Fast Non-Markovian Diffusion Model for Weakly Supervised Anomaly Detection in Brain MR Images
MEDICAL IMAGE COMPUTING AND COMPUTER ASSISTED INTERVENTION, MICCAI 2023, PT V(2023)
摘要
In medical image analysis, anomaly detection in weakly supervised settings has gained significant interest due to the high cost associated with expert-annotated pixel-wise labeling. Current methods primarily rely on auto-encoders and flow-based healthy image reconstruction to detect anomalies. However, these methods have limitations in terms of high-fidelity generation and suffer from complicated training processes and low-quality reconstructions. Recent studies have shown promising results with diffusion models in image generation. However, their practical value in medical scenarios is restricted due to their weak detail-retaining ability and low inference speed. To address these limitations, we propose a fast non-Markovian diffusion model (FNDM) with hybrid-condition guidance to detect high-precision anomalies in the brain MR images. A non-Markovian diffusion process is designed to enable the efficient transfer of anatomical information across diffusion steps. Additionally, we introduce new hybrid pixel-wise conditions as more substantial guidance on hidden states, which enables the model to concentrate more efficiently on the anomaly regions. Furthermore, to reduce computational burden during clinical applications, we have accelerated the encoding and sampling procedures in our FNDM using multi-step ODE solvers. As a result, our proposed FNDM method outperforms the previous state-of-the-art diffusion model, achieving a 9.56% and 19.98% improvement in Dice scores on the BRATS 2020 and ISLES datasets, respectively, while requiring only six times less computational cost.
更多查看译文
关键词
Anomaly Detection,Diffusion Probabilistic Model,Medical Image Analysis
AI 理解论文
溯源树
样例
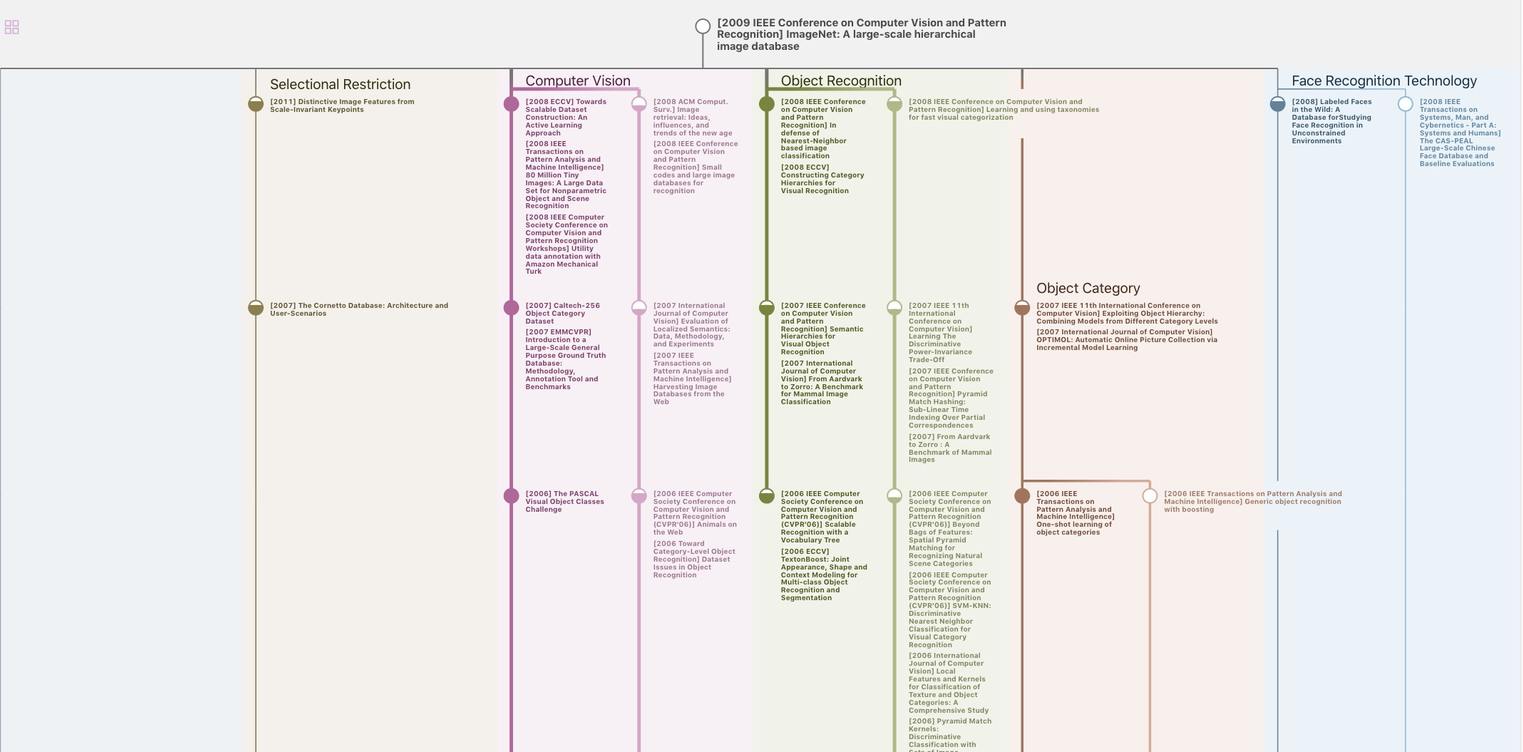
生成溯源树,研究论文发展脉络
Chat Paper
正在生成论文摘要