EdgeMixup: Embarrassingly Simple Data Alteration to Improve Lyme Disease Lesion Segmentation and Diagnosis Fairness
MEDICAL IMAGE COMPUTING AND COMPUTER ASSISTED INTERVENTION, MICCAI 2023, PT IV(2023)
摘要
Lyme disease is a severe skin disease caused by tick bites, which affects hundreds of thousands of people. One task in diagnosing Lyme disease is lesion segmentation, i.e., separating benign skin from lesions, which can not only help clinicians to focus on lesions but also improve downstream tasks such as disease classification. However, it is challenging to segment Lyme disease lesions due to the lack of well-segmented, labeled Lyme datasets and the nature of Lyme, e.g., the typical bull's eye lesion and its closeness to normal skin. In this paper, we design a simple yet novel data preprocessing and alteration method, called EdgeMixup, to help segment Lyme lesions on imbalanced training datasets. The key insight is to deploy a linear combination of lesion edge, either detected or computed, and the source image highlights the affected lesion area so that a learning model focuses more on the preserved lesion structure instead of skin tone, thus iteratively improving segmentation performance. Additionally, the improved edge from lesion segmentation can be further used for Lyme disease classification-e.g., in differentiating Lyme from other similar lesions including tinea corporis and herpes zoster-with improved model fairness on different subpopulations.
更多查看译文
AI 理解论文
溯源树
样例
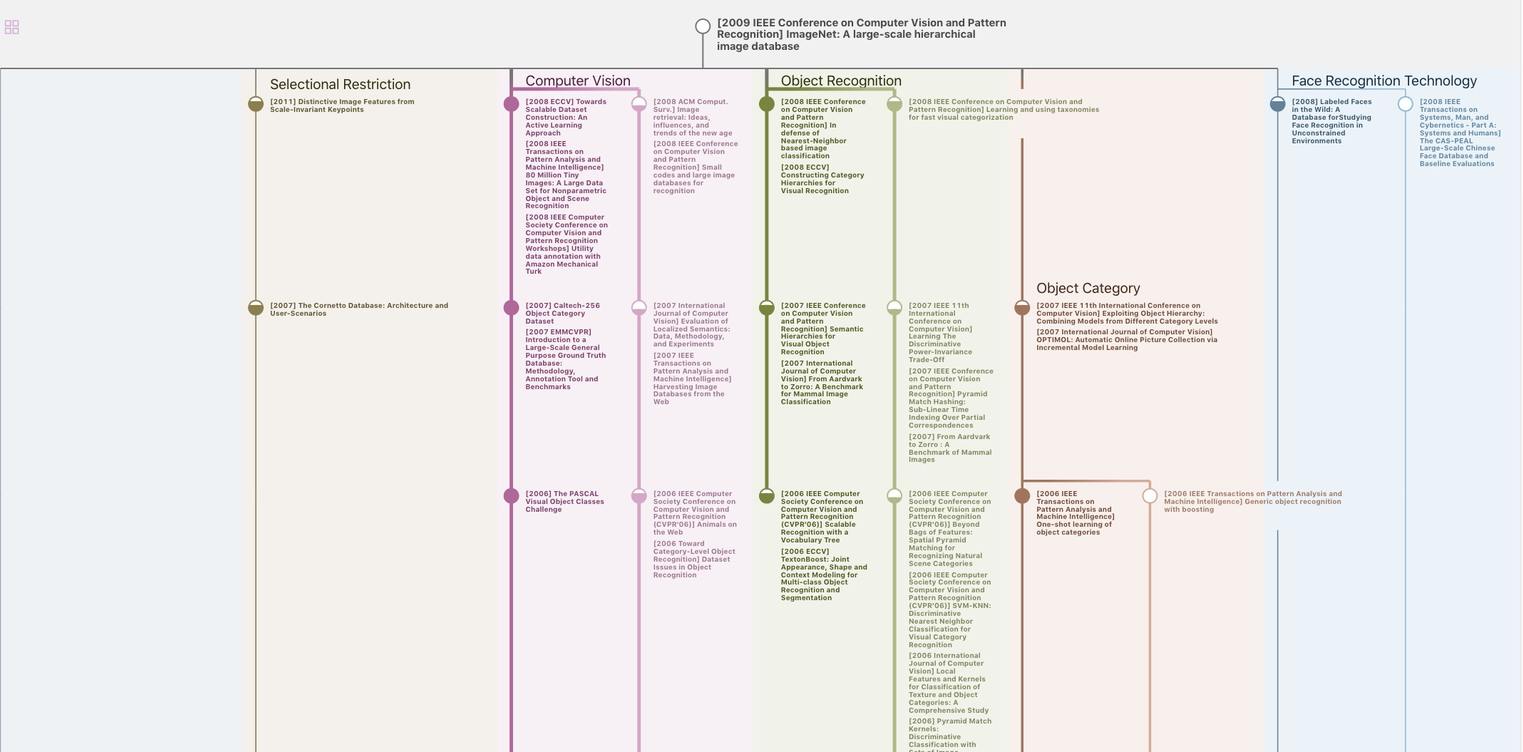
生成溯源树,研究论文发展脉络
Chat Paper
正在生成论文摘要