Mixing Temporal Graphs with MLP for Longitudinal Brain Connectome Analysis
MEDICAL IMAGE COMPUTING AND COMPUTER ASSISTED INTERVENTION, MICCAI 2023, PT II(2023)
摘要
Analyses of longitudinal brain networks, i.e., graphs, are of significant interest to understand the dynamics of brain changes with respect to aging and neurodegenerative diseases. However, each subject has a graph of heterogeneous structure and time-points as the data are obtained over several years. Moreover, most existing datasets suffer from lack of samples as the images are expensive to acquire, which leads to overfitting with complex deep neural networks. To address these issues for characterizing progressively alternations of brain connectome and region-wise measures as early as possible, we develop Spatio-Temporal Graph Multi-Layer Perceptron (STGMLP) that mixes features over both graph and time spaces to classify sets of longitudinal human brain connectomes. The proposed model is made efficient and interpretable such that it can be easily adopted to medical imaging datasets and identify personalized features responsible for a specific diagnostic label. Extensive experiments show that our method achieves successful results in both performance and computational efficiency on Alzheimer's Disease Neuroimaging Initiative (ADNI) and Adolescence Brain Cognitive Development (ABCD) datasets independently.
更多查看译文
AI 理解论文
溯源树
样例
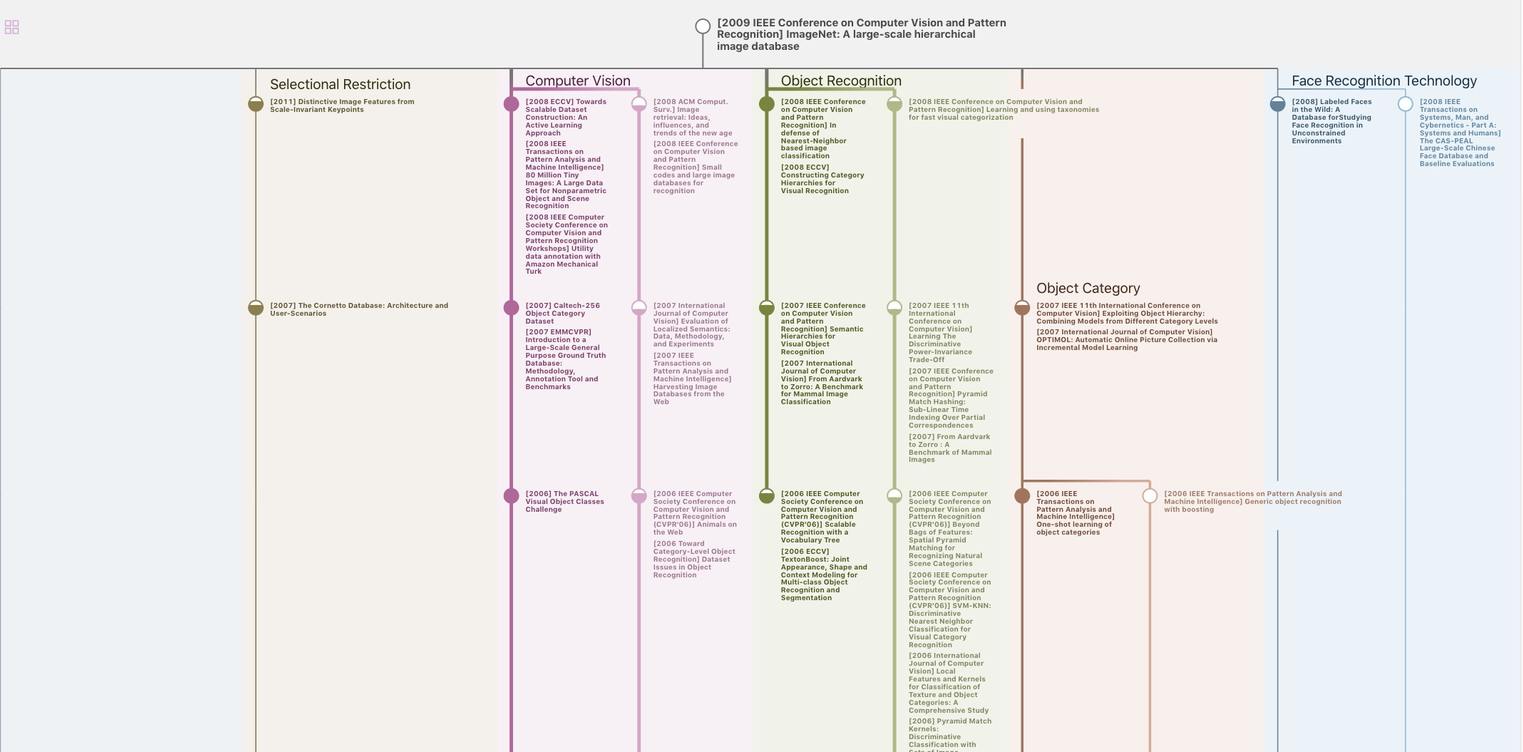
生成溯源树,研究论文发展脉络
Chat Paper
正在生成论文摘要