IIB-MIL: Integrated Instance-Level and Bag-Level Multiple Instances Learning with Label Disambiguation for Pathological Image Analysis
MEDICAL IMAGE COMPUTING AND COMPUTER ASSISTED INTERVENTION, MICCAI 2023, PT VI(2023)
摘要
Digital pathology plays a pivotal role in the diagnosis and interpretation of diseases and has drawn increasing attention in modern healthcare. Due to the huge gigapixel-level size and diverse nature of whole-slide images (WSIs), analyzing them through multiple instance learning (MIL) has become a widely-used scheme, which, however, faces the challenges that come with the weakly supervised nature of MIL. Conventional MIL methods mostly either utilized instance-level or bag-level supervision to learn informative representations from WSIs for downstream tasks. In this work, we propose a novel MIL method for pathological image analysis with integrated instance-level and bag-level supervision (termed IIB-MIL). More importantly, to overcome the weakly supervised nature of MIL, we design a label-disambiguation-based instance-level supervision for MIL using Prototypes and Confidence Bank to reduce the impact of noisy labels. Extensive experiments demonstrate that IIB-MIL outperforms state-of-the-art approaches in both benchmarking datasets and addressing the challenging practical clinical task. The code is available at https://github.com/TencentAILabHealthcare/IIB-MIL.
更多查看译文
关键词
computational pathology,multi-instance learning,label disambiguation,prototype,confidence bank
AI 理解论文
溯源树
样例
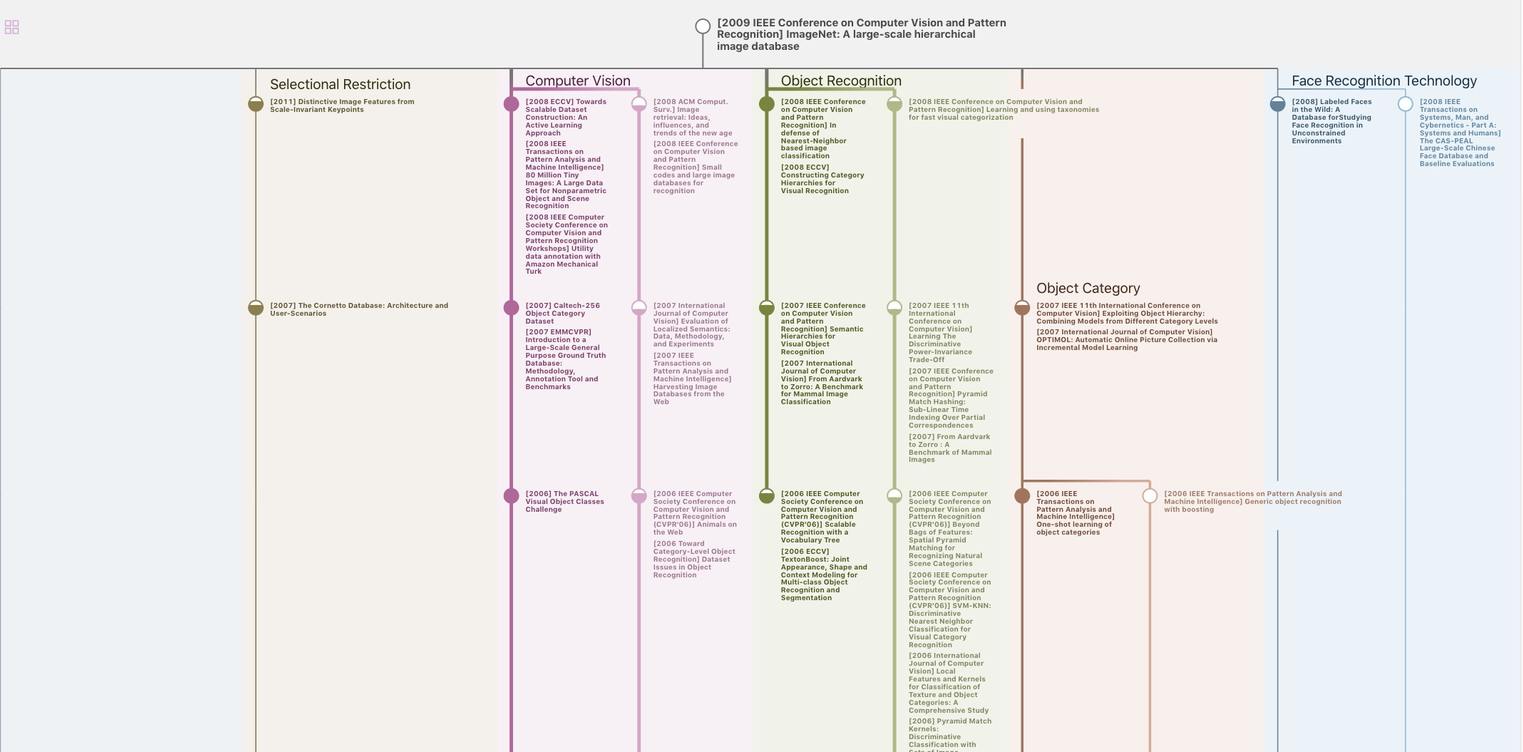
生成溯源树,研究论文发展脉络
Chat Paper
正在生成论文摘要