A Multi-task Method for Immunofixation Electrophoresis Image Classification
MEDICAL IMAGE COMPUTING AND COMPUTER ASSISTED INTERVENTION, MICCAI 2023, PT VI(2023)
摘要
In the field of plasma cell disorders diagnosis, the detection of abnormal monoclonal (M) proteins through Immunofixation Electrophoresis (IFE) is a widely accepted practice. However, the classification of IFE images into nine distinct categories is a complex task due to the significant class imbalance problem. To address this challenge, a two-sub-task classification approach is proposed, which divides the classification task into the determination of severe and mild cases, followed by their combination to produce the final result. This strategy is based on the expert understanding that the nine classes are different combinations of severe and mild cases. Additionally, the examination of the dense band co-location on the electrophoresis lane and other lanes is crucial in the expert evaluation of the image class. To incorporate this expert knowledge into the model training, inner-task and inter-task regularization is introduced. The effectiveness of the proposed method is demonstrated through experiments conducted on approximately 15,000 IFE images, resulting in interpretable visualization outcomes that are in alignment with expert expectations. Codes are available at https://github.com/shiy19/IFE-classification.
更多查看译文
关键词
IFE image classification,Multi-task learning,Task-related regularization,Class imbalance,Expert knowledge
AI 理解论文
溯源树
样例
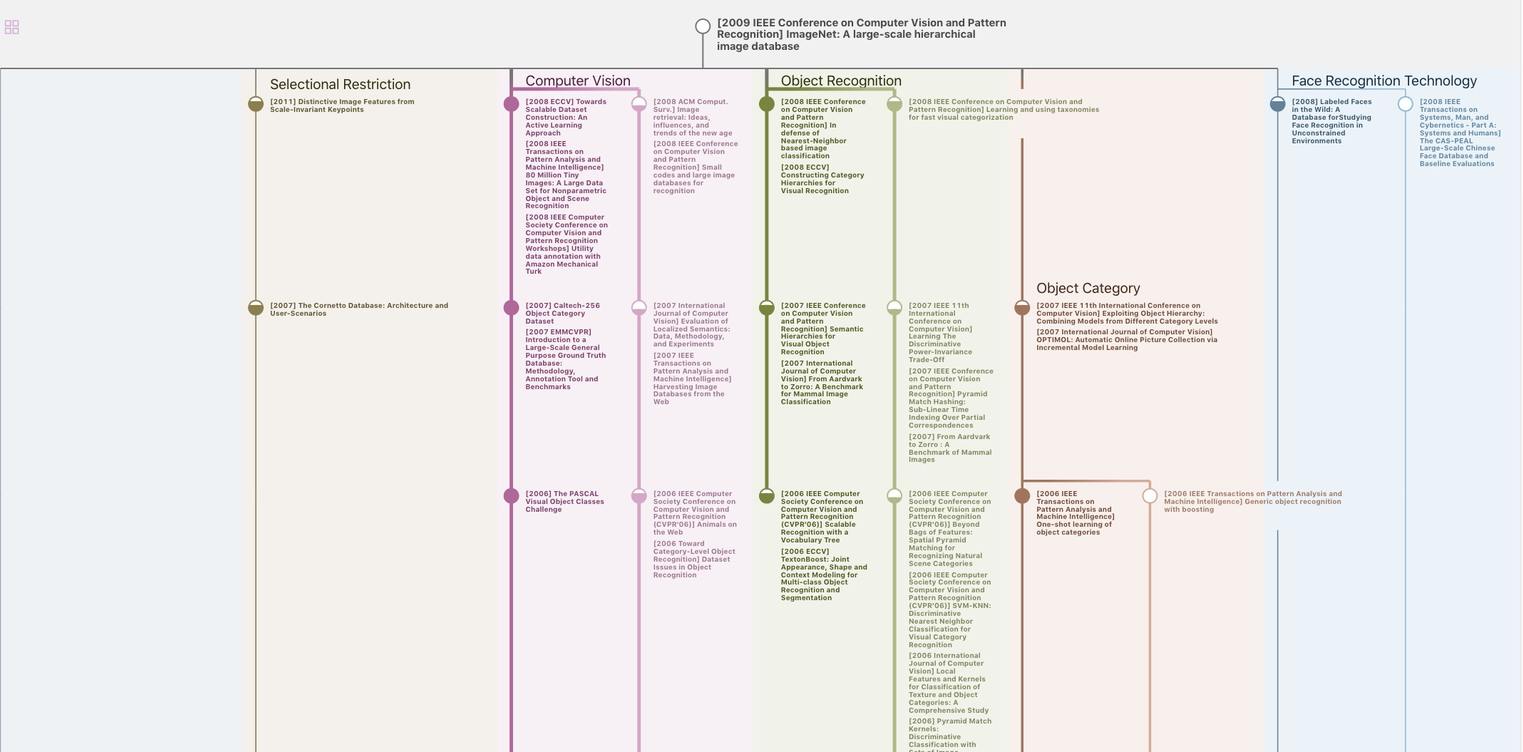
生成溯源树,研究论文发展脉络
Chat Paper
正在生成论文摘要