Dose Guidance for Radiotherapy-Oriented Deep Learning Segmentation
MEDICAL IMAGE COMPUTING AND COMPUTER ASSISTED INTERVENTION, MICCAI 2023, PT IX(2023)
摘要
Deep learning-based image segmentation for radiotherapy is intended to speed up the planning process and yield consistent results. However, most of these segmentation methods solely rely on distribution and geometry-associated training objectives without considering tumor control and the sparing of healthy tissues. To incorporate dosimetric effects into segmentation models, we propose a new training loss function that extends current state-of-the-art segmentation model training via a dose-based guidance method. We hypothesized that adding such a dose-guidance mechanism improves the robustness of the segmentation with respect to the dose (i.e., resolves distant outliers and focuses on locations of high dose/dose gradient). We demonstrate the effectiveness of the proposed method on Gross Tumor Volume segmentation for glioblastoma treatment. The obtained dosimetry-based results show reduced dose errors relative to the ground truth dose map using the proposed dosimetry-segmentation guidance, outperforming state-of-the-art distribution and geometry-based segmentation losses.
更多查看译文
关键词
Segmentation,Radiotherapy,Dose Guidance,Deep Learning
AI 理解论文
溯源树
样例
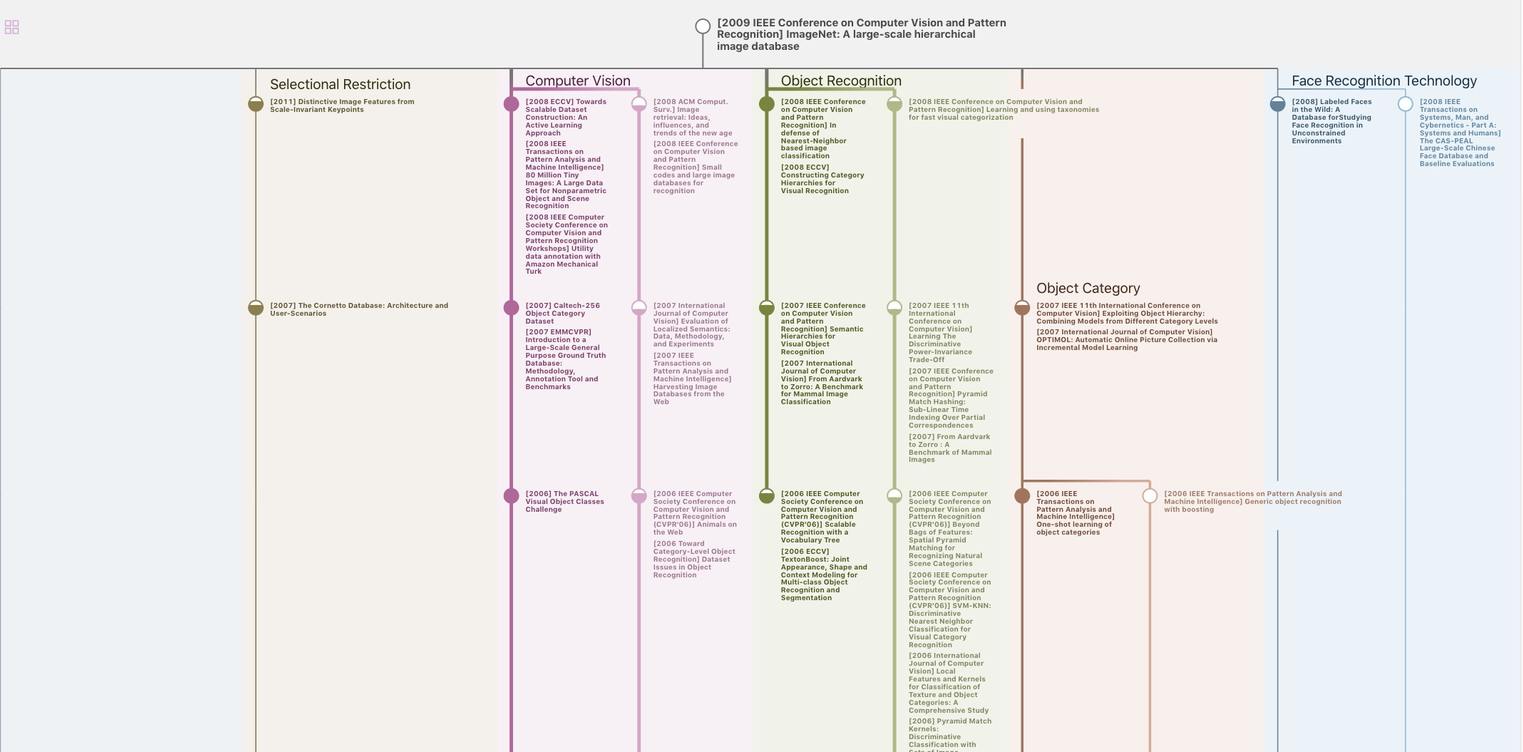
生成溯源树,研究论文发展脉络
Chat Paper
正在生成论文摘要