Multikernel adaptive filtering over graphs based on normalized LMS algorithm
SIGNAL PROCESSING(2024)
摘要
To address the difficulty and inflexibility associated with choosing kernel parameters for single kernel adaptive filters (KAFs), this article proposes a multikernel adaptive filter for graph signals based on the least mean square (LMS) algorithm. First, normalized by its largest eigenvalue, the combinatorial graph Laplacian is adopted as the graph shift operator (GSO) to preprocess graph input signals. Then, the graph multikernel normalized least mean square (GMKNLMS) algorithm is developed to estimate nonlinear graph filter coefficients. To limit the growth in dictionary size, a coherence-check (CC) based sparsification method is introduced to form the new GMKNLMS-CC algorithm. In addition, numerical simulation examples are presented to demonstrate the improved performance of the proposed algorithms compared with the linear graph least mean square (GLMS) and graph kernel normalized least mean square (GKNLMS) algorithms. Finally, the real sensor measurement data taken from the Intel Lab is used as time-varying graph signals to demonstrate the tracking performance of the GMKNLMS-CC algorithm.
更多查看译文
关键词
Multikernel adaptive filtering,Graph signals,Least mean square algorithm,Coherence-check
AI 理解论文
溯源树
样例
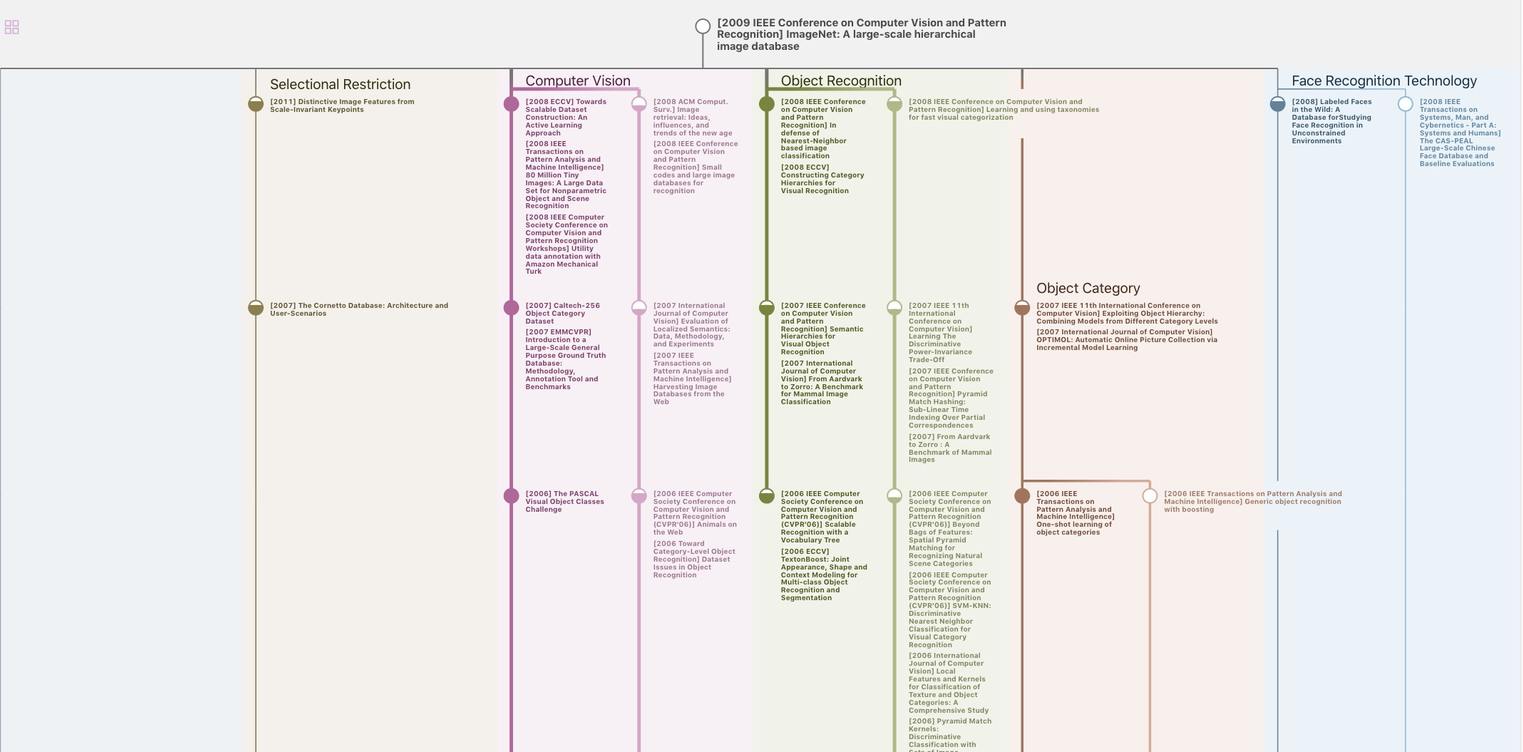
生成溯源树,研究论文发展脉络
Chat Paper
正在生成论文摘要