CAL-Net: Conditional Attention Lightweight Network for In-Orbit Landslide Detection
IEEE Trans. Geosci. Remote. Sens.(2023)
摘要
Landslides are common and highly threatening geological disasters, and detecting landslide occurrence areas using remote sensing images has important social significance for disaster warning, prediction, and proactive rescue. Currently, the most effective and direct application is in-orbit real-time detection, which obtains the boundary, location and other relevant information of the post-disaster areas by performing in-orbit calculations on the raw images, thereby shortening the relief cycle. However, challenges associated with this application are high efficiency and portability, while overcoming complex surface distributions to ensure high accuracy. In this article, we propose a lightweight network framework and deploy it on a civilian QK series environmental monitoring satellite, transmitting the detection results in graphic form to the ground station. This framework addresses the problems of parameter redundancy and low real-time performance in existing embedded device landslide detection methods. The model adopts a conditional attention mechanism, multifeature fusion, and asymmetric upsampling modules (AUMs). It employs only 3.045 MB of parameters, with a mean intersection over union (MIoU) of 88.38% and an F1-scores of 93.77%, striking a good balance between computational efficiency and accuracy, and its performance can meet the requirements of inorbit applications. Additionally, we have labeled and published landslide samples that occurred in Luding, Sichuan Province, Southwest China, in September 2022, which is available at https://github.com/fuadou/project/tree/main/Luding_datasets.
更多查看译文
关键词
Terrain factors,Feature extraction,Image segmentation,Remote sensing,Satellites,Decoding,Real-time systems,Conditional convolution,landslide detection,lightweight network,satellite application
AI 理解论文
溯源树
样例
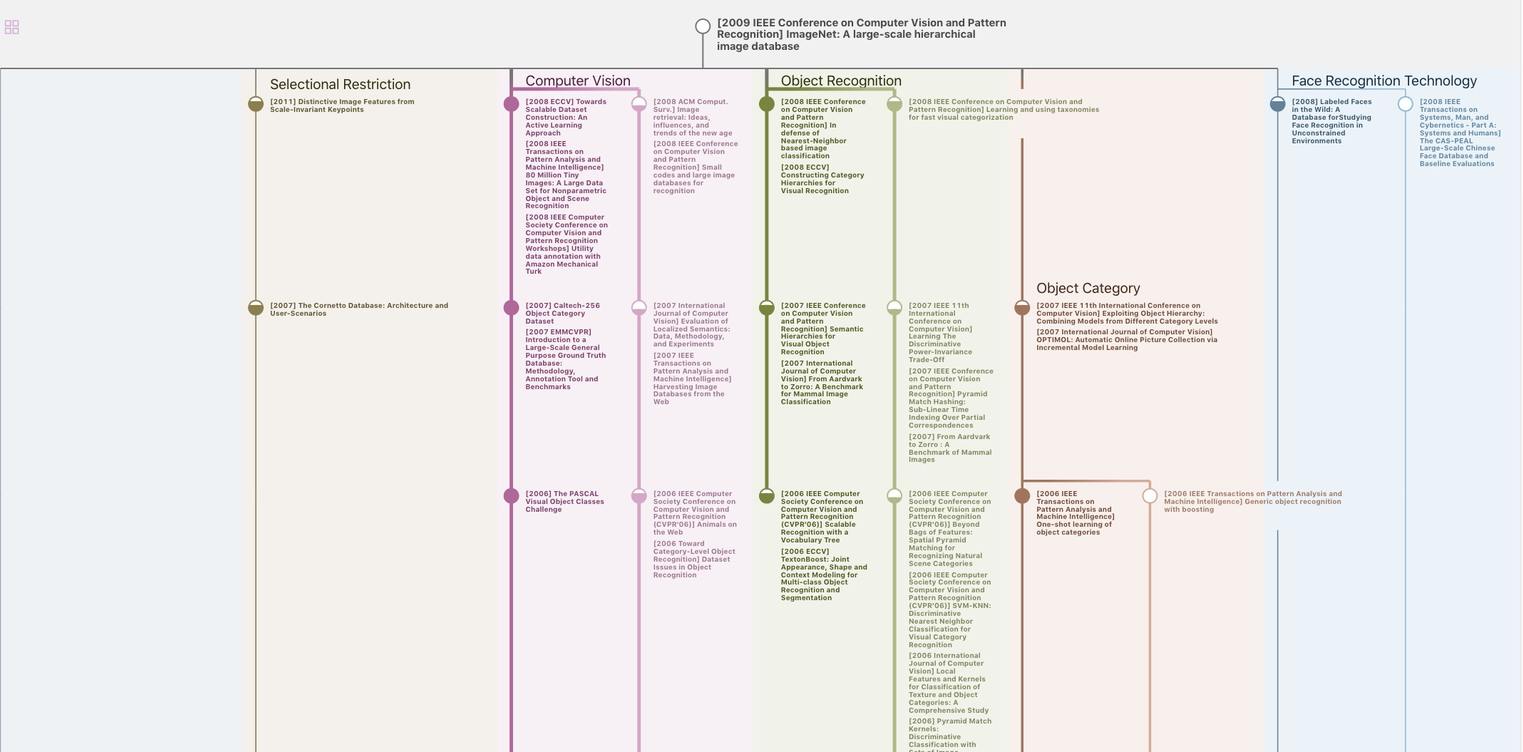
生成溯源树,研究论文发展脉络
Chat Paper
正在生成论文摘要