A Ground-Roll Separation Method Based on Neural Networks With Morphological Similarity Loss.
IEEE Geosci. Remote. Sens. Lett.(2023)
摘要
Ground-roll is a typical Rayleigh-type interference noise in field seismic data, which is characterized by low frequency, low velocity, and high amplitude. Since it will interfere with effective seismic signals and severely degrade the signal-to-noise ratio of observed seismic records, many approaches have been developed for ground-roll attenuation or separation. In this letter, we proposed an improved ground-roll separation algorithm through the combination of deep-learning-based low-frequency generation and dictionary-learning-based low-frequency reconstruction. Moreover, to utilize the interband morphological similarity prior to seismic response, we introduce the morphological similarity constraint into the learning approach of pseudo-low-frequency generation networks. Experiments demonstrate that compared to previous methods, the introduced morphological similarity loss (MSLoss) can effectively improve the quality of generated pseudo-low-frequency signals, which results in better low-frequency reflection reconstruction and ground-roll separation performances.
更多查看译文
关键词
Dictionary learning,ground-roll separation,morphological similarity,neural networks
AI 理解论文
溯源树
样例
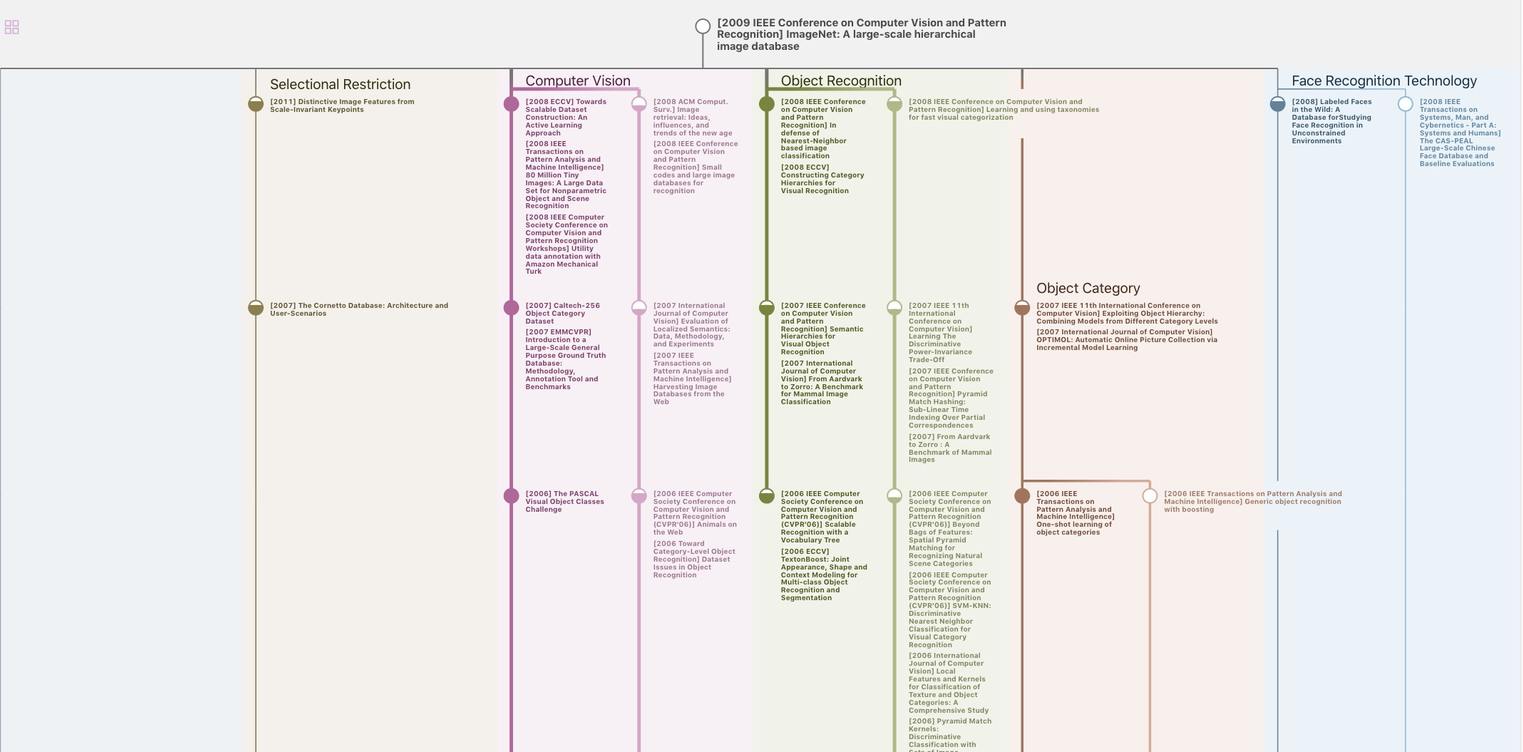
生成溯源树,研究论文发展脉络
Chat Paper
正在生成论文摘要