Multi-scale Self-Supervised Learning for Longitudinal Lesion Tracking with Optional Supervision
MEDICAL IMAGE COMPUTING AND COMPUTER ASSISTED INTERVENTION, MICCAI 2023, PT I(2023)
摘要
Longitudinal lesion or tumor tracking is an essential task in different clinical workflows, including treatment monitoring with followup imaging or planning of re-treatments for radiation therapy. Accurately establishing correspondence between lesions at different timepoints, recognizing new lesions or lesions that have disappeared is a tedious task that only grows in complexity as the number of lesions or timepoints increase. To address this task, we propose a generic approach based on multi-scale self-supervised learning. The multi-scale approach allows the efficient and robust learning of a similarity map between multi-timepoint image acquisitions to derive correspondence, while the self-supervised learning formulation enables the generic application to different types of lesions and image modalities. In addition, we impose optional supervision during training by leveraging tens of anatomical landmarks that can be extracted automatically. We train our approach at large scale with more than 50,000 computed tomography (CT) scans and validate it on two different applications: 1) Tracking of generic lesions based on the DeepLesion dataset, including liver tumors, lung nodules, enlarged lymph-nodes, for which we report highest matching accuracy of 92%, with localization accuracy that is nearly 10% higher than the state-of-the-art; and 2) Tracking of lung nodules based on the NLST dataset for which we achieve similarly high performance. In addition, we include an error analysis based on expert radiologist feedback, and discuss next steps as we plan to scale our system across more applications.
更多查看译文
关键词
Self-supervised learning,Multi-scale,Longitudinal lesion tracking
AI 理解论文
溯源树
样例
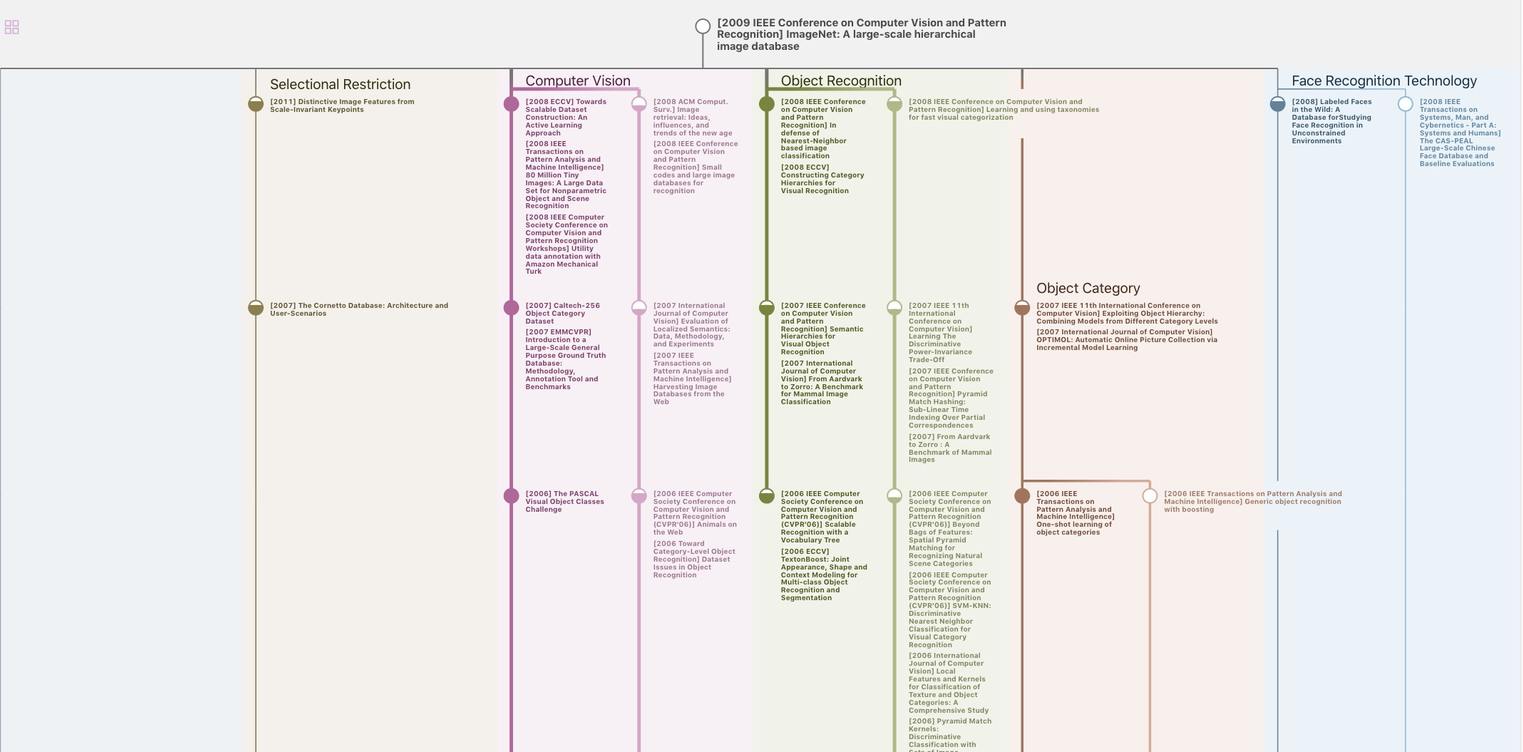
生成溯源树,研究论文发展脉络
Chat Paper
正在生成论文摘要