Physics-based Data-Augmented Deep Learning for Enhanced Autogenous Shrinkage Prediction on Experimental Dataset.
IC3(2023)
摘要
Prediction of the autogenous shrinkage referred to as the reduction of apparent volume of concrete under seal and isothermal conditions is of great significance in the service life analysis and design of durable concrete structures, especially with the increasing use of concrete with low water-to-cement ratios. However, due to the highly complex mechanism of autogenous shrinkage, it is hard to design accurate mechanistic models for it. Existing state-of-the-art models for autogenous shrinkage do not perform well for several reasons such as not being able to capture faster shrinkage change at early ages (swelling), coefficients used are derived using statistical optimization methods to fit certain databases only, and mechanism to identify the most influencing factors on autogenous shrinkage is not present. Moreover, it is also challenging to deploy a machine learning framework directly to perform predictive analysis due to the sparse and noisy nature of the available experimental dataset. In this paper, we study and propose a method to combine the physics-based knowledge and the predictive ability of deep regression neural networks to mitigate the shortcomings of the existing models. We introduce a novel data augmentation technique that utilizes physics based knowledge to improve the accuracy while maintaining the characteristics of autogenous shrinkage in its predictions simultaneously. Using state-of-the-art B4 model, a genetic algorithm, and a deep neural network trained using raw data for comparison, we show that the proposed methods help improve the accuracy of the model as compared to other methods. We also observe that the proposed method is able to successfully learn and predict the swelling component of the shrinkage strain curve as well, which cannot be predicted using the existing state-of-the-art models.
更多查看译文
AI 理解论文
溯源树
样例
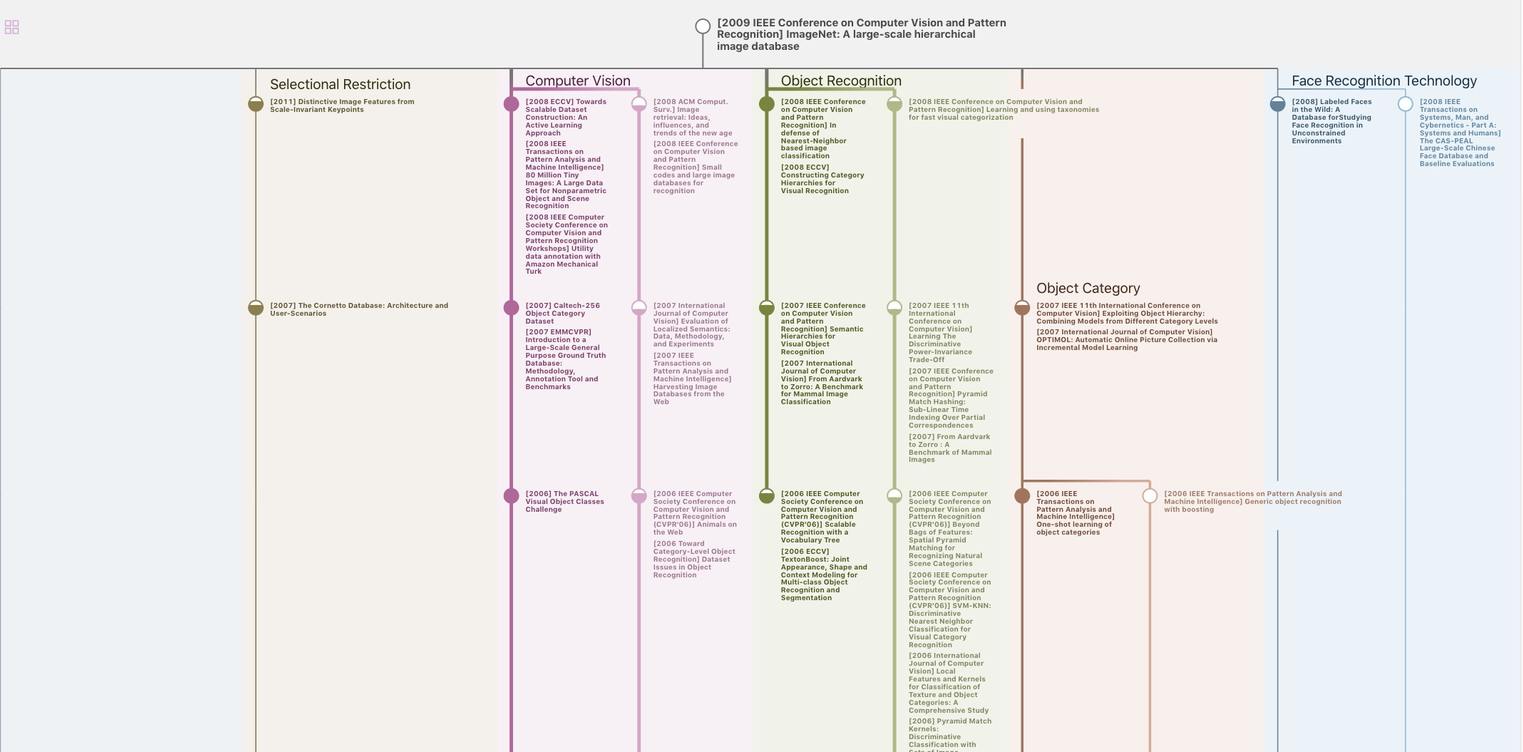
生成溯源树,研究论文发展脉络
Chat Paper
正在生成论文摘要