Improving Outcome Prediction of Pulmonary Embolism by De-biased Multi-modality Model
MEDICAL IMAGE COMPUTING AND COMPUTER ASSISTED INTERVENTION, MICCAI 2023, PT V(2023)
摘要
Bias in healthcare negatively impacts marginalized populations with lower socioeconomic status and contributes to healthcare inequalities. Eliminating bias in AI models is crucial for fair and precise medical implementation. The development of a holistic approach to reducing bias aggregation in multimodal medical data and promoting equity in healthcare is highly demanded. Racial disparities exist in the presentation and development of algorithms for pulmonary embolism (PE), and deep survival prediction model can be de-biased with multimodal data. In this paper, we present a novel survival prediction (SP) framework with demographic bias disentanglement for PE. The CTPA images and clinical reports are encoded by the state-of-the-art backbones pretrained with large-scale medical-related tasks. The proposed de-biased SP modules effectively disentangle latent race-intrinsic attributes from the survival features, which provides a fair survival outcome through the survival prediction head. We evaluate our method using a multimodal PE dataset with time-to-event labels and race identifications. The comprehensive results show an effective de-biased performance of our framework on outcome predictions.
更多查看译文
关键词
Pulmonary Embolism,Deep Survival Prediction,De-Bias learning,Multi-modal learning
AI 理解论文
溯源树
样例
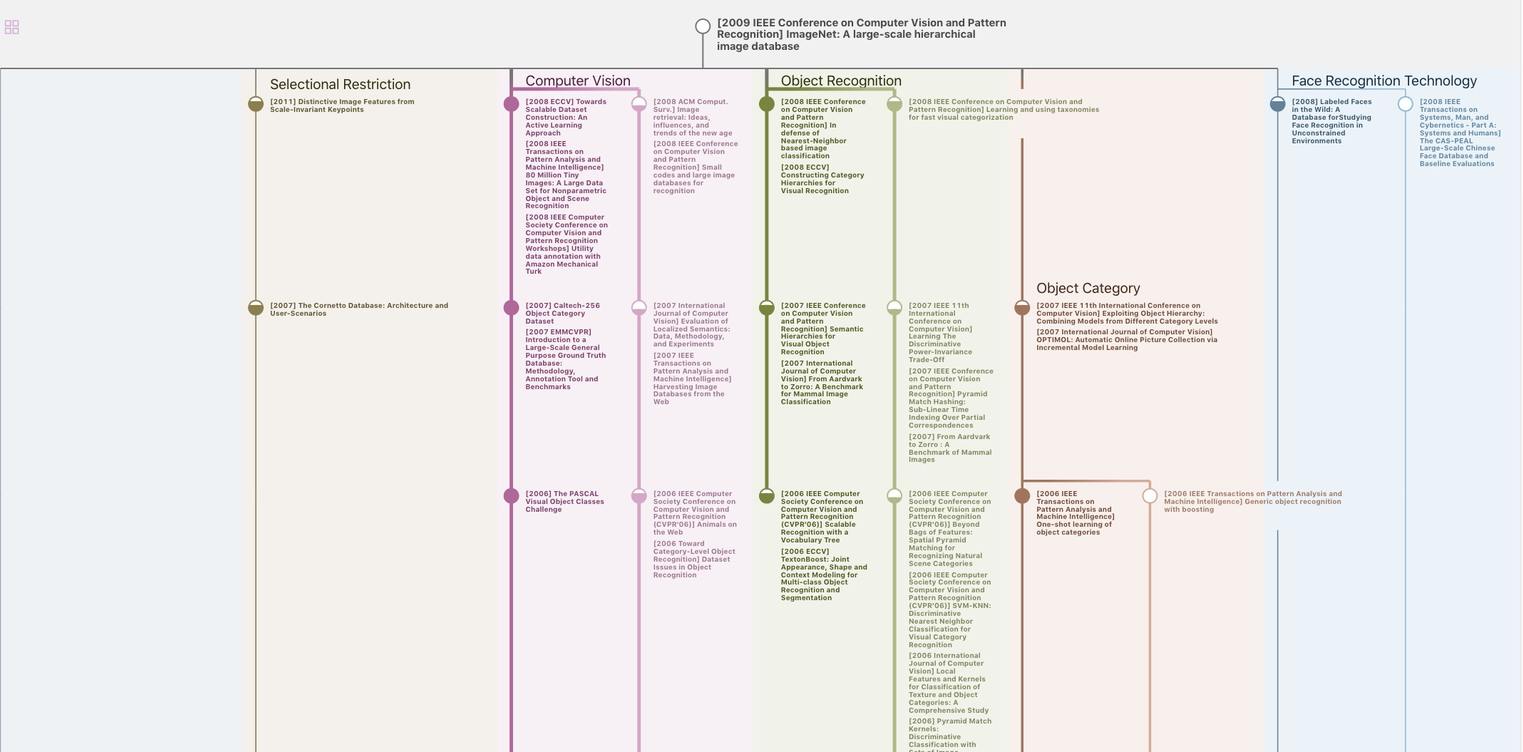
生成溯源树,研究论文发展脉络
Chat Paper
正在生成论文摘要