BrewER: Entity Resolution On-Demand.
Proc. VLDB Endow.(2023)
摘要
The task of entity resolution (ER) aims to detect multiple records describing the same real-world entity in datasets and to consolidate them into a single consistent record. ER plays a fundamental role in guaranteeing good data quality, e.g., as input for data science pipelines. Yet, the traditional approach to ER requires cleaning the entire data before being able to run consistent queries on it; hence, users struggle to tackle common scenarios with limited time or resources (e.g., when the data changes frequently or the user is only interested in a portion of the dataset for the task). We previously introduced BrewER, a framework to evaluate SQL SP queries on dirty data while progressively returning results as if they were issued on cleaned data, according to a priority defined by the user. In this demonstration, we show how BrewER can be exploited to ease the burden of ER, allowing data scientists to save a significant amount of resources for their tasks.
更多查看译文
关键词
brewer,on-demand
AI 理解论文
溯源树
样例
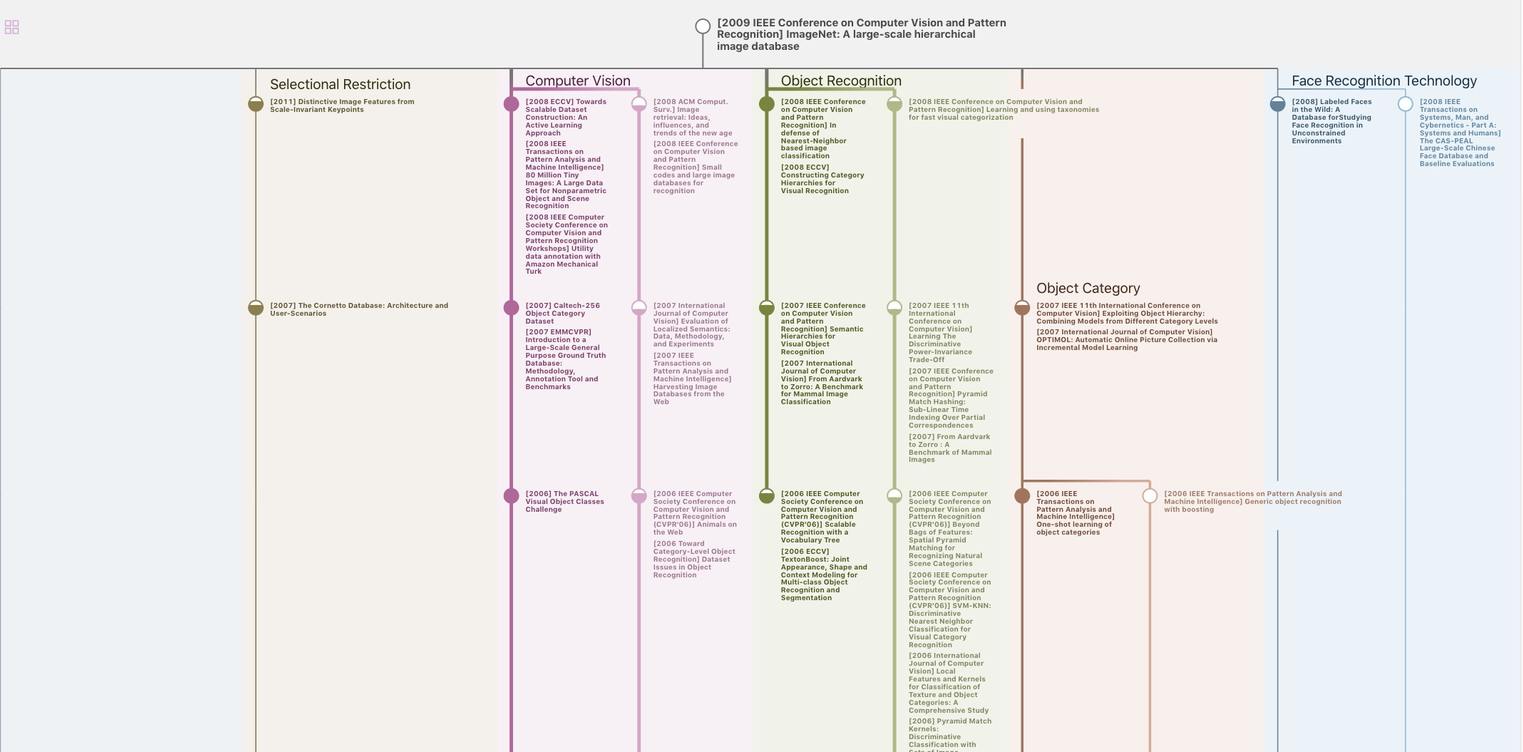
生成溯源树,研究论文发展脉络
Chat Paper
正在生成论文摘要