Transfer-Learning-Based Approach to Retrieve the Cloud Properties Using Diverse Remote Sensing Datasets.
IEEE Trans. Geosci. Remote. Sens.(2023)
摘要
Clouds play an important role in the Earth’s climate system; however, various observational methods describe clouds differently, leading to cloud products being described with different characteristics, and affecting our understanding of cloud effects. To address this problem, this study integrates different cloud products into the transfer-learning procedure of a deep-learning model and determines the cloud effective radius (CER), cloud optical thickness (COT), and cloud top height (CTH) from Himawari-8 thermal infrared measurements. The retrieval results were independently evaluated against the moderate-resolution imaging spectroradiometer science products and further compared with Himawari-8 operational products during the day. The root mean squared errors (RMSEs) of the model for the CER, COT, and CTH were
$4.490~\mu \text{m}$
, 11.198, and 1.904 km, respectively, which are lower than those of Himawari-8 operational products (RMSE:
$11.172~\mu \text{m}$
, 14.755, and 2.860 km). Moreover, validation results against active sensors show that the model performs slightly better during the day than at night, and both are generally better than the Himawari-8 operational product. Overall, the model maintains stable performance during both day and night, and its accuracy is higher than that of Himawari-8 operational products.
更多查看译文
关键词
Cloud properties,Himawari-8,Small Attention-UNet (SmaAt-UNet),transfer-learning
AI 理解论文
溯源树
样例
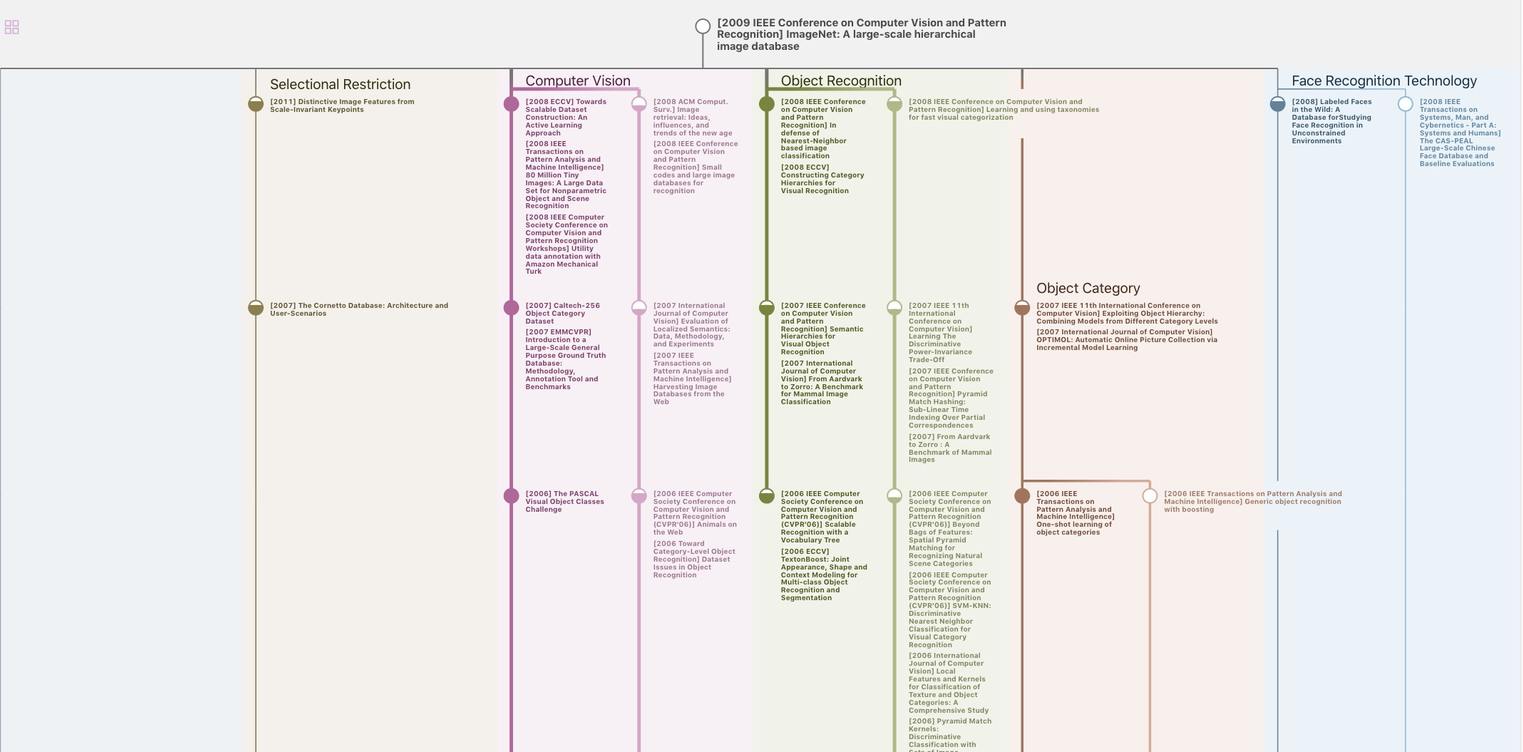
生成溯源树,研究论文发展脉络
Chat Paper
正在生成论文摘要