Deep Learning-Based Recognition of Unsafe Acts in Manufacturing Industry.
IEEE Access(2023)
摘要
Despite technological progress and the tendency for automation, the majority of manufacturing workplaces still rely on human labor. Although industrial tasks are frequently composed of simple operator actions, non-ergonomic execution of such repetitive tasks has been reported as the primary cause of musculoskeletal disorders. Considering the sizes of manufacturing halls and large numbers of employees, there is an increasing need for tools that can improve the recognition of unsafe acts. Herein, a deep learning-based procedure for pose safety assessment is proposed and validated using monocular videos captured with a conventional IP camera. The two key composing components of the proposed pipeline are the three-dimensional (3D) pose estimator and mesh classifier. The proposed method was validated experimentally by considering three different methodologically selected industrial tasks: a laborious task that requires all-body effort (pushing and pulling), a task that requires an upper-limb action comprising intensive interaction and motion control (drilling), and a typical collaborative task (polishing with a collaborative robot with variable mechanical impedance). Accuracies of 84.67%, 92%, and 98%, respectively, were achieved. Besides higher accuracy, the proposed method has shown practical advantages over existing alternatives based on analyzing the parameters derived from the human poses. Particularly, we report that the proposed procedure is generic, and it works directly with 3D human body poses, which significantly increases applicability while reducing the complexity and effort needed for data annotation and output interpretation by non-experts.
更多查看译文
关键词
unsafe acts,recognition,industry,deep,learning-based
AI 理解论文
溯源树
样例
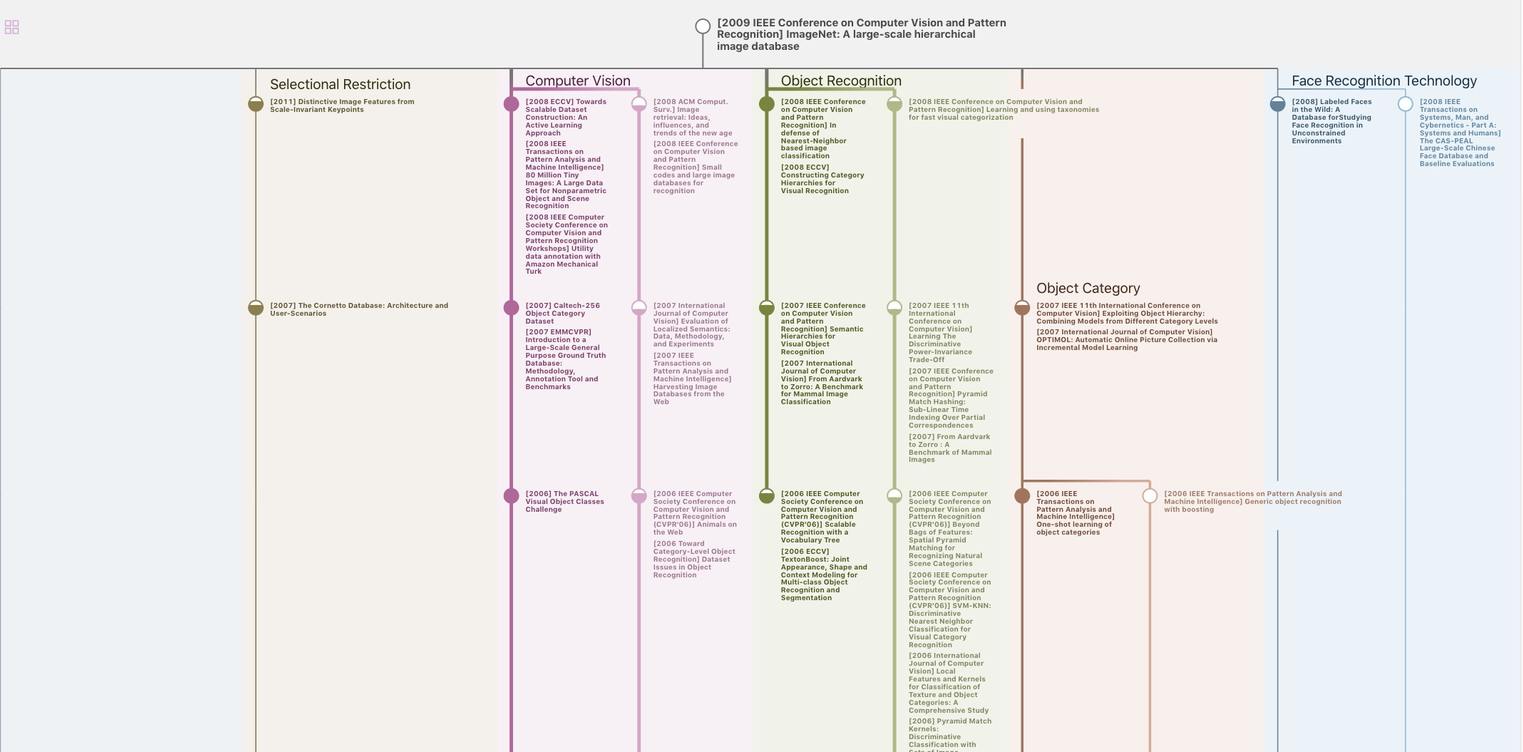
生成溯源树,研究论文发展脉络
Chat Paper
正在生成论文摘要