Diversity-Preserving Chest Radiographs Generation from Reports in One Stage
MEDICAL IMAGE COMPUTING AND COMPUTER ASSISTED INTERVENTION, MICCAI 2023, PT V(2023)
摘要
Automating the analysis of chest radiographs based on deep learning algorithms has the potential to improve various steps of the radiology workflow. Such algorithms require large, labeled and domain-specific datasets, which are difficult to obtain due to privacy concerns and laborious annotations. Recent advances in generating X-rays from radiology reports provide a possible remedy for this problem. However, due to the complexity of medical images, existing methods synthesize low-fidelity X-rays and cannot guarantee image diversity. In this paper, we propose a diversity-preserving report-to-X-ray generation method with one-stage architecture, named DivXGAN. Specifically, we design a domain-specific hierarchical text encoder to extract medical concepts inherent in reports. This information is incorporated into a one-stage generator, along with the latent vectors, to generate diverse yet relevant Xray images. Extensive experiments on two widely used datasets, namely Open-i and MIMIC-CXR, demonstrate the high fidelity and diversity of our synthesized chest radiographs. Furthermore, we demonstrate the efficacy of the generated X-rays in facilitating supervised downstream applications via a multi-label classification task.
更多查看译文
关键词
Chest X-ray generation,Radiology report,Generative adversarial networks,One-stage architecture
AI 理解论文
溯源树
样例
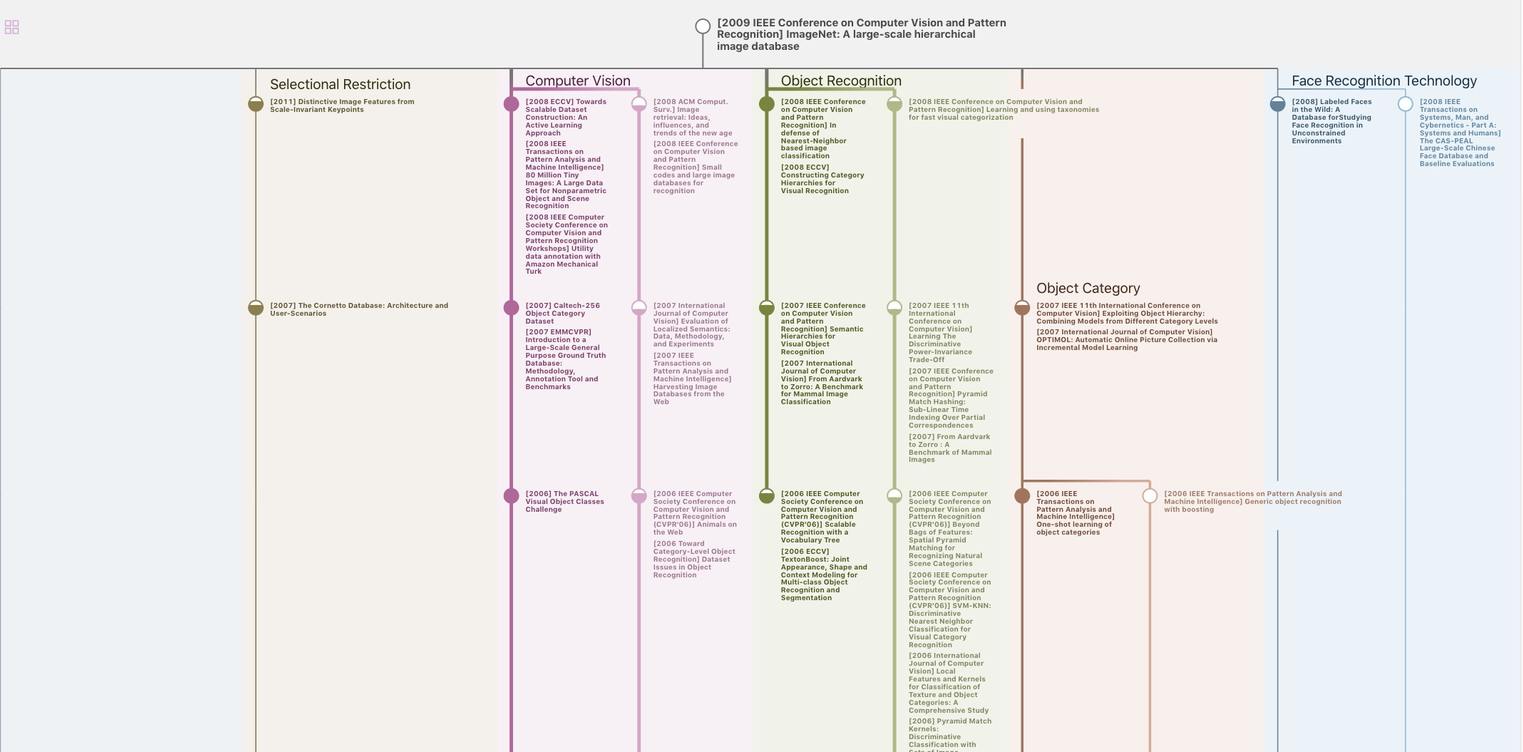
生成溯源树,研究论文发展脉络
Chat Paper
正在生成论文摘要