Latent Stochastic Differential Equations for Change Point Detection
IEEE Access(2023)
摘要
Automated analysis of complex systems based on multiple readouts remains a challenge. Change point detection algorithms are aimed to locating abrupt changes in the time series behaviour of a process. In this paper, we present a novel change point detection algorithm based on Latent Neural Stochastic Differential Equations (SDE). Our method learns a non-linear deep learning transformation of the process into a latent space and estimates a SDE that describes its evolution over time. The algorithm uses the likelihood ratio of the learned stochastic processes in different timestamps to find change points of the process. We demonstrate the detection capabilities and performance of our algorithm on synthetic and real-world datasets. The proposed method outperforms the state-of-the-art algorithms on the majority of our experiments.
更多查看译文
关键词
Time series analysis,Signal processing algorithms,Deep learning,Approximation algorithms,Task analysis,Monitoring,Machine learning algorithms,Anomaly detection,Machine learning,change point detection,deep learning,machine learning,timeseries
AI 理解论文
溯源树
样例
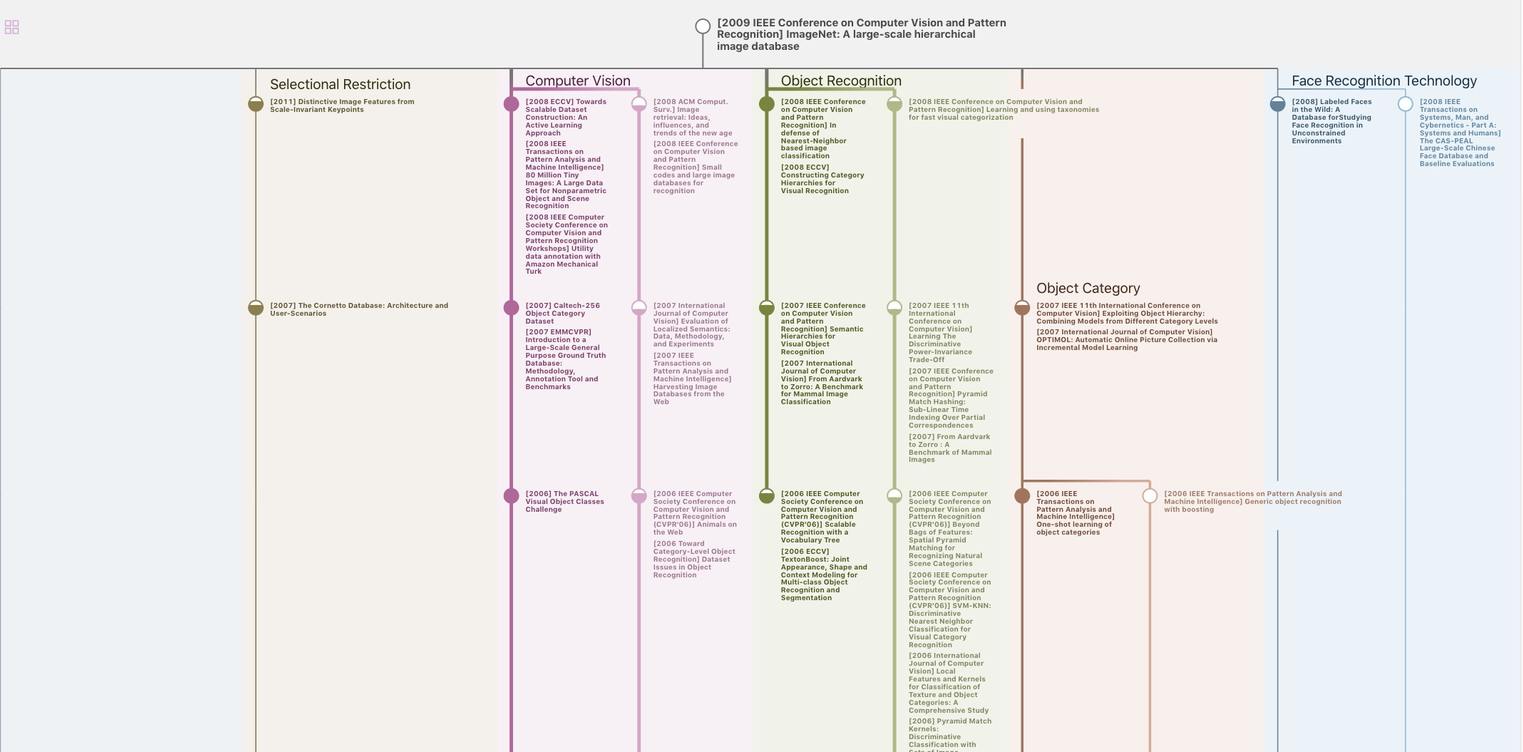
生成溯源树,研究论文发展脉络
Chat Paper
正在生成论文摘要