Explaining Massive-Training Artificial Neural Networks in Medical Image Analysis Task Through Visualizing Functions Within the Models
MEDICAL IMAGE COMPUTING AND COMPUTER ASSISTED INTERVENTION, MICCAI 2023, PT II(2023)
摘要
In this study, we proposed a novel explainable artificial intelligence (XAI) technique to explainmassive-training artificial neural networks (MTANNs). Firstly, we optimized the structure of anMTANNto find a compact model that performs equivalently well to the original one. This enables to "condense" functions in a smaller number of hidden units in the network by removing "redundant" units. Then, we applied an unsupervised hierarchical clustering algorithm to the function maps in the hidden layers with the single-linkage method. From the clustering and visualization results, we were able to group the hidden units into those with similar functions together and reveal the behaviors and functions of the trained MTANN models. We applied this XAI technique to explain the MTANN model trained to segment liver tumors in CT. The original MTANN model with 80 hidden units (F1 = 0.6894, Dice = 0.7142) was optimized to the one with nine hidden units (F1 = 0.6918, Dice = 0.7005) with almost equivalent performance. The nine hidden units were clustered into three groups, and we found the following three functions: 1) enhancing liver area, 2) suppressing non-tumor area, and 3) suppressing the liver boundary and false enhancement. The results shed light on the "black-box" problem with deep learning (DL) models; and we demonstrated that our proposed XAI technique was able to make MTANN models "transparent".
更多查看译文
关键词
Deep Learning,Explainable AI (XAI),Visualizing Functions,Liver Tumor Segmentation,Unsupervised Hierarchical Clustering
AI 理解论文
溯源树
样例
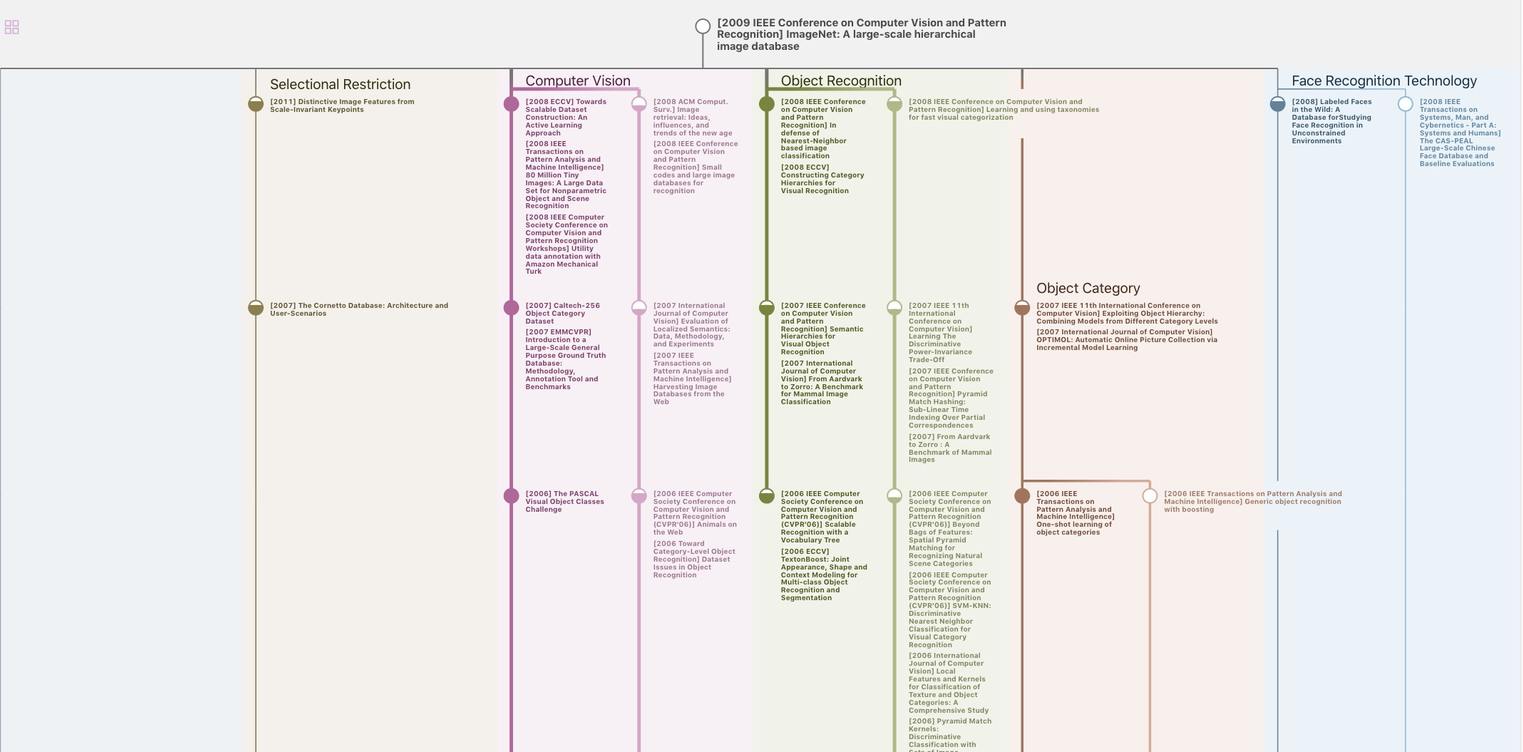
生成溯源树,研究论文发展脉络
Chat Paper
正在生成论文摘要