Style Enhanced Domain Adaptation Neural Network for Cross-Modality Cervical Tumor Segmentation
COMPUTATIONAL MATHEMATICS MODELING IN CANCER ANALYSIS, CMMCA 2023(2023)
摘要
Cervical tumor segmentation is an essential step of cervical cancer diagnosis and treatment. Considering that multi-modality data contain more information and are widely available in clinical routine, multi-modality medical image analysis has emerged as a significant field of study. However, annotating tumors for each modality is expensive and time-consuming. Consequently, unsupervised domain adaptation (UDA) has attracted a lot of attention for its ability to achieve excellent performance on unlabeled cross-domain data. Most current UDA methods adapt image translation networks to achieve domain adaptation, however, the generation process may create visual inconsistency and incorrect generation styles due to the instability of generative adversarial networks. Therefore, we propose a novel and efficient method without image translation networks by introducing a style enhancement method into Domain Adversarial Neural Network (DANN)-based model to improve the generalization performance of the shared segmentation network. Experimental results show that our method achieves the best performance on the cross-modality cervical tumor segmentation task compared to current state-of-the-art UDA methods.
更多查看译文
关键词
Unsupervised domain adaptation,Cervical tumor segmentation,Shuffle Remap
AI 理解论文
溯源树
样例
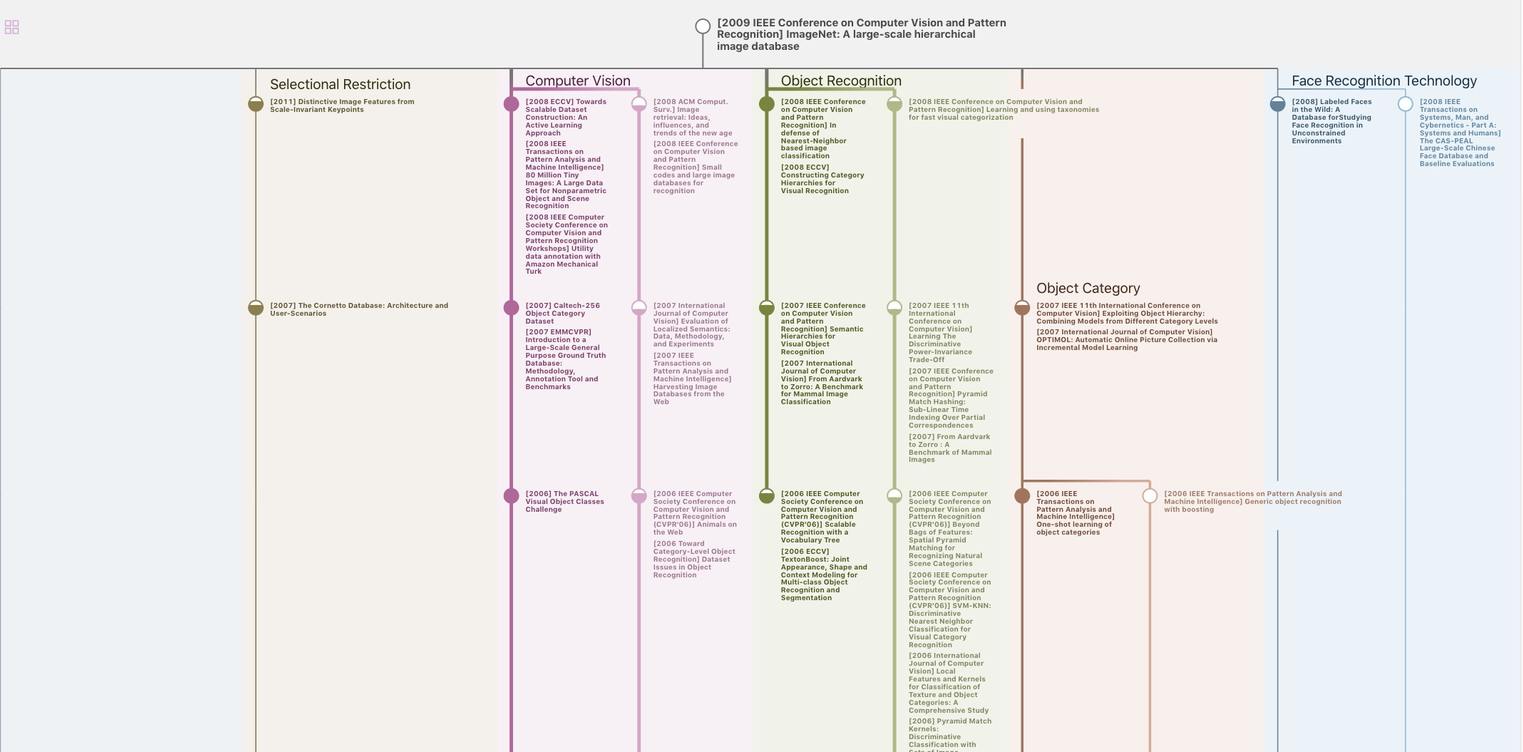
生成溯源树,研究论文发展脉络
Chat Paper
正在生成论文摘要