A Two-Stage Hierarchical One-Class Classification Structure for HFSWR Ship-Target Detection.
IEEE Trans. Geosci. Remote. Sens.(2023)
摘要
A high-frequency surface wave radar (HFSWR) is an effective tool for monitoring an exclusive economic zone (EEZ). However, the presence of diverse clutters and noises that contaminate the echo signals of the radar hinders its maritime surveillance. To address this issue, this article presents a two-stage hierarchical one-class classification network (HOCN) designed specifically for ship-target detection in range–Doppler (RD) images. In Stage 1, the plausible region of interest (PROI) is extracted. This stage uses a dynamic threshold optimization strategy and Laplacian kernel to identify the potential regions of interest. In Stage 2, the proposed one-class deconvolutional-and-convolutional network (OC-DCNet) is used for fine detection of ship targets. This stage comprises two submodules: the deconvolutional submodule, which expands the input into a 2-D matrix, and the convolutional submodule, which classifies the input pattern as either a ship target or a nonship target. The experimental results on a newly collected dataset called HFRD demonstrate the effectiveness of the proposed HFSWR ship-target detection algorithm.
更多查看译文
关键词
High-frequency surface wave radar (HFSWR),one-class classification (OCC),representation learning,ship-target detection
AI 理解论文
溯源树
样例
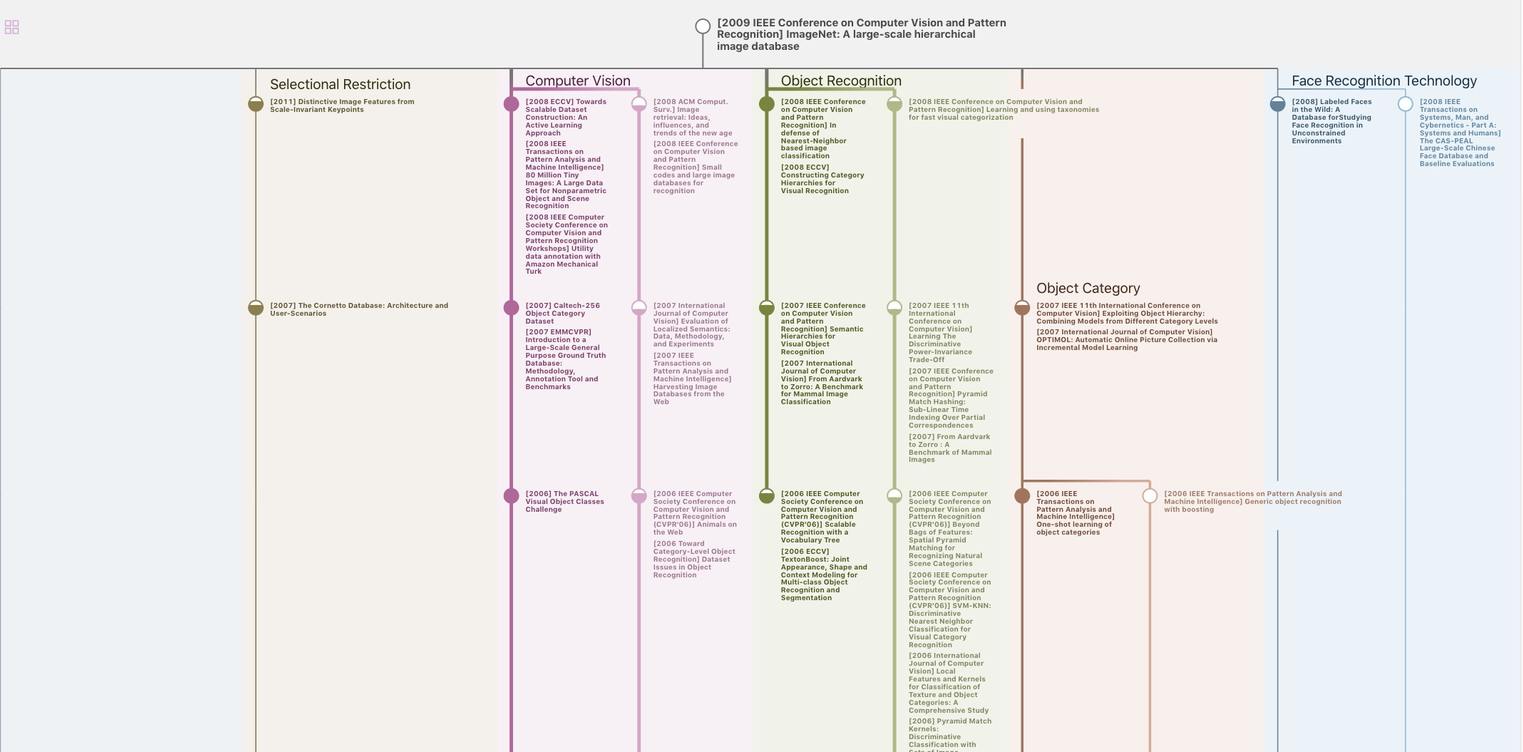
生成溯源树,研究论文发展脉络
Chat Paper
正在生成论文摘要