ALL-IN: ALocal GLobal Graph-Based DIstillatioN Model for Representation Learning of Gigapixel Histopathology Images With Application In Cancer Risk Assessment.
MICCAI (6)(2023)
摘要
The utility of machine learning models in histopathology image analysis for disease diagnosis has been extensively studied. However, efforts to stratify patient risk are relatively under-explored. While most current techniques utilize small fields of view (so-called local features) to link histopathology images to patient outcome, in this work we investigate the combination of global (i.e., contextual) and local features in a graph-based neural network for patient risk stratification. The proposed network not only combines both fine and coarse histological patterns but also utilizes their interactions for improved risk stratification. We compared the performance of our proposed model against the state-of-the-art (SOTA) techniques in histopathology risk stratification in two cancer datasets. Our results suggest that the proposed model is capable of stratifying patients into statistically significant risk groups ( $$p < 0.01$$ across the two datasets) with clinical utility while competing models fail to achieve a statistical significance endpoint ( $$p = 0.148-0.494$$ ).
更多查看译文
关键词
gigapixel histopathology images,cancer risk assessment,representation learning,graph-based
AI 理解论文
溯源树
样例
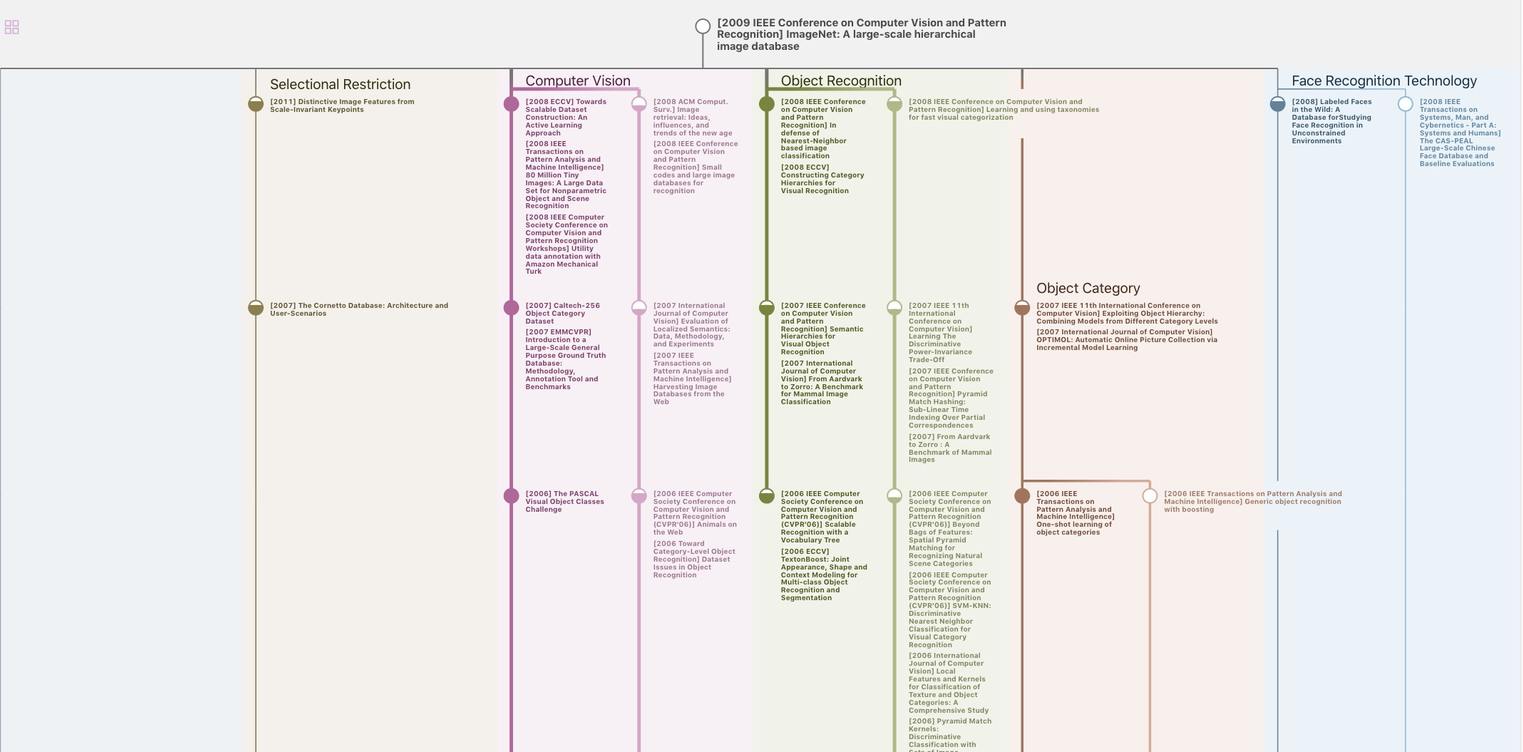
生成溯源树,研究论文发展脉络
Chat Paper
正在生成论文摘要