Robust Wireless Network Anomaly Detection with Collaborative Adversarial Autoencoders
ICC 2023 - IEEE INTERNATIONAL CONFERENCE ON COMMUNICATIONS(2023)
摘要
Anomaly detection is often deployed in centralised systems, for which critical failure points exist. However, the rising availability of low-cost, wireless-connected devices introduces opportunities for new anomaly detection techniques that leverage more robust topologies. In this paper, we propose a novel collaborative training scheme for anomaly detection models that involves sharing machine learning models amongst devices for incremental training. Using the Adversarial Autoencoder architecture, pseudo-rehearsal, and gossip-based communication, our framework provides all participating devices with a structured representation of other devices' data, so that training can continue even in the event of a device failure, with a 43% smaller performance degradation than state of the art alternatives. Under both optimal conditions and those with device failure, our model consistently exhibits better anomaly detection performance.
更多查看译文
关键词
Anomaly Detection,Wireless Networks,Cognitive Radio
AI 理解论文
溯源树
样例
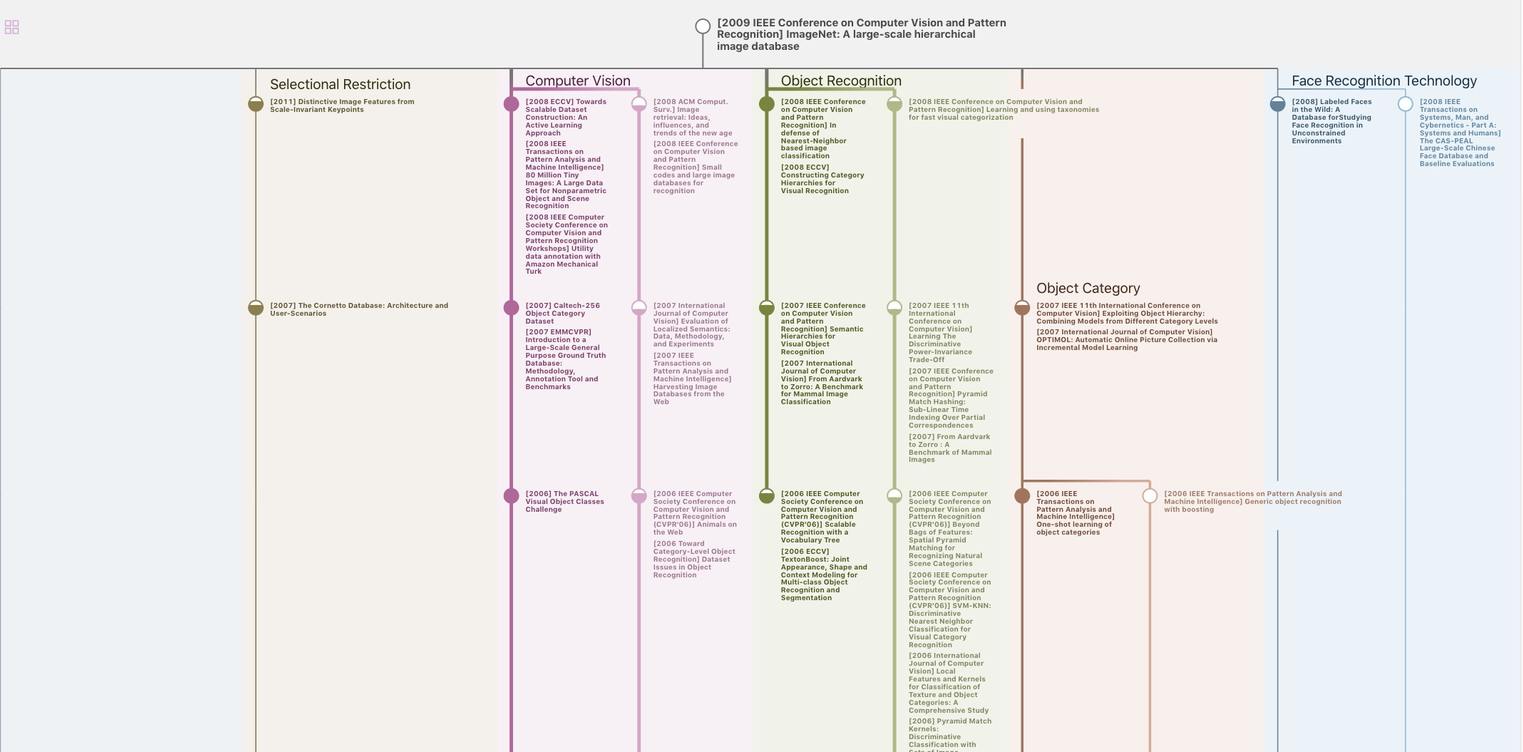
生成溯源树,研究论文发展脉络
Chat Paper
正在生成论文摘要