Treat Noise as Domain Shift: Noise Feature Disentanglement for Underwater Perception and Maritime Surveys in Side-Scan Sonar Images.
IEEE Trans. Geosci. Remote. Sens.(2023)
摘要
In underwater perception and maritime surveys, due to the scarcity of training data and perturbation of speckle noise, the detection performance of underwater objects in side-scan sonar (SSS) images is limited. To address these problems, we proposed a noise feature disentanglement you only look once (YOLO) (NFD-YOLO) by combining noise-agnostic features learning and attention mechanism. First, we rethink the speckle noise by treating it as the domain shift between the training dataset and real-measured SSS images and build a domain generalization-based (DG-based) underwater object detection framework. Then, we extend YOLOv5 with a feature manipulation module, a noise-agnostic subnetwork, and an auxiliary noise-biased subnetwork for noise features disentanglement, more biases toward noise-agnostic features and less reliance on noise-biased features in underwater object detection, respectively. Finally, the ACmix attention module is introduced for a more powerful learning capacity and attention to the object areas based on a small dataset. According to the experiment results, the proposed NFD-YOLO achieved 75.1% mean average precision (mAP) in the test domain, which increased by 7.5% than YOLOv5, and 75.7% ± 0.4% mAP and 77.5% ± 1.6% mAP for different speckle noise distributions and transfer directions, respectively, which verified its generalization ability and robustness for speckle noise; therefore, the proposed method can mitigate the effects of speckle noise and provides a new thought to address the speckle noise in underwater object detection with a small dataset, which is of significance and benefits for underwater perception and maritime surveys.
更多查看译文
关键词
underwater perception,maritime surveys,noise,disentanglement,side-scan
AI 理解论文
溯源树
样例
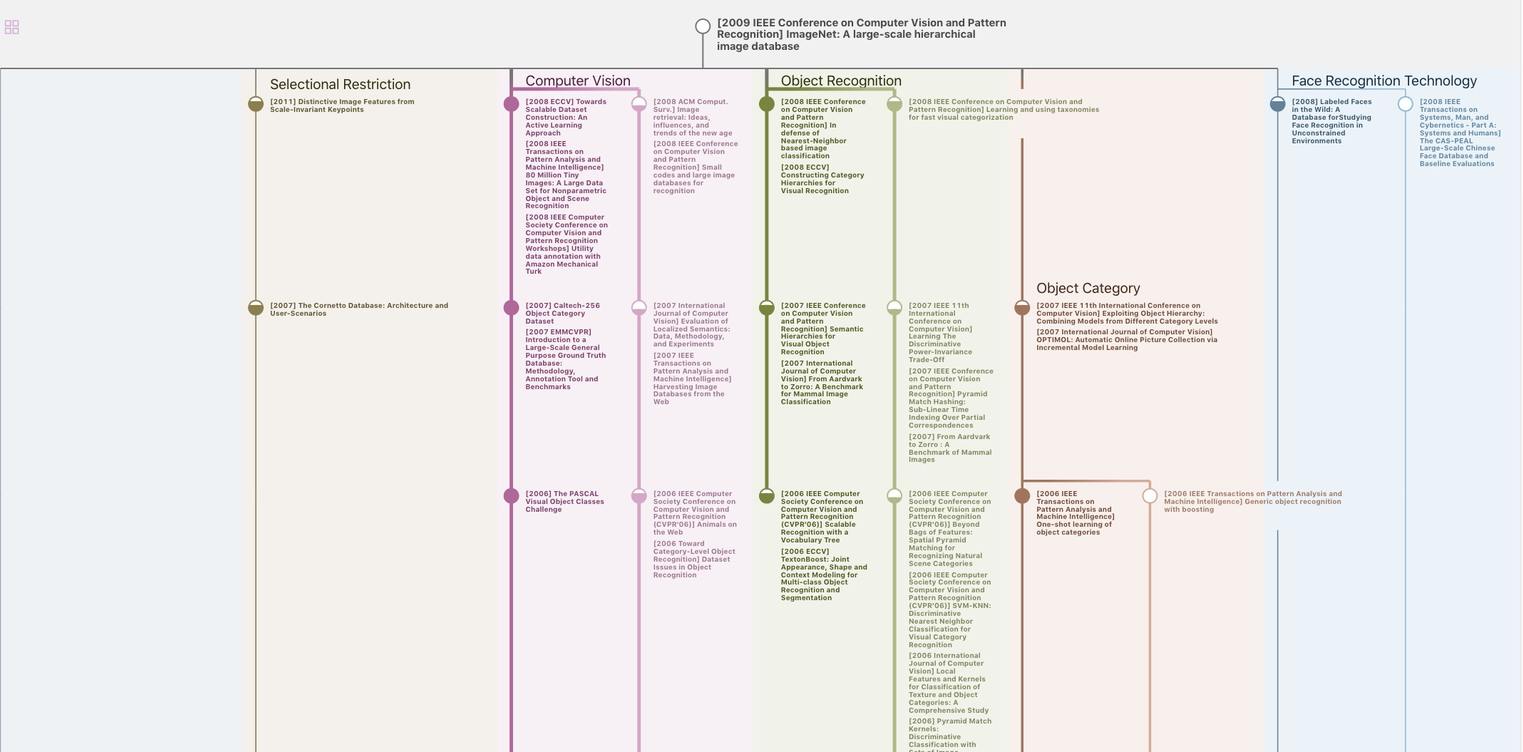
生成溯源树,研究论文发展脉络
Chat Paper
正在生成论文摘要