Image Reconstruction in Electrical Capacitance Tomography Using ROI-Shrinkage Adaptive Block Sparse Bayesian Learning.
IEEE Trans. Instrum. Meas.(2023)
摘要
In this article, a region of interest (ROI)-shrinkage adaptive block sparse Bayesian learning (R-ABSBL) method is proposed for image reconstruction in electrical capacitance tomography (ECT). The conventional block sparse Bayesian learning (BSBL) method adapts the fixed block structure to encode the image but ignoring its changes with iteration and expends a huge amount of computational cost to do the large-scale matrix inversion required in image reconstruction. To overcome these shortcomings, the proposed method utilizes adaptive block structure to encode the image per iteration, adaptively exploiting both sparsity prior and intrablock correlation in reconstructed image. Besides, it realizes the shrinking ROI-based reconstruction by adaptively selecting the blocks to be updated in the next iteration and simplifies the large-scale matrix inversion by applying a dedicated formula transform, significantly reducing the dimension of unknowns and alleviating the computational complexity. The proposed method incorporates adaptive block encoding, ROI-shrinkage, and improved inversion strategies in image reconstruction, efficiently balancing both spatial resolution and imaging speed. Numerical simulation and phantom experiment were carried out to evaluate the performance of the proposed method. It is shown that the proposed method can generate more accurate images and is much less computationally intensive than the conventional BSBL method. It has great potential in reconstructing sparse abnormal changes of dielectric distribution in multiphase flow measurements requiring both imaging resolution and speed.
更多查看译文
关键词
electrical capacitance tomography,image reconstruction,roi-shrinkage
AI 理解论文
溯源树
样例
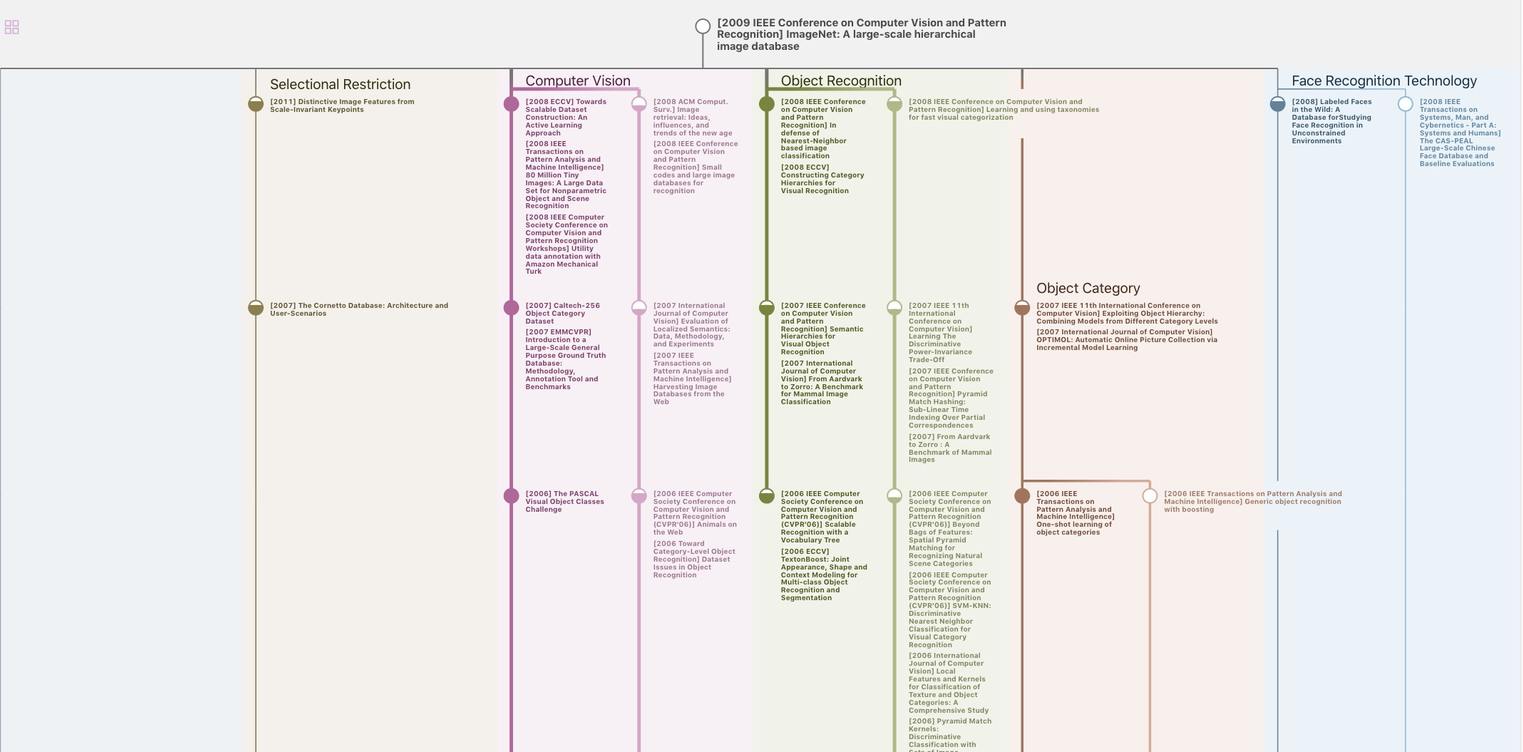
生成溯源树,研究论文发展脉络
Chat Paper
正在生成论文摘要