Using Training Samples as Transitive Information Bridges in Predicted 4D MRI.
MILLanD@MICCAI(2023)
摘要
The lack of real-time techniques for monitoring respiratory motion impairs the development of guidance systems for image-guided interventions. Recent works show that U-Net based real-time 4D MRI prediction methods are promising, but prone to bad image quality when small training data sets and inputs with multiple MR contrast are used. To overcome this problem, we propose a more efficient use of the spare training data and re-utilize 2D training samples as a secondary input for construction of transitive information bridges between the navigator slice primary input and the data slice prediction. We thus remove the need for a separate 3D breath-hold MRI with different MR contrast as the secondary input. Results show that our novel construction leads to improved prediction quality with very sparse training data, with a significant decrease in root mean squared error (RMSE) from 0.3 to 0.27 (p < 2.2 e - 16 , d=0.19). Additionally, removing 3D imaging reduces prior acquisition time from 3 to 2 min.
更多查看译文
关键词
mri,transitive information bridges,training samples
AI 理解论文
溯源树
样例
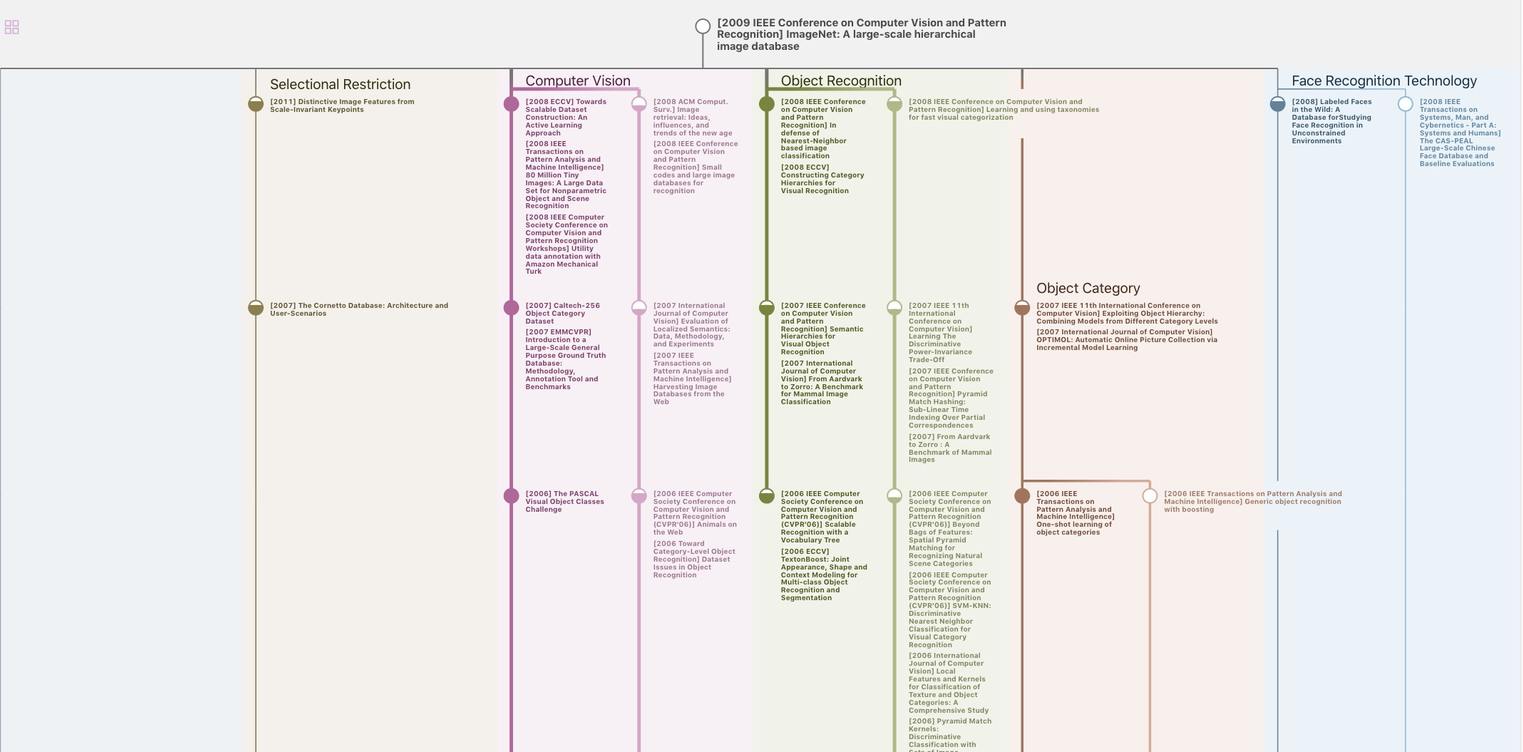
生成溯源树,研究论文发展脉络
Chat Paper
正在生成论文摘要