Signed attention based graph neural network for graphs with heterophily.
Neurocomputing(2023)
摘要
Graph Neural Networks (GNNs) are an effective deep learning methodology used to encode graph-structured data. Several popular GNNs have demonstrated excellent results in various downstream tasks, including node classification and visualization, especially when homophily occurs in graphs where connected nodes may share identical features or labels. Although recent models can extend GNNs beyond homophily, two fundamental weaknesses limit their expressive ability. First, most of the existing GNNs fail to adaptively aggregate information from different neighbors, especially negative information from different classes. Even worse, they fail to encode the positional information, leading to the inevitable conflation of information from different neighborhoods that should be specified with different importance. To overcome these limitations, we propose a novel graph neural network model based on the Signed Attention mechanism (SA), namely SAGNN. Specifically, the signed attention mechanism is presented to adaptively learn the complex relationship between exact neighboring nodes. Compared with the original non-negative attention mechanism, SA aggregates information from both positive and negative neighbors, especially on graphs with heterophily. Furthermore, we propose an Exactly Higher-Order Neighborhood Aggregation that implicitly encodes positional information to specify the importance of different neighborhoods. Extensive experiments conducted on eight benchmark datasets demonstrate that the proposed SAGNN model learns more robust and expressive representations, and achieves superior performance on node classification and visualization tasks.
更多查看译文
关键词
Graph neural networks,Signed attention mechanism,Exactly higher-order neighborhood aggregation,Heterophily graph
AI 理解论文
溯源树
样例
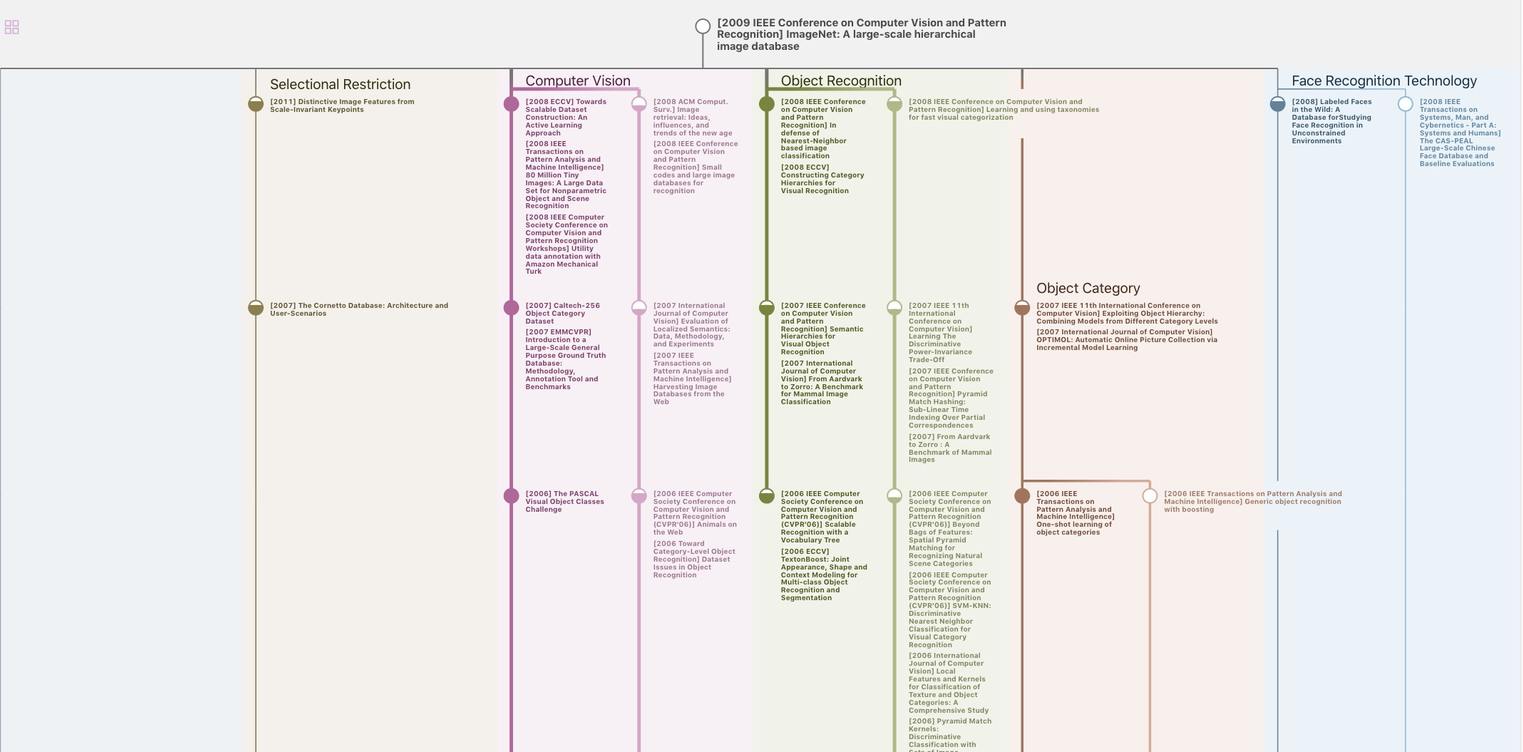
生成溯源树,研究论文发展脉络
Chat Paper
正在生成论文摘要