Adversarial Domain Alignment With Contrastive Learning for Hyperspectral Image Classification.
IEEE Trans. Geosci. Remote. Sens.(2023)
摘要
Recently, deep learning-based hyperspectral image (HSI) classification techniques are flourishing and exhibit good performance, where cross-domain information is usually utilized to reduce the dependence on large labeled samples. However, the gap between the source domain and the target domain makes it difficult to carry out knowledge transfer directly. In this article, an adversarial domain alignment with a contrastive learning method is designed for the HSI classification task to achieve feature consistency that benefits transferring knowledge. In detail, spectral alignment and semantic alignment are conducted at local and global levels, respectively, in an adversarial learning way, and the adversarial loss acts on both source and target domains. In order to learn specific features for objects with different spatial scales, a multiscale selection module is constructed in semantic alignment to select channel features adaptively. Moreover, contrastive learning is employed to increase both robustness and sensitiveness, where augmented data from the same/different samples are forced to be similar/dissimilar with each other. The training process is conducted in a few-shot learning way; then, the few-shot classification loss, the adversarial loss, and the contrastive loss are optimized together. Tested on one source dataset and four target datasets, the experimental results show that the proposed method outperforms the other comparisons.
更多查看译文
关键词
Adversarial learning, classification, contrastive learning, domain alignment, hyperspectral image (HSI)
AI 理解论文
溯源树
样例
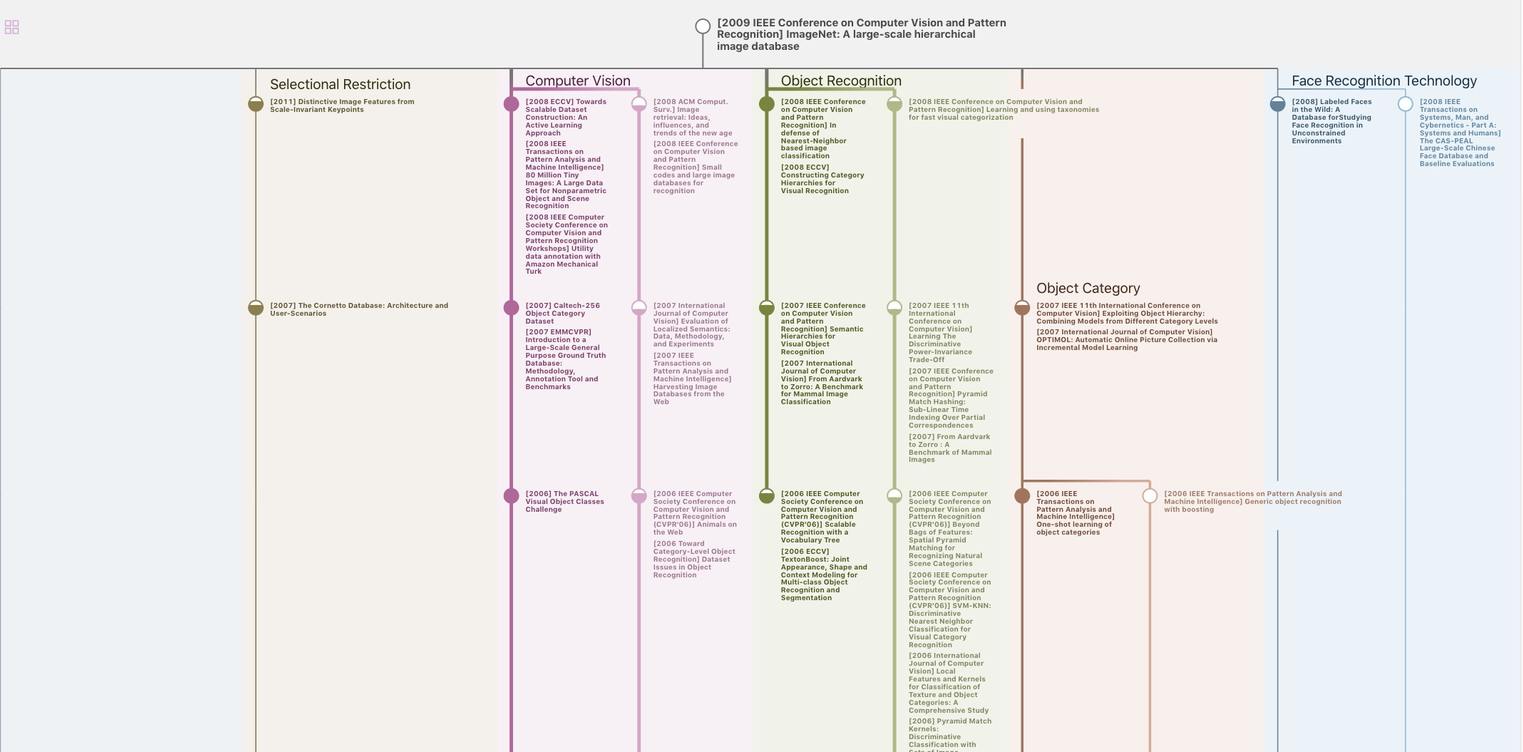
生成溯源树,研究论文发展脉络
Chat Paper
正在生成论文摘要