COVID-19 Lesion Segmentation Framework for the Contrast-Enhanced CT in the Absence of Contrast-Enhanced CT Annotations.
MILLanD@MICCAI(2023)
摘要
Medical imaging is a dynamic domain where new acquisition protocols are regularly developed and employed to meet changing clinical needs. Deep learning models for medical image segmentation have proven to be a valuable tool for medical image processing. Creating such a model from scratch requires a lot of effort in terms of annotating new types of data and model training. Therefore, the amount of annotated training data for the new imaging protocol might still be limited. In this work we propose a framework for segmentation of images acquired with a new imaging protocol(contrast-enhanced lung CT) that does not require annotating training data in the new target domain. Instead, the framework leverages the previously developed models, data and annotations in a related source domain. Using contrast-enhanced lung CT data as a target data we demonstrate that unpaired image translation from the non-contrast enhanced source data, combined with self-supervised pretraining achieves 0.726 Dice Score for the COVID-19 lesion segmentation task on the target data, without the necessity to annotate any target data for the model training.
更多查看译文
关键词
lesion,ct,segmentation,contrast-enhanced,contrast-enhanced
AI 理解论文
溯源树
样例
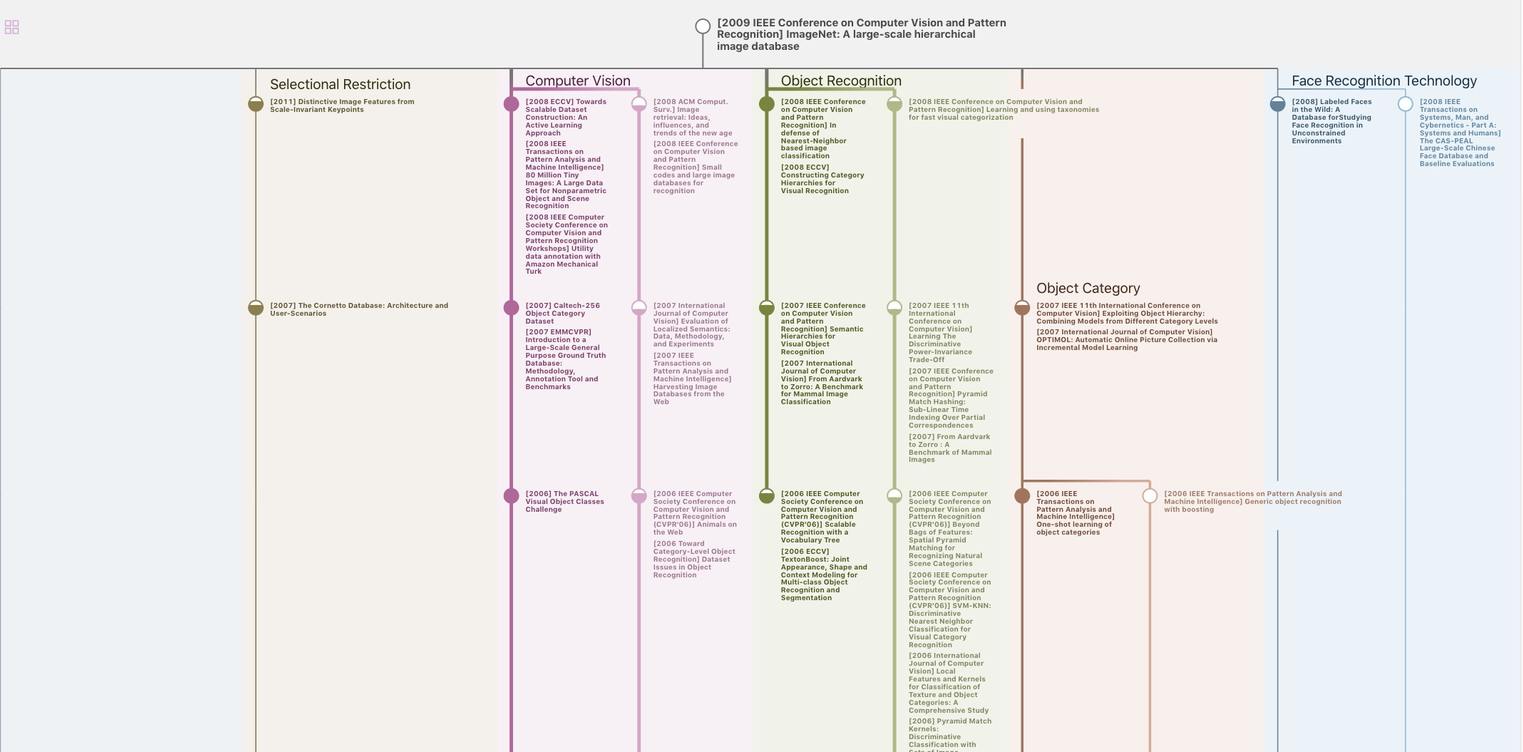
生成溯源树,研究论文发展脉络
Chat Paper
正在生成论文摘要