Adjustable Robust Transformer for High Myopia Screening in Optical Coherence Tomography
MEDICAL IMAGE COMPUTING AND COMPUTER ASSISTED INTERVENTION, MICCAI 2023, PT V(2023)
摘要
Myopia is a manifestation of visual impairment caused by an excessively elongated eyeball. Image data is critical material for studying high myopia and pathological myopia. Measurements of spherical equivalent and axial length are the gold standards for identifying high myopia, but the available image data for matching them is scarce. In addition, the criteria for defining high myopia vary from study to study, and therefore the inclusion of samples in automated screening efforts requires an appropriate assessment of interpretability. In this work, we propose a model called adjustable robust transformer (ARTran) for high myopia screening of optical coherence tomography (OCT) data. Based on vision transformer, we propose anisotropic patch embedding (APE) to capture more discriminative features of high myopia. To make the model effective under variable screening conditions, we propose an adjustable class embedding (ACE) to replace the fixed class token, which changes the output to adapt to different conditions. Considering the confusion of the data at high myopia and low myopia threshold, we introduce the label noise learning strategy and propose a shifted subspace transition matrix (SST) to enhance the robustness of the model. Besides, combining the two structures proposed above, the model can provide evidence for uncertainty evaluation. The experimental results demonstrate the effectiveness and reliability of the proposed method. Code is available at: https://github.com/maxiao0234/ARTran.
更多查看译文
关键词
High myopia screening,Optical coherence tomography,Adjustable model,Label noise learning
AI 理解论文
溯源树
样例
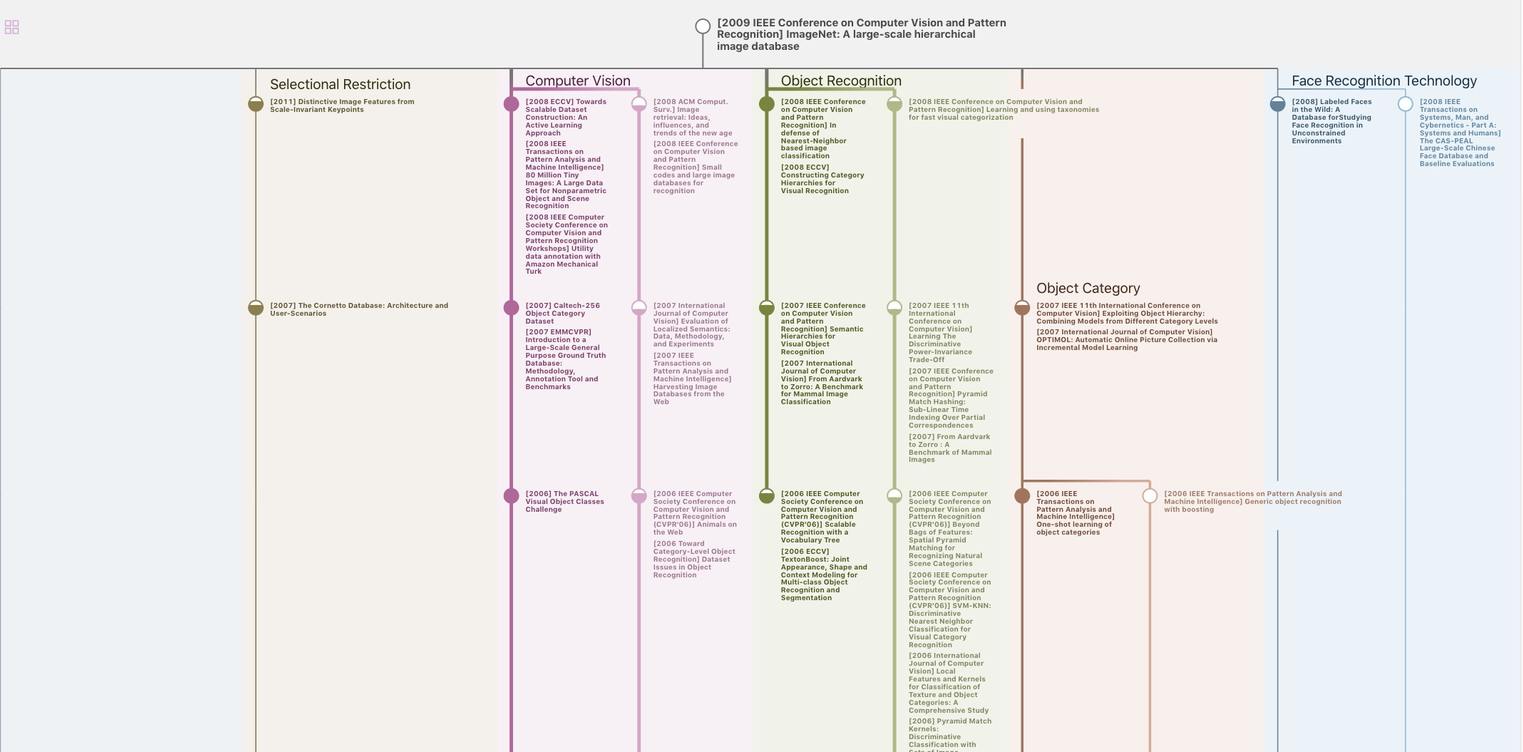
生成溯源树,研究论文发展脉络
Chat Paper
正在生成论文摘要