Weakly Supervised Medical Image Segmentation Through Dense Combinations of Dense Pseudo-Labels
DATA ENGINEERING IN MEDICAL IMAGING, DEMI 2023(2023)
摘要
Annotating a large amount of medical imaging data thoroughly for training purposes can be expensive, particularly for medical image segmentation tasks. Instead, obtaining less precise scribblelike annotations is more feasible for clinicians. In this context, training semantic segmentation networks with limited-signal supervision remains a technical challenge. We present an innovative scribble-supervised approach to image segmentation via densely combining dense pseudo-labels which consists of groups of CNN- and ViT-based segmentation networks. A simple yet efficient dense collaboration scheme called Collaborative Hybrid Networks (CHNets) ensembles dense pseudo-labels to expand the dataset such that it mimics full-signal supervision. Additionally, internal consistency and external consistency training of the collaborating networks are proposed, so as to ensure that each network is beneficial to the others. This results in a significant overall improvement. Our experiments on a public MRI benchmark dataset demonstrate that our proposed approach outperforms other weakly-supervised methods on various metrics. The source code of CHNets, ten baseline methods, and dataset are available at https://github.com/ziyangwang007/CV-WSL-MIS.
更多查看译文
关键词
Weakly-Supervised Learning,Convolution,Vision Transformer,Image Segmentation,Pseudo-Labels
AI 理解论文
溯源树
样例
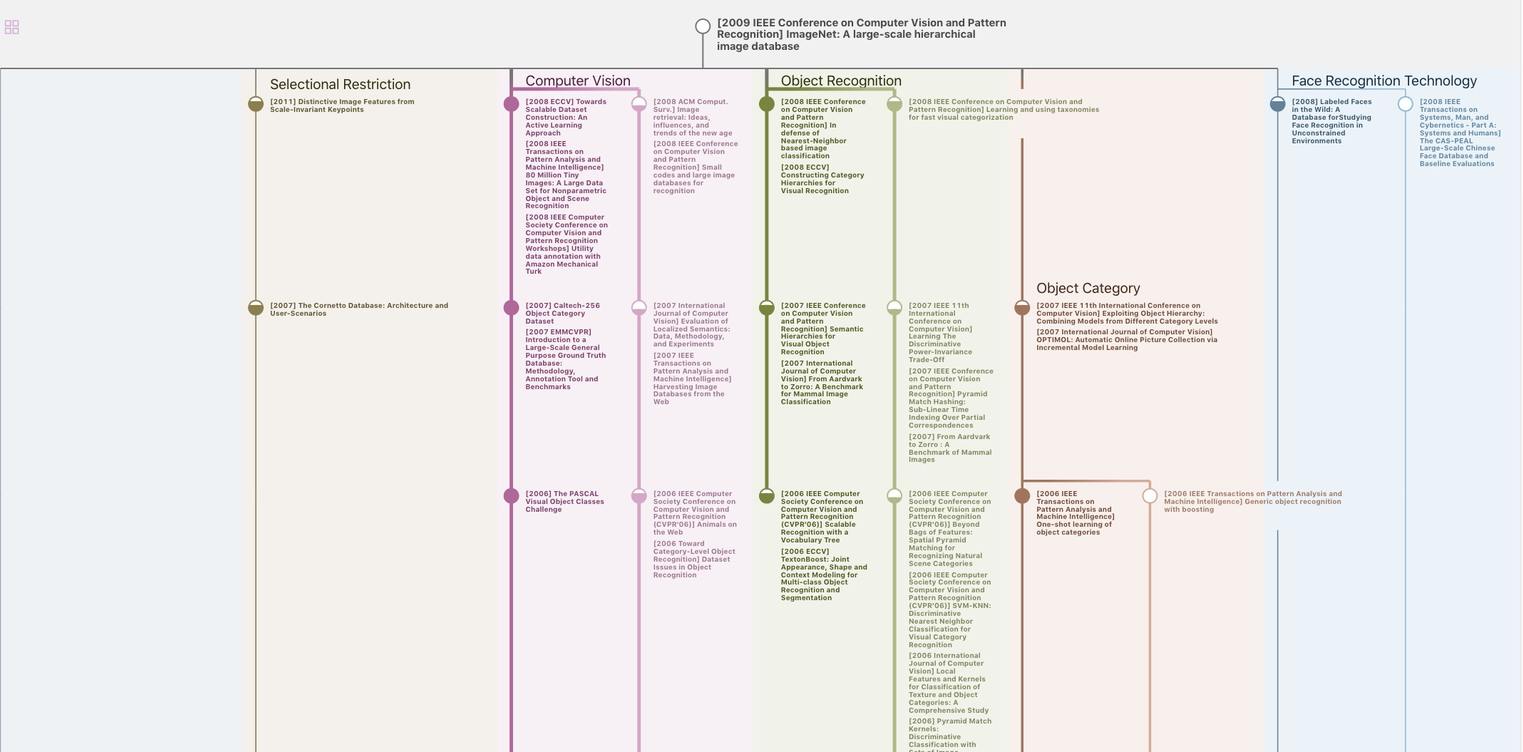
生成溯源树,研究论文发展脉络
Chat Paper
正在生成论文摘要