A Novel Digital Twin (DT) Model Based on WiFi CSI, Signal Processing and Machine Learning for Patient Respiration Monitoring and Decision-Support.
IEEE Access(2023)
摘要
Digital Twin (DT) in Healthcare 4.0 (H4.0) presents a digital model of the patient with all its biological properties and characteristics. One of the application areas is patient respiration monitoring for enhanced patient care and decision support to healthcare professionals. Obtrusive methods of patient monitoring create hindrances in the patient's daily routine. This research presents a novel Respiration DT (ResDT) model based on Wi-Fi Carrier State Information (CSI), improved signal processing, and Machine Learning (ML) algorithms for monitoring and classification (binary and multi-class) of patient respiration. A Wi-Fi sensor ESP32 with Wi-Fi CSI was utilized for the collection of respiration data. This provides an added advantage of unobtrusive monitoring of patient vital signs. The Patient's Breaths Per Minute (BPM) is estimated from raw sensor data through the integration of multiple signal processing methodologies for denoising (smoothing and filtering) and dimensionality reduction (PCA, SVM, EMD, EMD-PCA). Multiple filters and dimensionality reduction methodologies are compared for accurate BPM estimation. The elliptical filter provides a relatively better estimation of the BPM with 87.5% accurate estimation as compared to other bandpass filters such as Butterworth (BF), Chebyshev type 1 Filter (CH1), Chebyshev type 2 Filter (CH2), and wavelet Decomposition (62.5%, 75%, 68.75%, and 75% respectively). Principal Component Analysis (PCA) was performed to provide better dimensionality reduction with 87.5% accurate BPM values compared to EMD, SVD, and EMD-PCA (57%, 44%, and 44% respectively). Additionally, the fine tree algorithm, from the implemented 21 ML supervised classification algorithms with K-fold cross-validation, was observed to be the optimal choice for multi-class and binary-class classification problems in the presented ResDT model with 96.9% and 95.8% accuracy respectively.
更多查看译文
关键词
Machine learning, Principal component analysis, Representation learning, Wireless fidelity, Biological processes, Patient monitoring, Biomedical signal processing, Digital twin, machine learning (ML), principle component analysis (PCA), respiration rate estimation, signal processing, unobtrusive Wi-Fi sensor
AI 理解论文
溯源树
样例
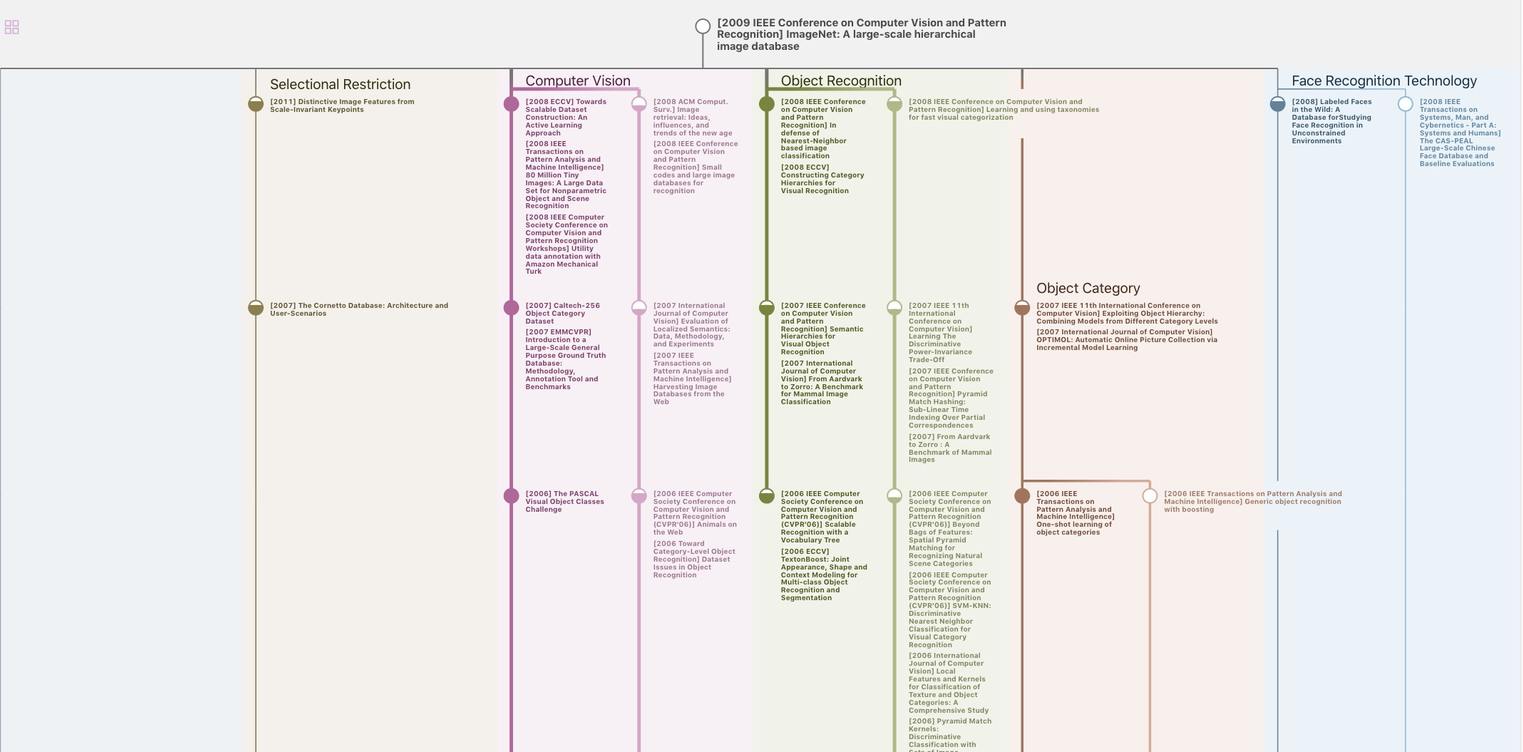
生成溯源树,研究论文发展脉络
Chat Paper
正在生成论文摘要