Distributionally Robust Image Classifiers for Stroke Diagnosis in Accelerated MRI
MEDICAL IMAGE COMPUTING AND COMPUTER ASSISTED INTERVENTION, MICCAI 2023, PT V(2023)
摘要
Magnetic Resonance Imaging (MRI) acceleration techniques using k-space sub-sampling (KS) can greatly improve the efficiency of MRI-based stroke diagnosis. Although Deep Neural Networks (DNN) have shown great potential on stroke lesion recognition tasks when the MR images are reconstructed from the full k-space, they are vulnerable to the lower quality MR images generated by KS. In this paper, we propose a Distributionally Robust Learning (DRL) approach to improve the performance of stroke recognition DNN models when the MR images are reconstructed from the sub-sampled k-space. For Convolutional Neural Network (CNN) and Vision Transformer (ViT)-based models, our methods improve the stroke classification AUROC and AUPRC by up to 11.91% and 9.32% on the KS-perturbed brain MR images, respectively, compared against Empirical Risk Minimization (ERM) and other baseline defensive methods. We further show that DRL models can successfully recognize the stroke cases from highly perturbed MR images where clinicians may fail, which provides a solution for improved diagnosis in an accelerated MRI setting.
更多查看译文
关键词
Stroke diagnosis,MRI acceleration,Distributionally robust optimization,Deep learning
AI 理解论文
溯源树
样例
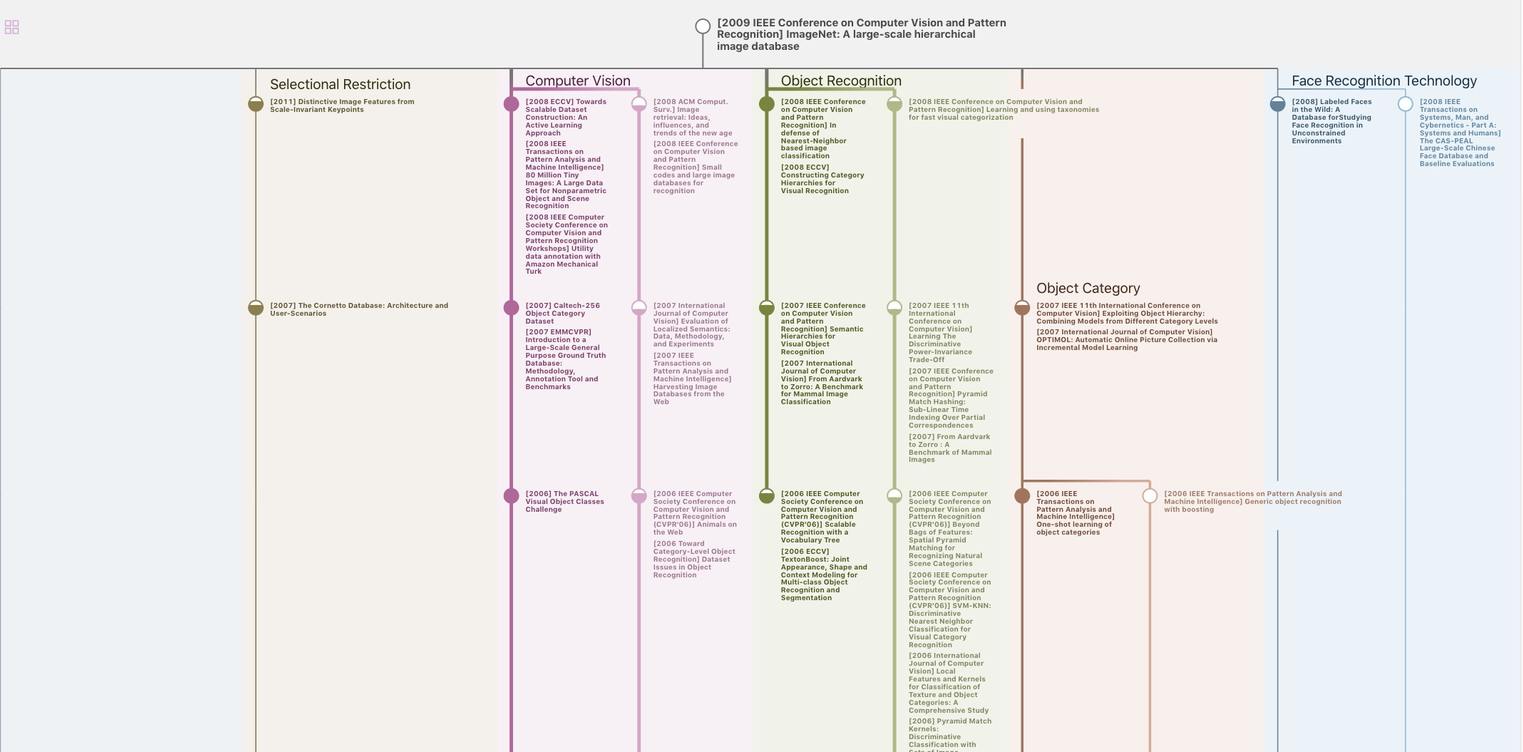
生成溯源树,研究论文发展脉络
Chat Paper
正在生成论文摘要