Learning with Synthesized Data for Generalizable Lesion Detection in Real PET Images
MEDICAL IMAGE COMPUTING AND COMPUTER ASSISTED INTERVENTION, MICCAI 2023, PT V(2023)
摘要
Deep neural networks have recently achieved impressive performance of automated tumor/lesion quantification with positron emission tomography (PET) imaging. However, deep learning usually requires a large amount of diverse training data, which is difficult for some applications such as neuroendocrine tumor (NET) image quantification, because of low incidence of the disease and expensive annotation of PET data. In addition, current deep lesion detection models often suffer from performance degradation when applied to PET images acquired with different scanners or protocols. In this paper, we propose a novel single-source domain generalization method, which learns with human annotation-free, list mode-synthesized PET images, for hepatic lesion identification in real-world clinical PET data. We first design a specific data augmentation module to generate out-of-domain images from the synthesized data, and incorporate it into a deep neural network for cross domain-consistent feature encoding. Then, we introduce a novel patch-based gradient reversal mechanism and explicitly encourage the network to learn domain-invariant features. We evaluate the proposed method on multiple cross-scanner Ga-68-DOTATATE PET liver NET image datasets. The experiments show that our method significantly improves lesion detection performance compared with the baseline and outperforms recent state-of-the-art domain generalization approaches.
更多查看译文
关键词
Lesion detection,PET images,domain generalization
AI 理解论文
溯源树
样例
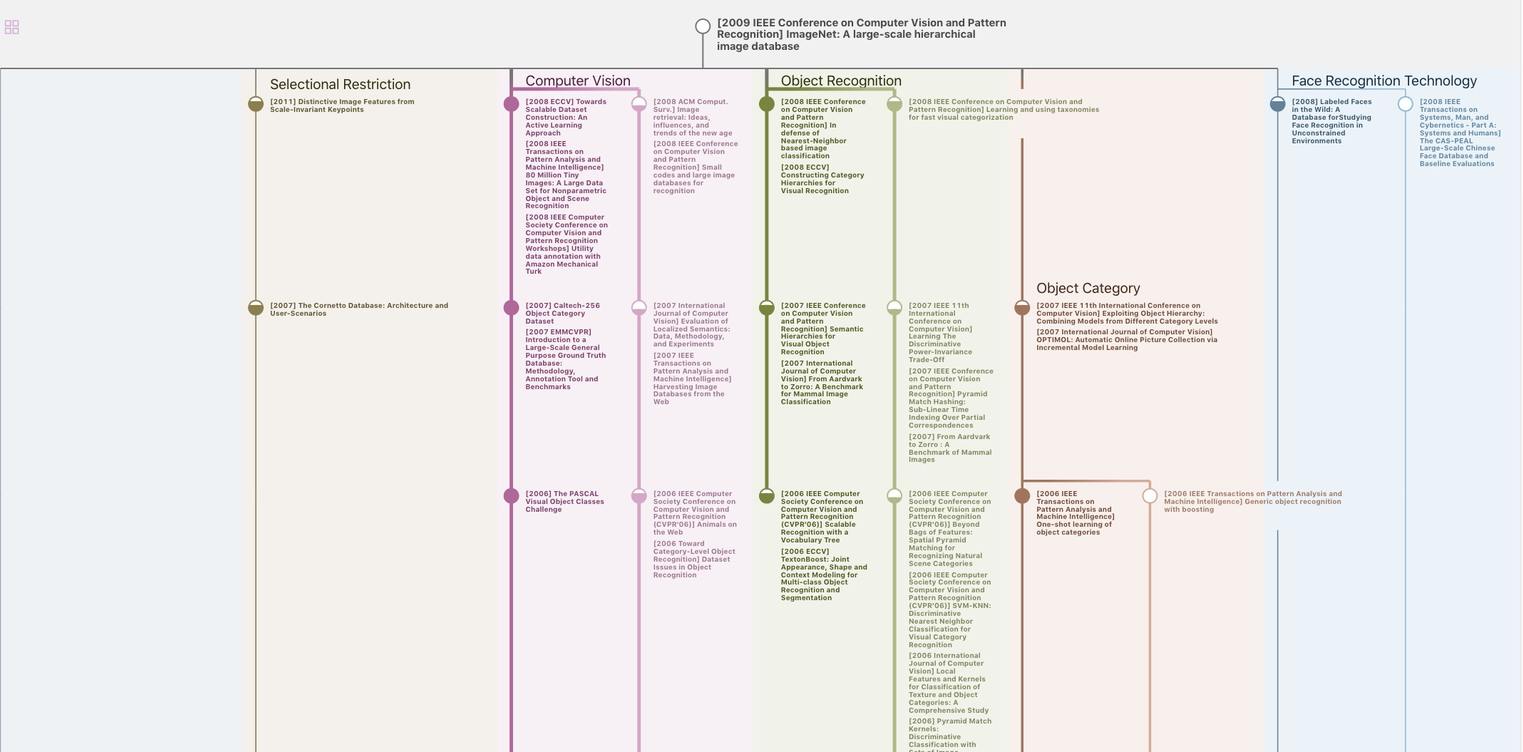
生成溯源树,研究论文发展脉络
Chat Paper
正在生成论文摘要