DL-2P-DDoSADF: Deep learning-based two-phase DDoS attack detection framework
J. Inf. Secur. Appl.(2023)
摘要
In today's tech-driven world, while Internet-based applications drive social progress, their architectural weaknesses, inadequate security measures, lack of network segmentation, unsecured IoT devices etc., offer ample opportunities for attackers to launch a multitude of attacks on their services. Despite numerous security solutions, the frequent changes in the methods employed by attackers present a challenge for security systems to stay up to date. Moreover, the existing machine learning approaches are confined to known attack patterns and necessitate annotated data. This paper proposes a deep learning-based two-phase DDoS attack detection framework named DL-2P-DDoSADF. The proposed framework has been validated using the CICDDoS2019 and DDoS-AT-2022 datasets. In the first phase, Autoencoder (AE) has been trained using the legitimate traffic and threshold value has been set using Reconstruction Error (RE). The test data comprising legitimate and attack traffic has been used to validate the proposed approach efficacy. The initial phase entails utilizing a trained AE model to enable the passage of predicted legitimate traffic through the network. In contrast, the predicted attack traffic proceeds to the second phase to classify the type of attack it represents. The performance and efficacy of various deep learning approaches: Deep Neural Network (DNN), Long Short-Term Memory (LSTM) and Gated Recurrent Units (GRU) are compared as part of the second phase. The autoencoder displayed an accuracy level of 99% in detecting both datasets in the initial phase. It has been observed that the DNN produced an overall accuracy of 97% and 96% for the CICDDoS2019 and DDoS-AT-2022 datasets, respectively, for multiclass classification. The DNN model performed better than LSTM and GRU models in the second phase.
更多查看译文
关键词
Deep learning,Autoencoder,Reconstruction error,Deep neural network,CICDDoS2019 dataset,DDoS-AT 2022 dataset
AI 理解论文
溯源树
样例
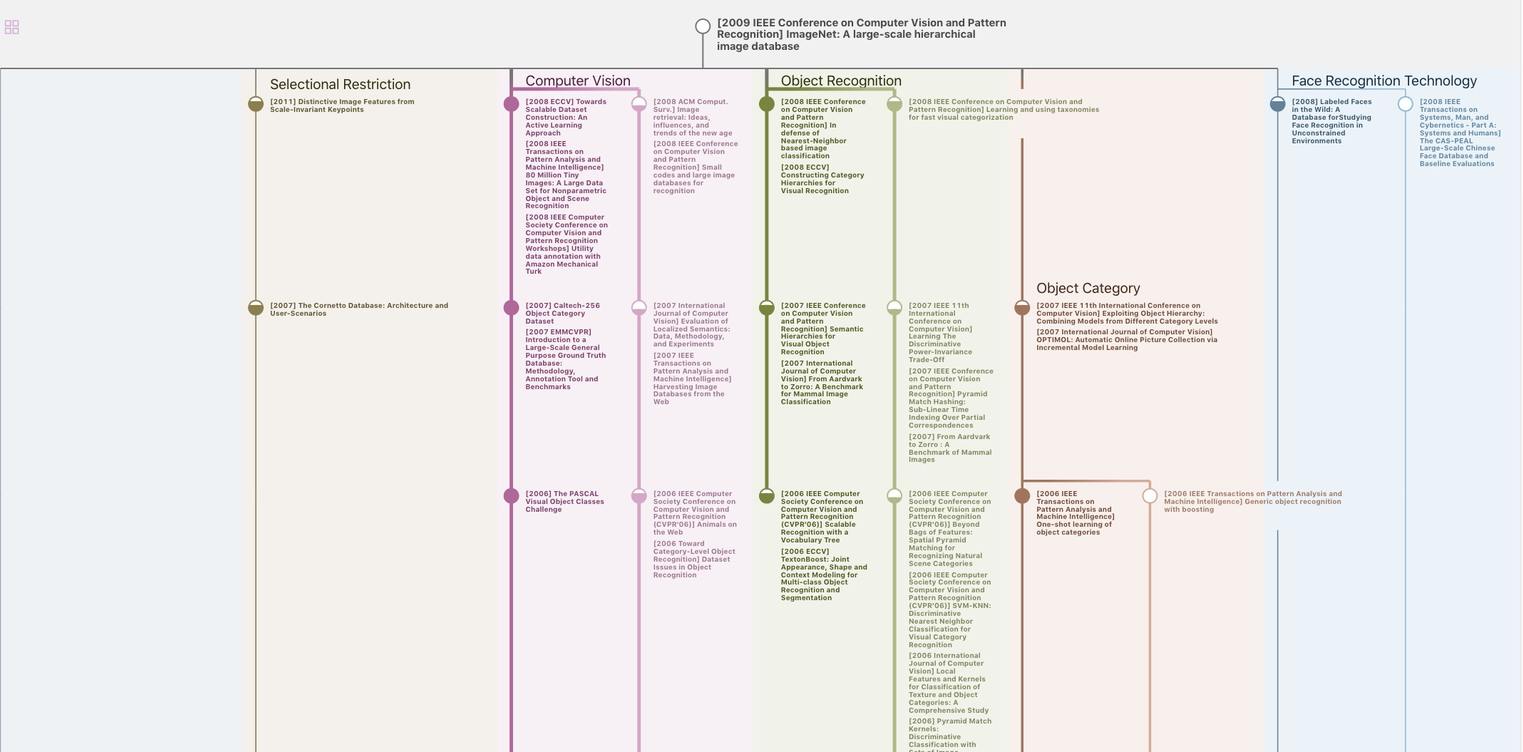
生成溯源树,研究论文发展脉络
Chat Paper
正在生成论文摘要