CLiCK: Continual Learning Exploiting Intermediate Network Models With A Slack Class.
IEEE Access(2023)
摘要
We propose a continual learning (CL) method (called CLiCK), a hybrid of an architecture-based approach that increments a model when it detects that the dataset characteristics have changed significantly, and a rehearsal-based approach that exploits an episodic memory to store past dataset samples. The proposed CLiCK makes the final decision by taking the ensemble of the inference results for the current and a series of past models. A novelty of CLiCK is to introduce a concept for a slack class, which is an auxiliary class to represent unseen or undetermined classes that do not belong to the current dataset. Because the models trained with a slack class have the capability to differentiate between the classes that they were trained on and unseen classes, the inference results of the models that do not have knowledge about input can be automatically neglected in the final decision. Our experiments show that the proposed CLiCK achieves performance comparable to joint learning, which uses the entire dataset for each task, in domain-incremental scenarios on the MNIST dataset. In class-incremental scenarios on the MNIST and CIFAR-100 datasets, CLiCK outperforms other existing CL methods significantly.
更多查看译文
关键词
Continual learning, episodic memory, intermediate models, slack class
AI 理解论文
溯源树
样例
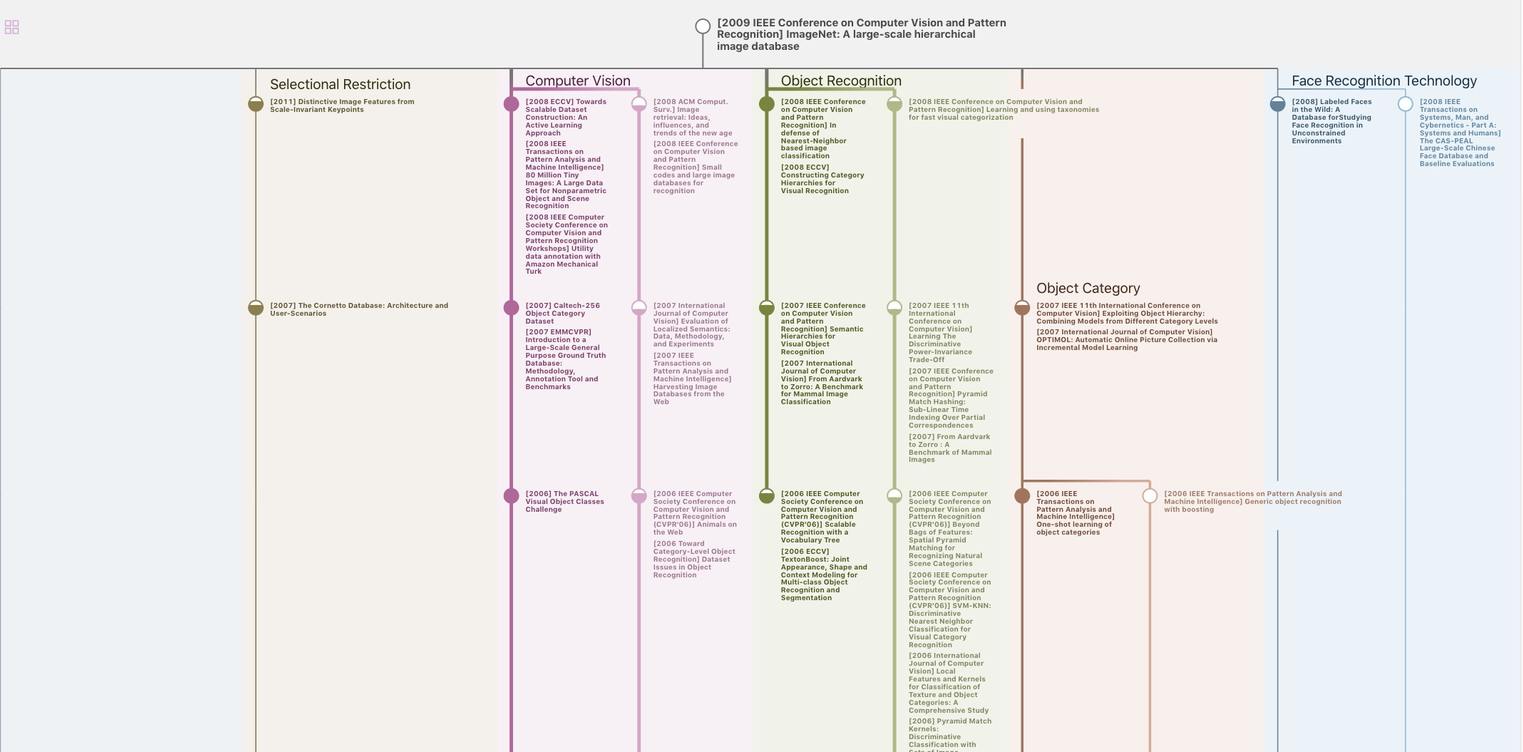
生成溯源树,研究论文发展脉络
Chat Paper
正在生成论文摘要