A General Stitching Solution for Whole-Brain 3D Nuclei Instance Segmentation from Microscopy Images
MEDICAL IMAGE COMPUTING AND COMPUTER ASSISTED INTERVENTION, MICCAI 2023, PT IV(2023)
摘要
High-throughput 3D nuclei instance segmentation (NIS) is critical to understanding the complex structure and function of individual cells and their interactions within the larger tissue environment in the brain. Despite the significant progress in achieving accurate NIS within small image stacks using cutting-edge machine learning techniques, there has been a lack of effort to extend this approach towards whole-brain NIS from light-sheet microscopy. This critical area of research has been largely overlooked, despite its importance in the neuroscience field. To address this challenge, we propose an efficient deep stitching neural network built upon a knowledge graph model characterizing 3D contextual relationships between nuclei. Our deep stitching model is designed to be agnostic, enabling existing limited methods (optimized for image stack only) to overcome the challenges of whole-brain NIS, particularly in addressing the issue of inter- and intra-slice gaps. We have evaluated the NIS accuracy on top of three state-of-the-art deep models with 128x128x64 image stacks, and visualized results in both inter- and intra-slice gaps of whole brain. With resolved gap issues, our deep stitchingmodel enables the whole-brain NIS (gigapixel-level) on entry-level GPU servers within 27 h.
更多查看译文
关键词
Image stitching,3D microscopy image,Whole-brain nucleus instance segmentation,Graph neural network
AI 理解论文
溯源树
样例
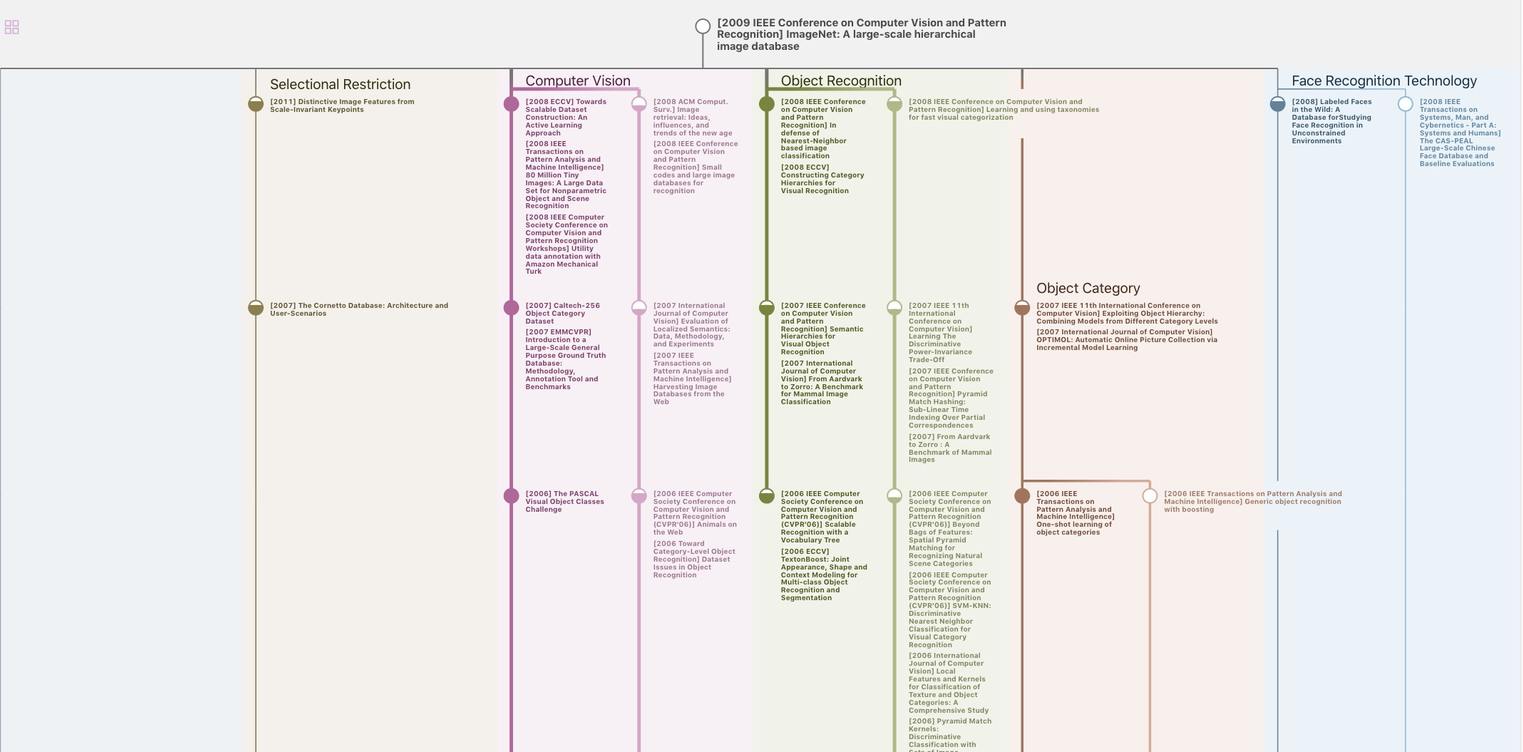
生成溯源树,研究论文发展脉络
Chat Paper
正在生成论文摘要