Multi-fine-grained DNNs Partition and Offloading over Fog Computing Networks
2023 IEEE INTERNATIONAL CONFERENCE ON COMMUNICATIONS WORKSHOPS, ICC WORKSHOPS(2023)
摘要
Deep neural networks (DNNs) have facilitated commendable performance in signal processing, thanks to their superior functions in feature extraction and abstraction representation. However, limited computing capacity of Internet of Things (IoT) devices imposes challenges to support resource-intensive DNNs inference with low latency and quality of service requirements. Benefiting from the pervasive wireless connectivity, offloading partial DNNs to fog nodes (FNs) becomes a viable solution for alleviating resource shortage and improving time and energy efficiency. This paper investigates a novel multi-fine-grained DNNs partition and offloading strategy over fog computing networks. A Multiagent Hybrid Actions Deep Deterministic Policy Gradient (MHADDPG)-based algorithm is proposed to maximize the long-term system utility, upon considering the DNNs execution delay and energy consumption of participating devices and FNs. Comprehensive simulations demonstrate that the proposed solution significantly reduces the average inference latency by 55.15%-67.08%, while saving energy by 44.39%-57.56%, for three widely adopted DNNs.
更多查看译文
关键词
DNNs inference,DNNs partition,DNNs offloading,Multiagent Hybrid Actions Deep Deterministic Policy Gradient (MHADDPG),Fog computing
AI 理解论文
溯源树
样例
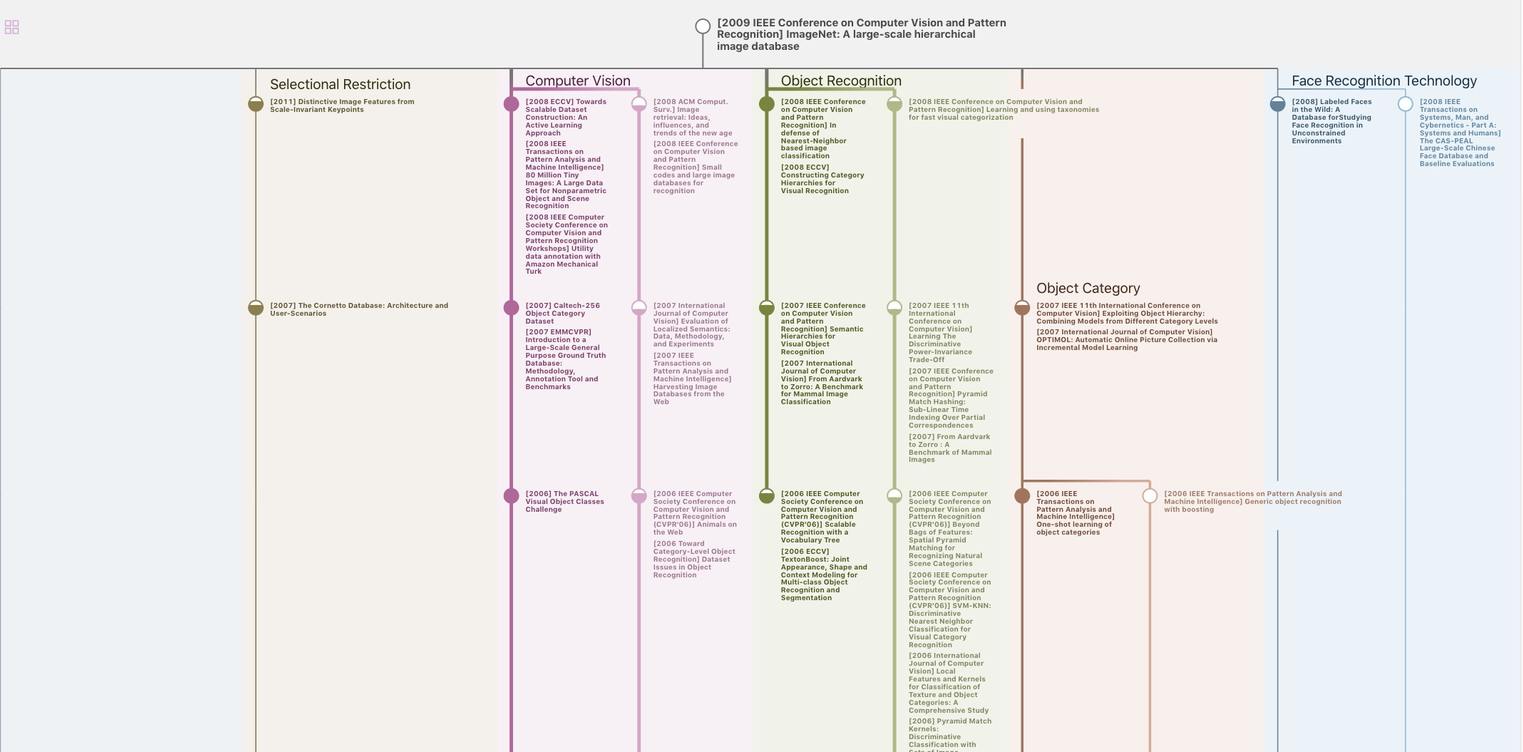
生成溯源树,研究论文发展脉络
Chat Paper
正在生成论文摘要