AirwayFormer: Structure-Aware Boundary-Adaptive Transformers for Airway Anatomical Labeling
MEDICAL IMAGE COMPUTING AND COMPUTER ASSISTED INTERVENTION, MICCAI 2023, PT VII(2023)
摘要
Pulmonary airway labeling identifies anatomical names for branches in bronchial trees. These fine-grained labels are critical for disease diagnosis and intra-operative navigation. Recently, various methods have been proposed for this task. However, accurate labeling of each bronchus is challenging due to the fine-grained categories and interindividual variations. On the one hand, training a network with limited data to recognize multitudinous classes sets an obstacle to the design of algorithms. We propose to maximize the use of latent relationships by a transformer-based network. Neighborhood information is properly integrated to capture the priors in the tree structure, while a U-shape layout is introduced to exploit the correspondence between different nomenclature levels. On the other hand, individual variations cause the distribution overlapping of adjacent classes in feature space. To resolve the confusion between sibling categories, we present a novel generator that predicts the weight matrix of the classifier to produce dynamic decision boundaries between subsegmental classes. Extensive experiments performed on publicly available datasets demonstrate that our method can perform better than state-of-the-art methods. The code is publicly available at https://github.com/EndoluminalSurgicalVision-IMR/AirwayFormer.
更多查看译文
关键词
Airway anatomical labeling,Structural prior,Dynamic decision boundary
AI 理解论文
溯源树
样例
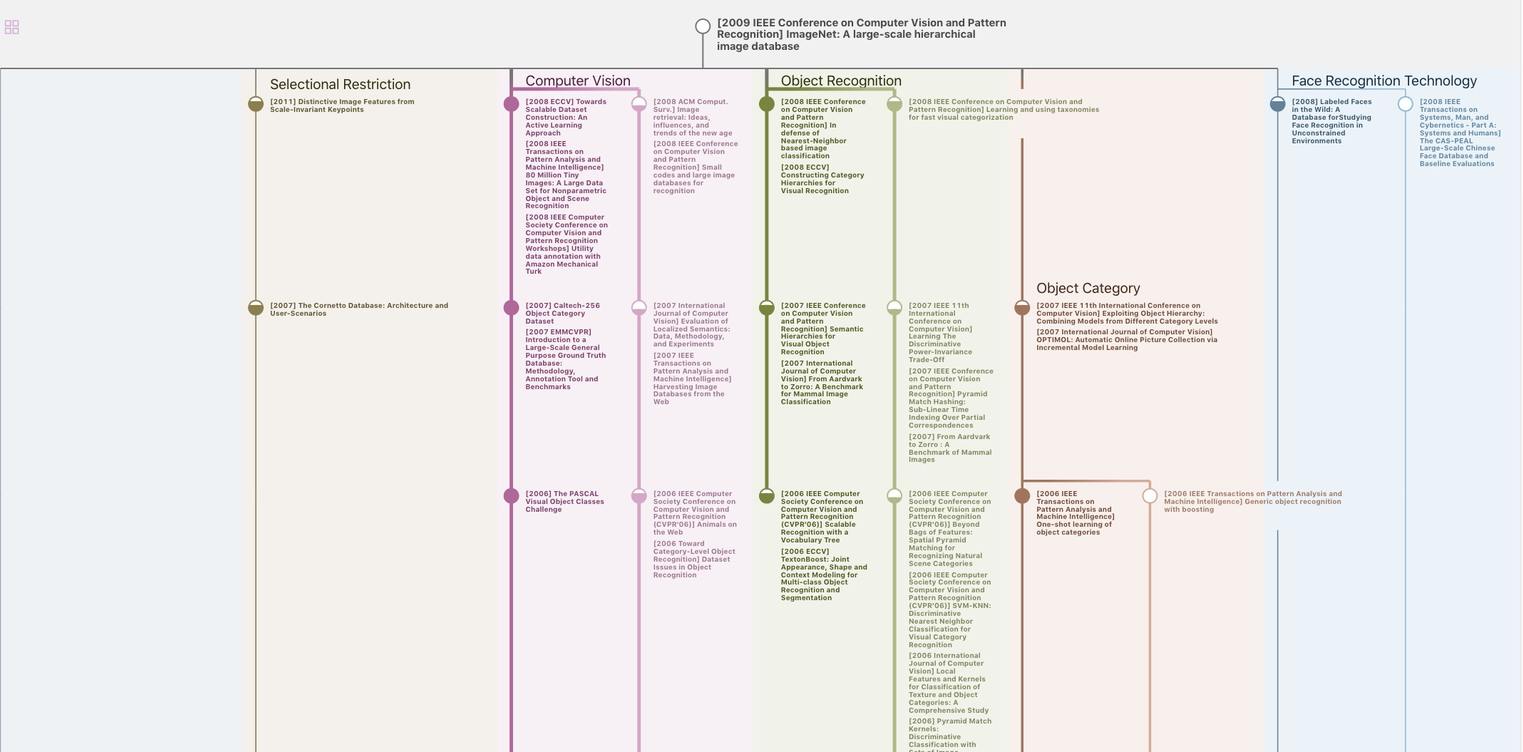
生成溯源树,研究论文发展脉络
Chat Paper
正在生成论文摘要