SynFMPL: A Federated Meta Pseudo Labeling Framework with Synergetic Strategy
ICC 2023 - IEEE INTERNATIONAL CONFERENCE ON COMMUNICATIONS(2023)
摘要
Recently, Google proposed a privacy-preserving framework, Federated Learning (FL), to realize the era of edge intelligence. However, the limited labeled data that can only be labeled by the data owner with domain know-how threatens the feasibility of FL. In fact, Semi-supervised Learning (SSL) is a key to leveraging the unlabeled data on the client side while the confirmation bias issue triggered by incorrect predictions in the old-fashion Teacher-Student structure drops the model accuracy significantly. In this paper, we introduce the state-of-the-art SSL technique called Meta Pseudo Labels (MPL), which advocates a feedback strategy in the Teacher-Student architecture to mitigate the performance degradation. Unfortunately, in FL the heterogeneous data without sharing among clients are non-Independent and Identically Distributed (non-IID). The knowledge that shuttles between the Teacher and Student model may be partial and undermine the effectiveness of MPL. To make a performance breakthrough, we proposed a novel FL framework called Federated Meta Learning with Synergetic Strategy (SynFMPL), which combines the advantages of both Teacher and Student models. To our best knowledge, we are the first work that integrates MPL technique in FL. Moreover, we specifically focus on the client side, which fully leverages the unlabeled data for model generalization. The experiment shows that SynFPML outperforms all baselines in various non-IID scenarios. The Student model in SynFMPL even exceeds the upper bound in all baselines by 4% and maintains a virtuous cycle in the learning process.
更多查看译文
关键词
Federated Learning,Semi-supervised Learning,Meta Pseudo Labels,Unlabeled Data,non-IID,Synergistic Effect
AI 理解论文
溯源树
样例
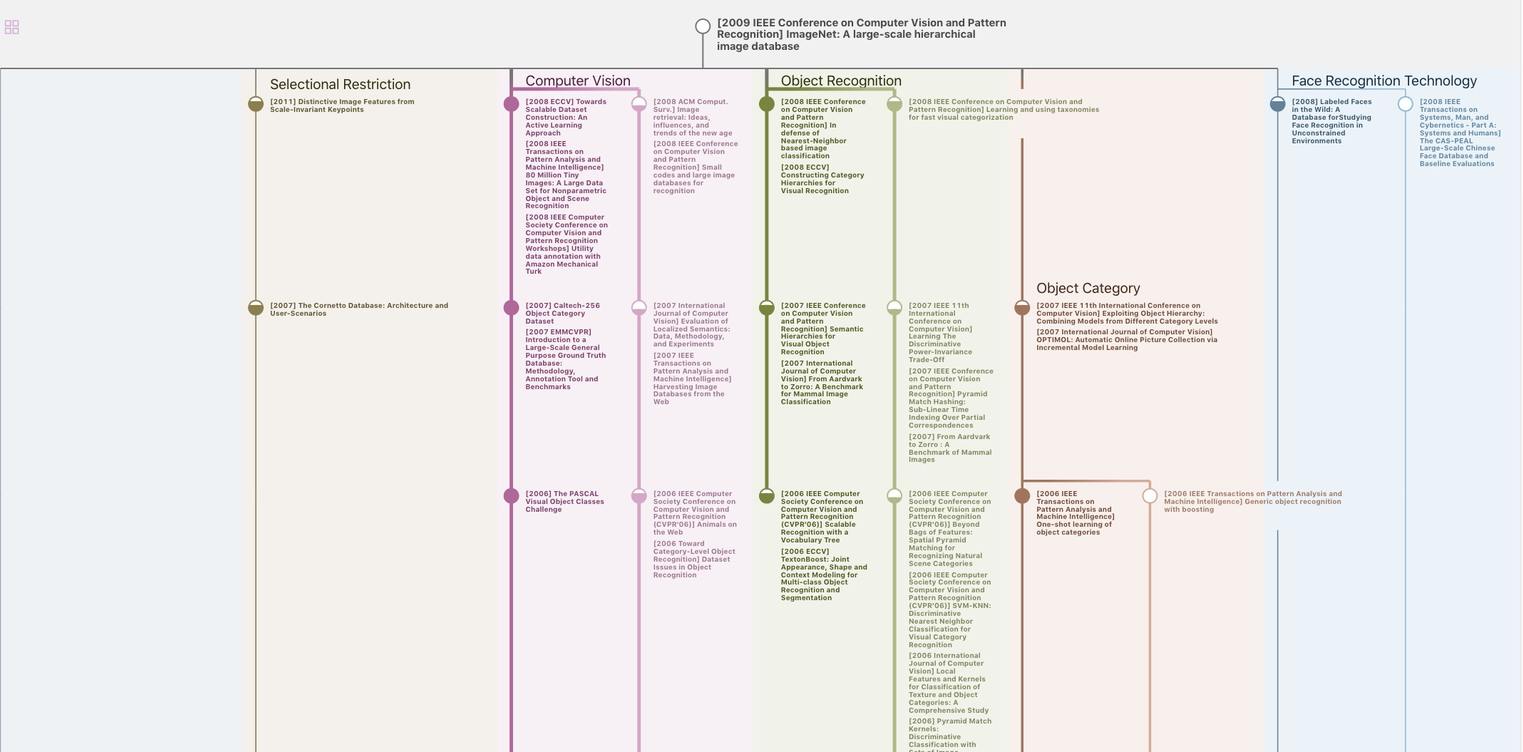
生成溯源树,研究论文发展脉络
Chat Paper
正在生成论文摘要