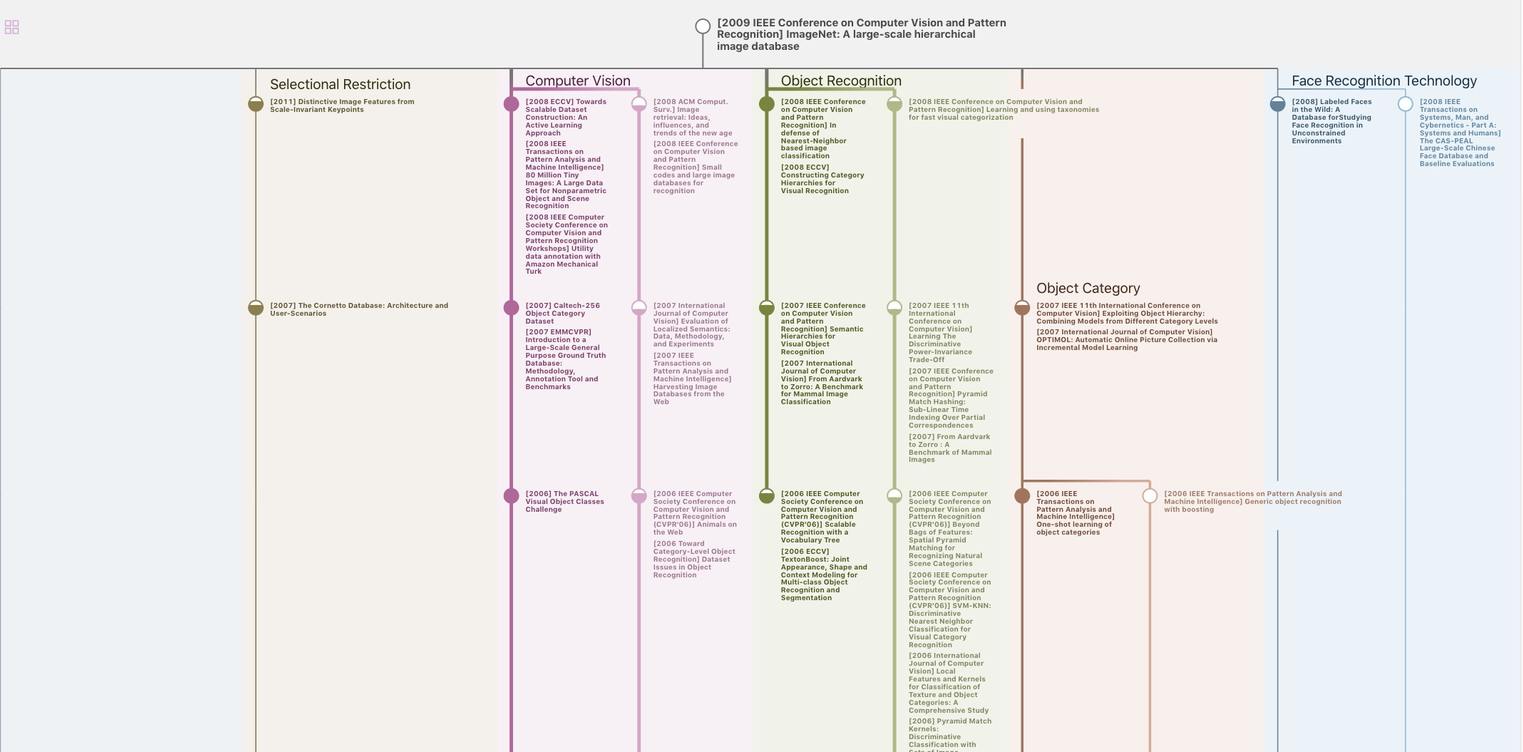
生成溯源树,研究论文发展脉络
Chat Paper
正在生成论文摘要