SADEM: An Effective Supervised Anomaly Detection Ensemble Model for Alert Account Detection
JOURNAL OF INFORMATION SCIENCE AND ENGINEERING(2023)
摘要
Anomaly detection has been an important research topic for a long time and has been applied to many real-world applications. However, due to the high cost of manually getting the instance label, researchers mostly resort to unsupervised or semi-supervised learning approaches. The supervised learning method has rarely been used in anomaly detection tasks. In this paper, we proposed a supervised learning ensemble method to detect alert accounts among transaction data. We solve the problem of low-confident predictions when the anomalies reside within normal data points. The ensemble model comprises the LightGBM and Multi-layer Perceptron (MLP) to synergize machine learning and neural network models. The proposed model preserves the result of high-confident predictions and improves the performance of low-confident predictions with the new features generated from encoding the leaf node of GBDT (Gradient Boosting Decision Tree). Our experiments on a real-world dataset show the effectiveness of the model when compared with the state-of-the-art methods.
更多查看译文
关键词
alert account detection,anomaly detection,imbalanced classifica-tion,supervised learning,low-confident predictions
AI 理解论文
溯源树
样例
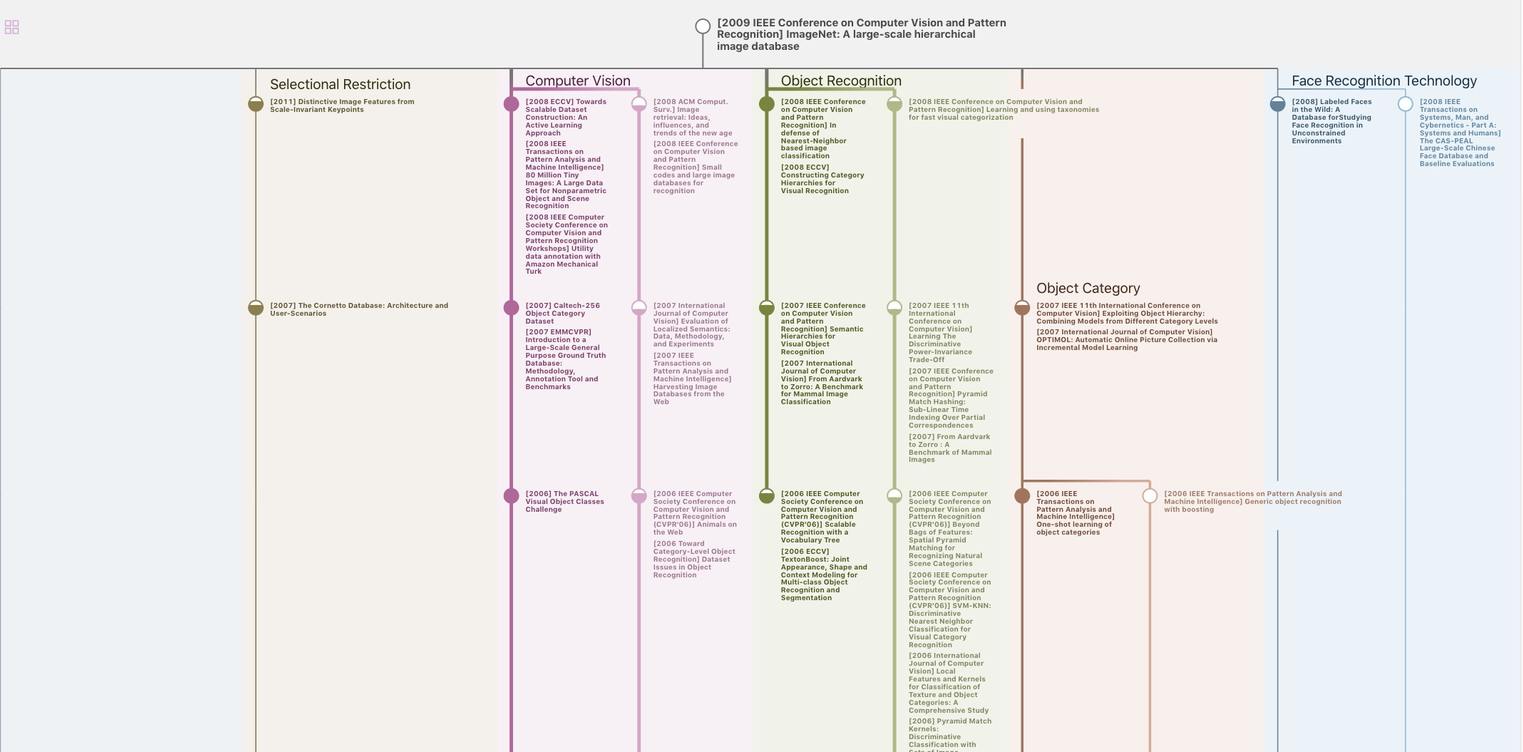
生成溯源树,研究论文发展脉络
Chat Paper
正在生成论文摘要