GSDG: Exploring a Global Semantic-Guided Dual-Stream Graph Model for Automated Volume Differential Diagnosis and Prognosis
MEDICAL IMAGE COMPUTING AND COMPUTER ASSISTED INTERVENTION, MICCAI 2023, PT V(2023)
摘要
Three-dimensional medical images are crucial for the early screening and prognosis of numerous diseases. However, constructing an accurate computer-aided prediction model is challenging when dealing with volumes of different sizes due to numerous slices (native nodes) in a single case and variable-length slice sequence. We propose a Global Semantic-guided Dual-stream Graph model to address this issue. Our approach differs from the existing solution that aligns volumes with varying numbers of slices through downsampling. Instead, we leverage global semantic vectors to guide the grouping of native nodes, construct supernodes, and build dual-stream graphs by incorporating the sequential association of each volume's unique slices and the feature association of global semantic vectors. Specifically, we propose a shared global semantic vectors-based grouping method that aligns the number and the semantic distribution of nodes among different volumes without discarding slices. Furthermore, we construct a dual-stream graph module that enables Graph Convolutional Networks (GCN) to make clinical predictions from computer tomography (CT) volumes through the natural sequence association between native nodes and, simultaneously, the latent feature association between semantic vectors. We provide interpretability by visualizing the distribution of native nodes within each group and weakly-supervised slice localization. The results demonstrate that our method outperforms previous work in diagnostic (96.74%, +2.81%) and prognostic accuracy (84.56%, +1.86%) while being more interpretable, making it a promising approach for medical image analysis scenarios with limited fine-grained annotation.
更多查看译文
关键词
Diagnosis,Prognosis,Semantic-guided grouping,Graph convolutional networks,Lesion localization
AI 理解论文
溯源树
样例
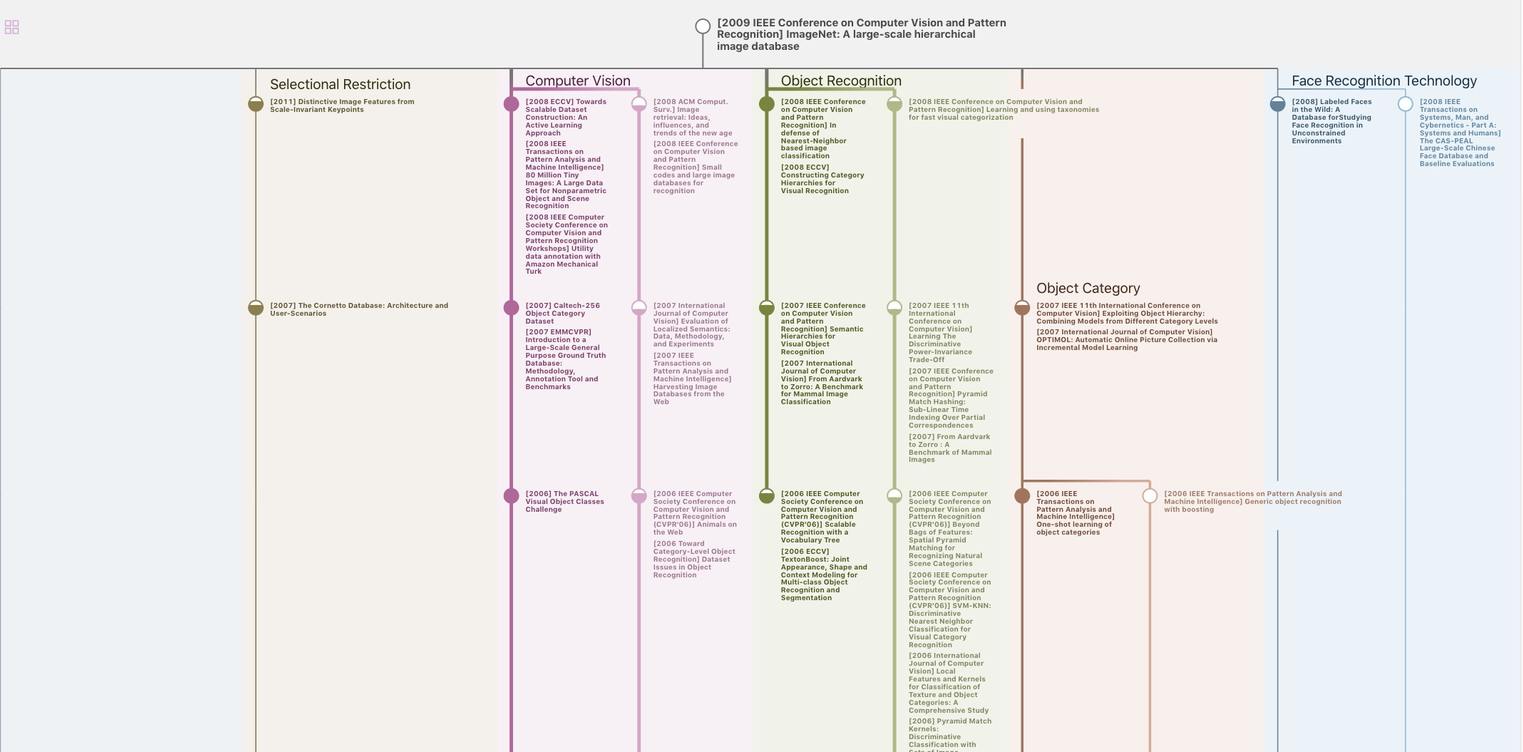
生成溯源树,研究论文发展脉络
Chat Paper
正在生成论文摘要