Stroke Outcome and Evolution Prediction from CT Brain Using a Spatiotemporal Diffusion Autoencoder
MACHINE LEARNING IN CLINICAL NEUROIMAGING, MLCN 2023(2023)
摘要
Stroke is a major cause of death and disability worldwide. Accurate outcome and evolution prediction has the potential to revolutionize stroke care by individualizing clinical decision-making leading to better outcomes. However, despite a plethora of attempts and the rich data provided by neuroimaging, modelling the ultimate fate of brain tissue remains a challenging task. In this work, we apply recent ideas in the field of diffusion probabilistic models to generate a self-supervised semantically meaningful stroke representation from Computed Tomography (CT) images. We then improve this representation by extending the method to accommodate longitudinal images and the time from stroke onset. The effectiveness of our approach is evaluated on a dataset consisting of 5,824 CT images from 3,573 patients across two medical centers with minimal labels. Comparative experiments show that our method achieves the best performance for predicting next-day severity and functional outcome at discharge.
更多查看译文
关键词
Stroke,Computed Tomography,Diffusion model,Outcome prediction
AI 理解论文
溯源树
样例
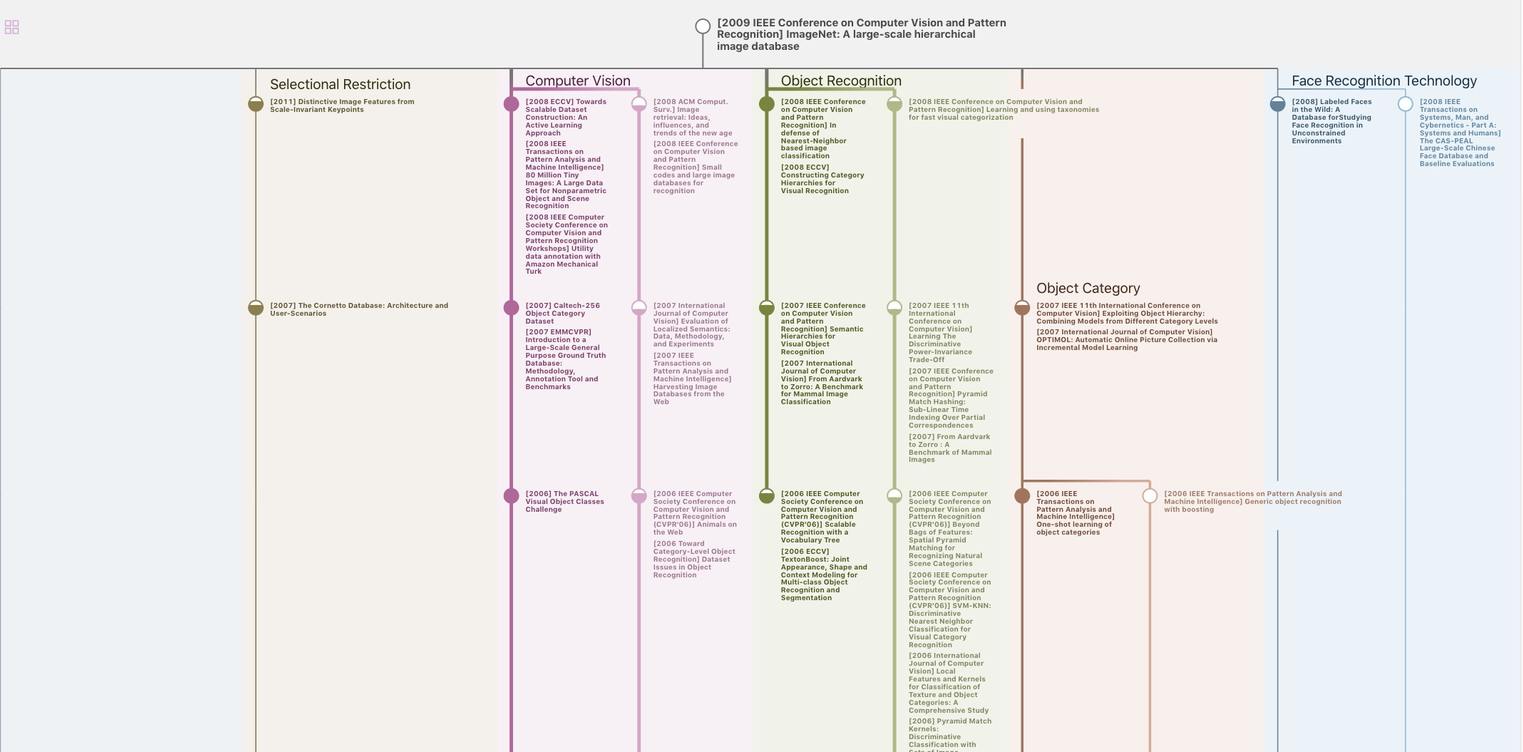
生成溯源树,研究论文发展脉络
Chat Paper
正在生成论文摘要