Lightweight Multi-Objective and Many-Objective Problem Formulations for Evolutionary Neural Architecture Search with the Training-Free Performance Metric Synaptic Flow.
Informatica (Slovenia)(2023)
摘要
Neural architecture search (NAS) with naive problem formulations and applications of conventional search algorithms often incur prohibitive search costs due to the evaluations of many candidate architectures. For each architecture, its accuracy performance can be properly evaluated after hundreds (or thousands) of computationally expensive training epochs are performed to achieve proper network weights. A so-called zero-cost metric, Synaptic Flow, computed based on random network weight values at initialization, is found to exhibit certain correlations with the neural network test accuracy and can thus be used as an efficient proxy performance metric during the search. Besides, NAS in practice often involves not only optimizing for network accuracy performance but also optimizing for network complexity, such as model size, number of floating point operations, or latency, as well. In this article, we study various NAS problem formulations in which multiple aspects of deep neural networks are treated as multiple optimization objectives. We employ a widely-used multi-objective evolutionary algorithm, i.e., the non-dominated sorting genetic algorithm II (NSGA-II), to approximate the optimal Pareto-optimal fronts for these NAS problem formulations. Experimental results on the NAS benchmark NATS-Bench show the advantages and disadvantages of each formulation.
更多查看译文
关键词
evolutionary neural architecture search,flow,multi-objective,many-objective,training-free
AI 理解论文
溯源树
样例
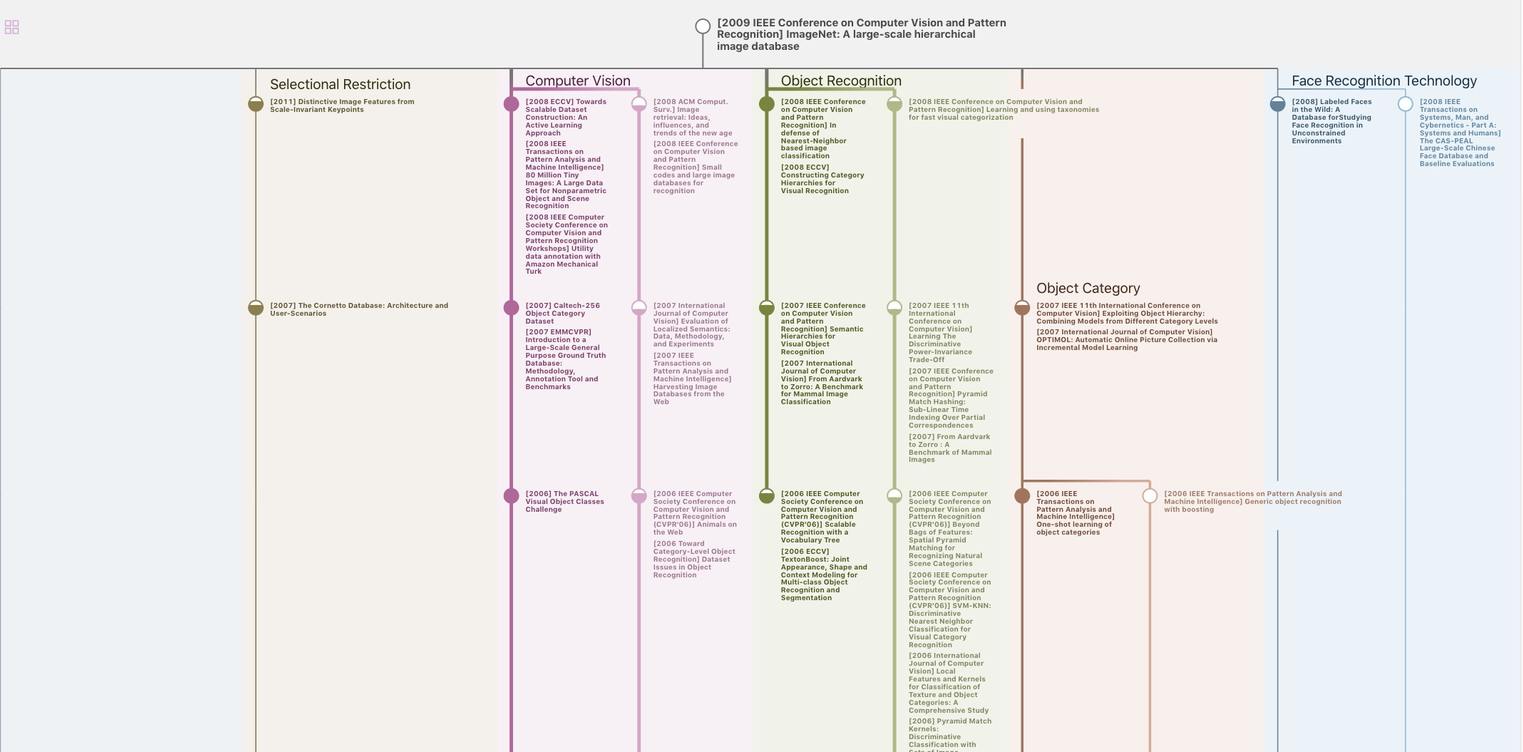
生成溯源树,研究论文发展脉络
Chat Paper
正在生成论文摘要