Brain Age Prediction Based on Head Computed Tomography Segmentation
MACHINE LEARNING IN CLINICAL NEUROIMAGING, MLCN 2023(2023)
摘要
Accurate estimation of an individual's brain age holds significant potential in understanding brain development, aging, and neurological disorders. Despite the widespread availability of head computed tomography (CT) images in clinical settings, limited research has been dedicated to predicting brain age within this modality, often constrained to narrow age ranges or substantial disparities between predicted and chronological age. To address this gap, our work introduces a novel machine learning-based approach for predicting brain age using interpretable features derived from head CT segmentation. By compiling an extensive input set of characteristics including gray matter volume, white matter, cerebrospinal fluid, bone, and soft tissue, we were able to test several linear and non-linear models. Across the entire dataset, our model achieved a mean absolute error (MAE) of 6.70 years in predicting brain age. Remarkably, the relationship between bone and gray matter, as well as the volume of cerebrospinal fluid, were identified as the most pivotal features for precise brain age estimation. To summarize, our proposed methodology exhibits encouraging potential for predicting brain age using head CT scans and offers a pathway to increasing the interpretability of brain age prediction models. Future research should focus on refining and expanding this methodology to improve its clinical application and extend its impact on our understanding of brain aging and related disorders.
更多查看译文
关键词
Brain age,head computed tomography,machine learning,aging
AI 理解论文
溯源树
样例
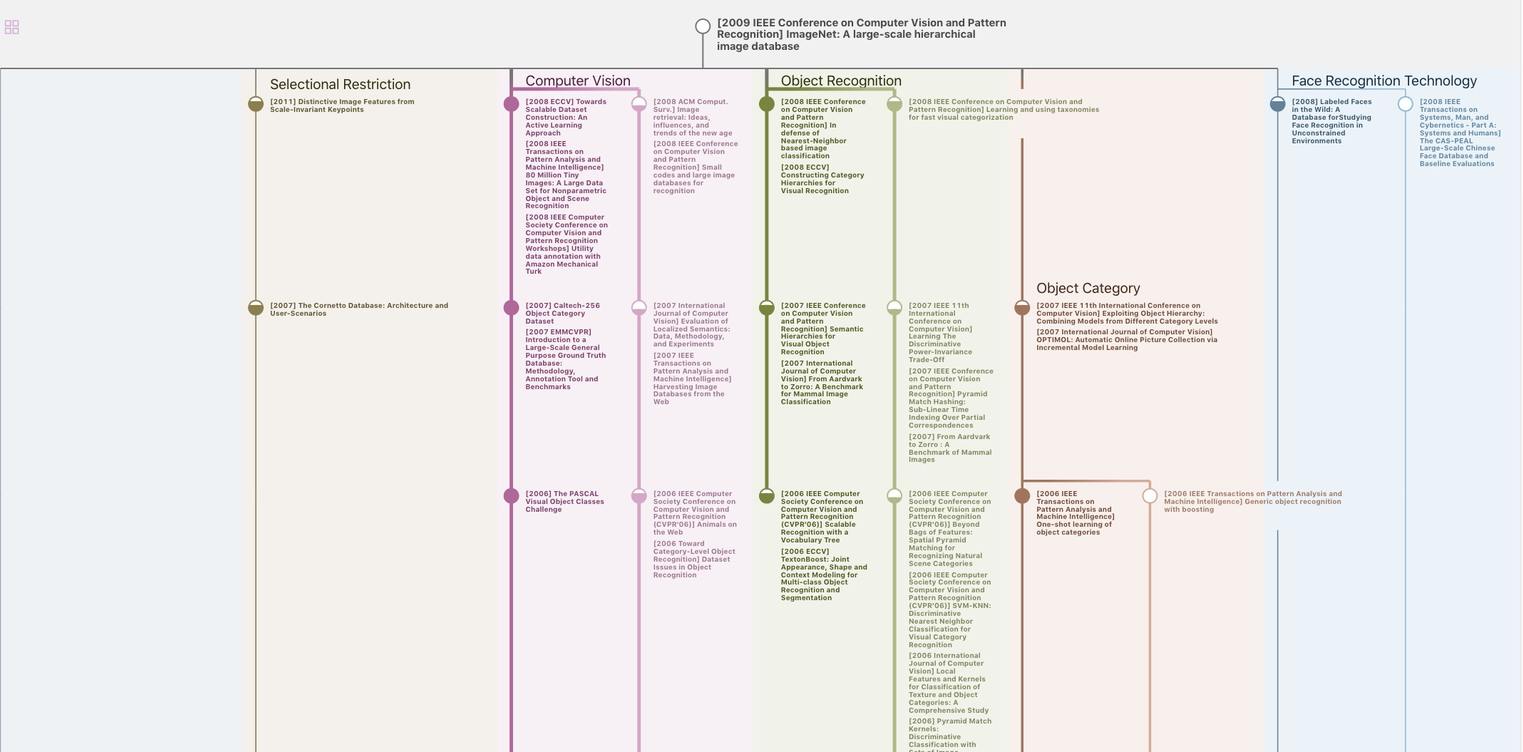
生成溯源树,研究论文发展脉络
Chat Paper
正在生成论文摘要