AACNet: Asymmetric Attention Convolution Network for Hyperspectral Image Dehazing.
IEEE Trans. Geosci. Remote. Sens.(2023)
摘要
Haze in hyperspectral images (HSIs) can lead to crosstalk between multiple bands, resulting in errors that can be amplified and transmitted during data processing. As a consequence, this may cause a reduction in the accuracy and precision of remote sensing data. The purpose of haze removal is to restore high-quality HSIs from degraded ones. The high spectral resolution and typically dozens to hundreds of spectral bands in HSIs pose significant challenges for haze removal. Thus, many methods designed for natural and multispectral images are not effective in removing haze in HSIs. To address this challenge, we develop a model called asymmetric attention convolution network (AACNet) designed for haze removal in HSIs. Specifically, the basic architecture of AACNet is mainly composed of several residual asymmetric attention groups (RAAGs), where the core components are residual asymmetric attention blocks (RAABs). This design enables the full utilization of deep spatial–spectral features while skipping low-frequency regions and focusing more on the haze-affected areas. To more accurately restore the spectral information in areas polluted by haze, a pooling channel self-attention (PCSA) module has been proposed. This module can effectively reconstruct the spectral response curve that is affected by the haze. Our experiments on both simulated and real datasets demonstrate that the proposed AACNet outperforms several leading haze removal methods in both precision and visual quality. The source code and data of this article will be made publicly available at
https://github.com/SZU710/AACNet
for reproducible research.
更多查看译文
关键词
asymmetric attention convolution network,aacnet
AI 理解论文
溯源树
样例
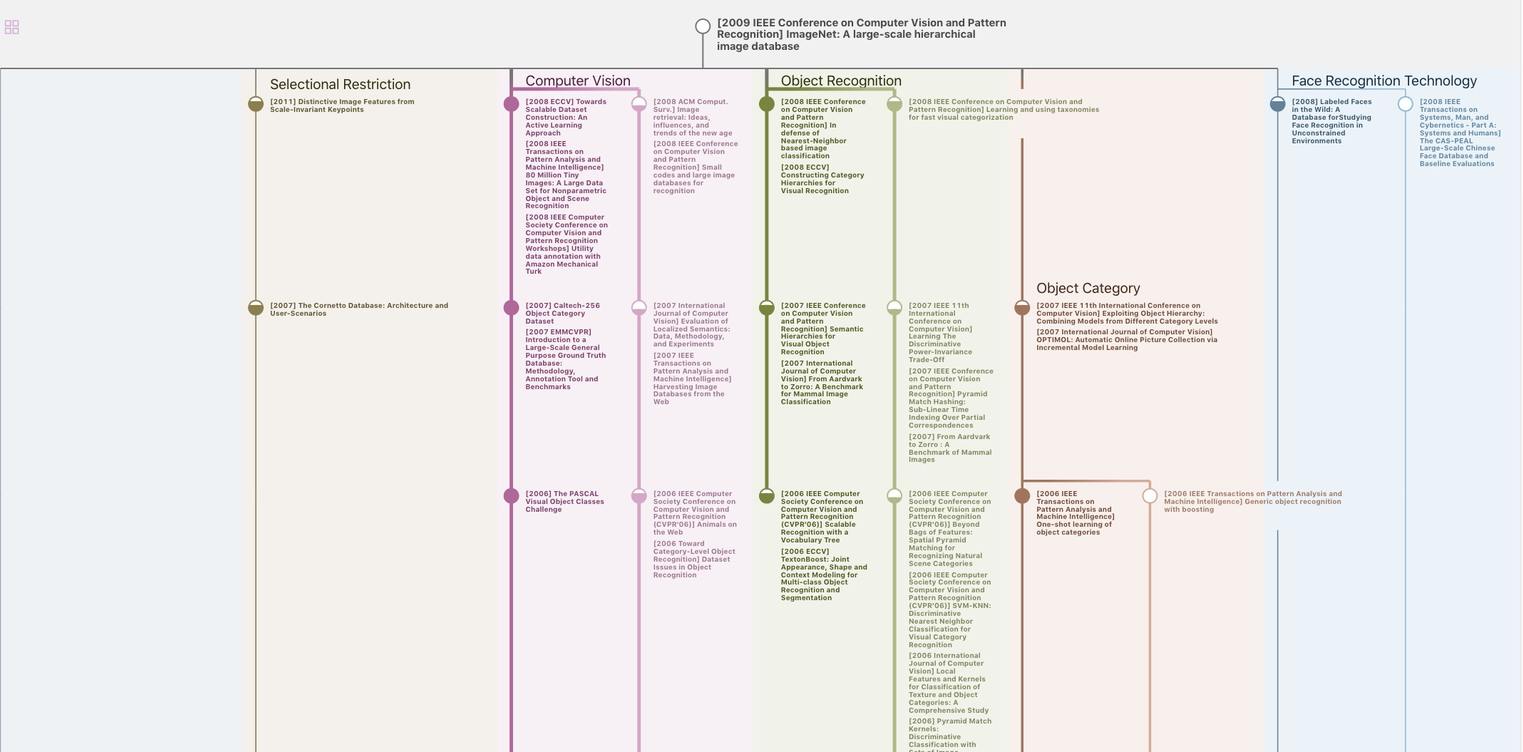
生成溯源树,研究论文发展脉络
Chat Paper
正在生成论文摘要