RNN BCJR: a fully trainable version of the additive BCJR algorithm.
ICC(2023)
摘要
We present a new version of the additive BCJR algorithm based on a recurrent neural network whose structure reflects an underlying trellis diagram. Starting from a matrix version of the equations of the additive BCJR algorithm, we derive the equivalent trainable recurrent neural network model, named Recurrent Neural Network (RNN) BCJR. The RNN BCJR consists of a linear layer to form the edge metrics from the state and input metrics, followed by a SOFTMAX/max* layer to marginalize the edge metrics back to the state and output spaces. We derive the recursions for delta propagation to train the two-layer mixing matrices from the output cost function. Unlike the previous approaches, the proposed RNN BCJR can completely replace the BCJR and is trainable from the cost functions of the outputs. The trained RNN BCJR achieves the same optimal performance as the BCJR when the model is known but at the same time can adapt itself to model mismatch, thus outperforming BCJR.
更多查看译文
关键词
Additive SISO,BCJR algorithm,model-driven recurrent neural networks
AI 理解论文
溯源树
样例
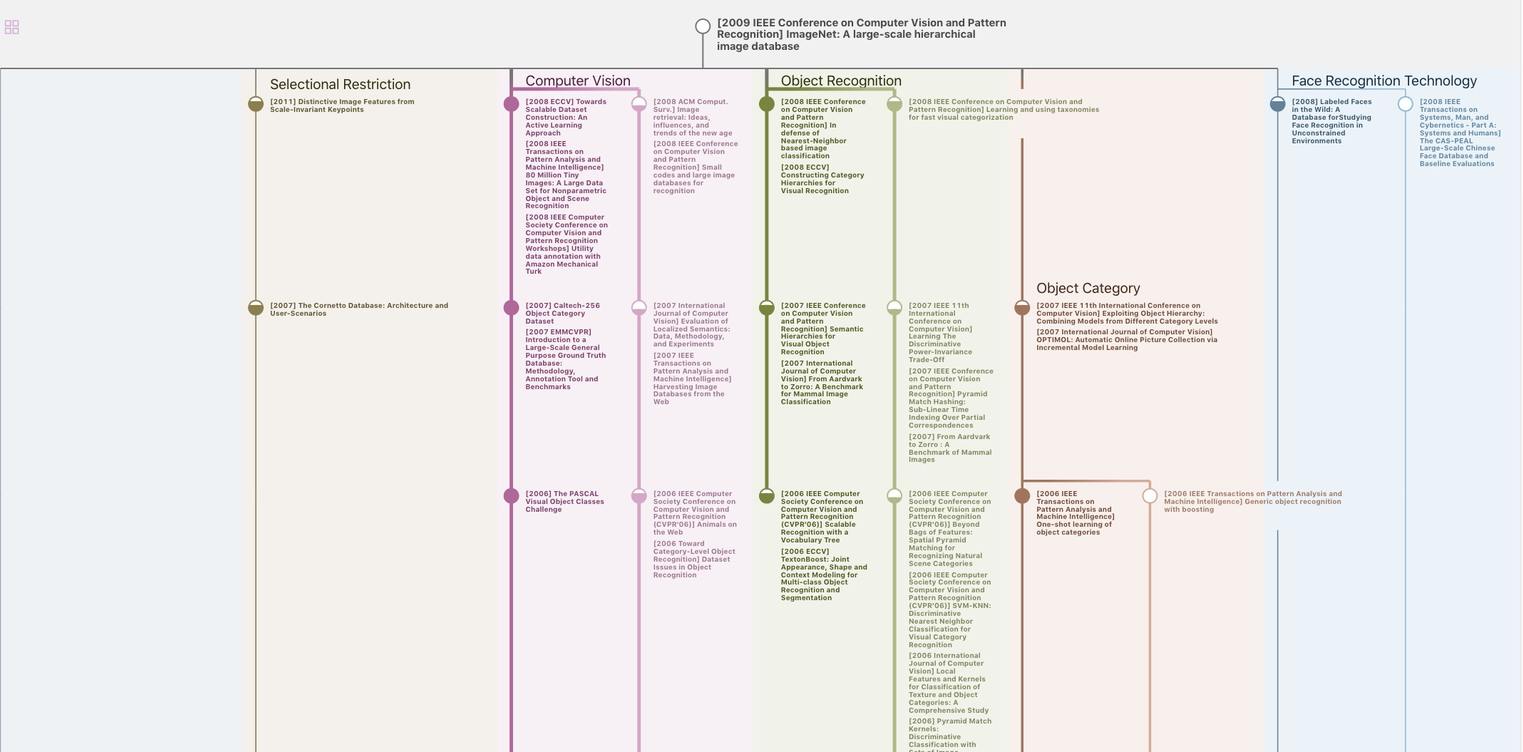
生成溯源树,研究论文发展脉络
Chat Paper
正在生成论文摘要