A dual-task region-boundary aware neural network for accurate pulmonary nodule segmentation
J. Vis. Commun. Image Represent.(2023)
摘要
Recently, the performance of pulmonary nodule segmentation has improved fast because of the development of deep learning-based methods. However, existing CNN-based methods still don’t solve the two issues, leading to poor segmentation: (1) The information of small nodules lost in continuous down-sampling operation; (2) Discriminative features can’t be extracted from non-solid nodules because whose gray values are close to the surrounding environment. This study proposes a novel dual-task region-boundary aware deep convolutional neural network to solve the problems above. A hierarchical feature module is proposed to capture multi-scale information, improving small nodules' segmentation accuracy. Boundary guided module is introduced to extract edge features and predict nodule boundaries directly. In addition, a feature aggregation module is proposed to aggregate features at different levels. Besides, to better optimize the segment results and reduce overfitting, the region-boundary aware loss function is proposed. The method is trained and tested on the LIDC-IDRI dataset and the LUNA16 dataset, and the results demonstrate that our method is efficient. More importantly, our method is suitable for the segmentation of various nodules, especially small nodules, GGO nodules, and part-solid nodules.
更多查看译文
关键词
Pulmonary nodules,Segmentation,Region-boundary joint learning,Multi-task learning
AI 理解论文
溯源树
样例
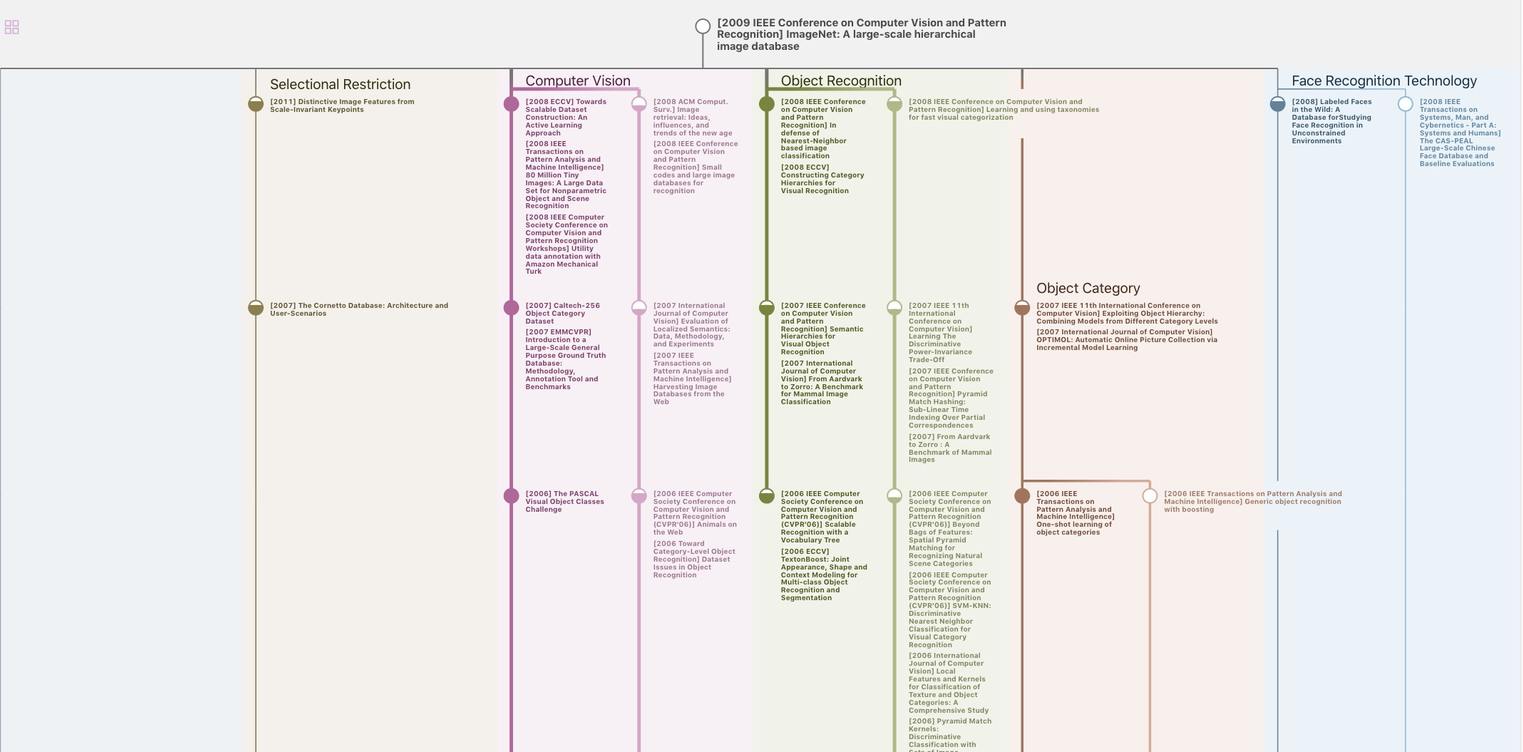
生成溯源树,研究论文发展脉络
Chat Paper
正在生成论文摘要