Neonatal Hypoxic-Ischemic Encephalopathy Grading from Multi-Channel EEG Time-Series Data Using a Fully Convolutional Neural Network
TECHNOLOGIES(2023)
摘要
A deep learning classifier is proposed for grading hypoxic-ischemic encephalopathy (HIE) in neonates. Rather than using handcrafted features, this architecture can be fed with raw EEG. Fully convolutional layers were adopted both in the feature extraction and classification blocks, which makes this architecture simpler, and deeper, but with fewer parameters. Here, two large (335 h and 338 h, respectively) multi-center neonatal continuous EEG datasets were used for training and testing. The model was trained based on weak labels and channel independence. A majority vote method was used for the post-processing of the classifier results (across time and channels) to increase the robustness of the prediction. A dimension reduction tool, UMAP, was used to visualize the model classification effect. The proposed system achieved an accuracy of 86.09% (95% confidence interval: 82.41-89.78%), an MCC of 0.7691, and an AUC of 86.23% on the large unseen test set. Two convolutional neural network architectures which utilized time-frequency distribution features were selected as the baseline as they had been developed or tested on the same datasets. A relative improvement of 23.65% in test accuracy was obtained as compared with the best baseline. In addition, if only one channel was available, the test accuracy was only reduced by 2.63-5.91% compared with making decisions based on the eight channels.
更多查看译文
关键词
hypoxic-ischemic encephalopathy (HIE),EEG,fully convolutional neural network,UMAP
AI 理解论文
溯源树
样例
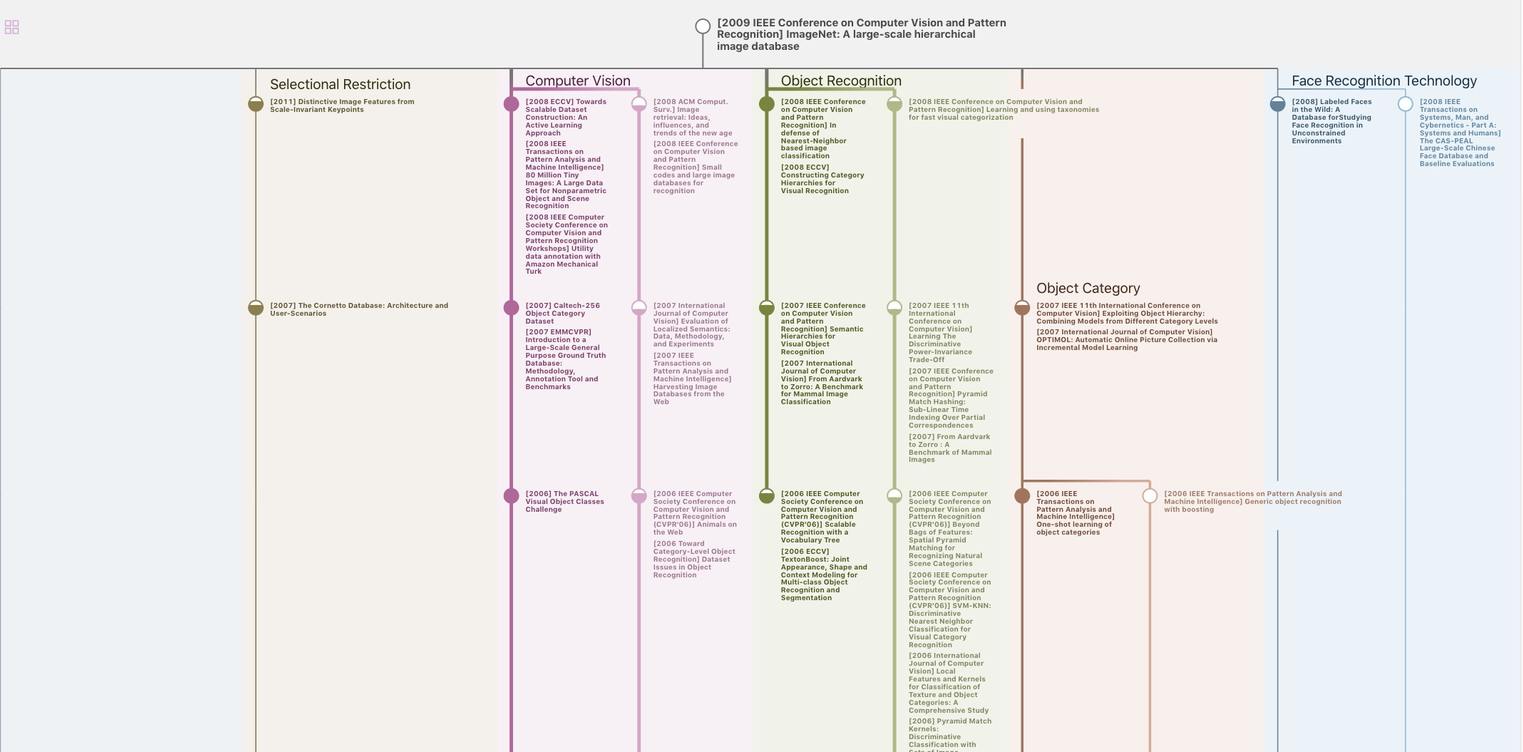
生成溯源树,研究论文发展脉络
Chat Paper
正在生成论文摘要