A Spatial-Temporally Adaptive PINN Framework for 3D Bi-Ventricular Electrophysiological Simulations and Parameter Inference
MEDICAL IMAGE COMPUTING AND COMPUTER ASSISTED INTERVENTION, MICCAI 2023, PT VII(2023)
摘要
Physics-informed neural networks (PINNs) is a new paradigm for solving the forward and inverse problems of partial differential equations (PDEs). Its penetration into 3D bi-ventricular electrophysiology (EP) however has been slow, owing to its fundamental limitations to solve PDEs over large or complex solution domains with sharp transitions. In this paper, we propose a new PINN framework to overcome these challenges via three key innovations: 1) a weak-form PDE residual to bypass the challenges of high-order spatial derivatives over irregular spatial domains, 2) a spatial-temporally adaptive training strategy to mitigate the failure of PINN to propagate correct solutions and accelerate convergence, and 3) a sequential learning strategy to enable solutions over longer time domains. We experimentally demonstrated the effectiveness of the presented PINN framework to obtain the complete forward and inverse EP solutions over the 3D bi-ventricular geometry, which is otherwise not possible with vanilla PINN frameworks.
更多查看译文
关键词
Physics-informed neural network,Parameter Inference,Cardiac electrophysiology,Spatial-temporally adaptive training
AI 理解论文
溯源树
样例
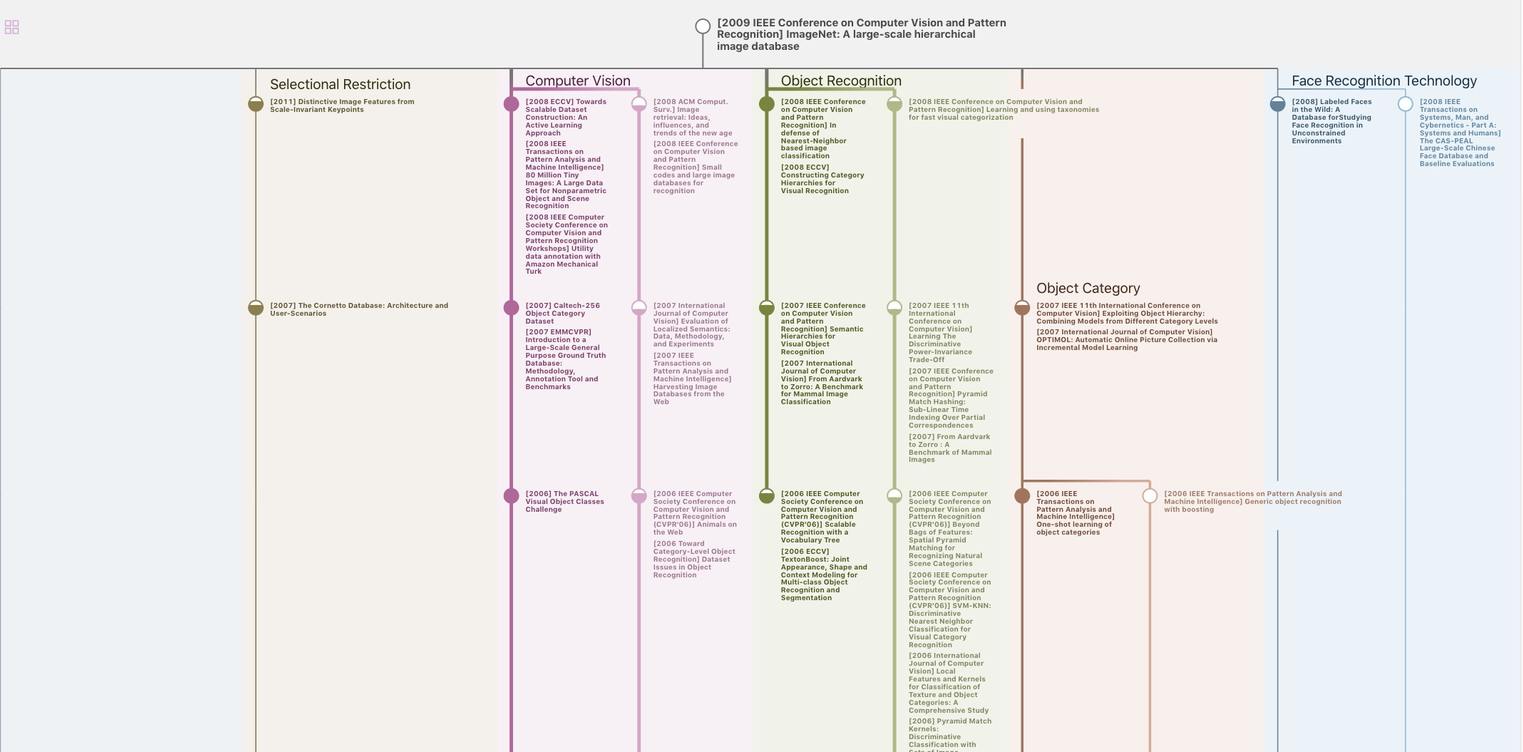
生成溯源树,研究论文发展脉络
Chat Paper
正在生成论文摘要